Multi-Reference-Based Cross-Scale Feature Fusion for Compressed Video Super Resolution
IEEE Transactions on Broadcasting(2024)
摘要
To save transmission bandwidth, there exists an approach to down-sample a video and then up-sample the compressed video to save bit rates. The existing Super Resolution (SR) methods generally design powerful networks to compensate the loss information introduced by down-sampling. But the information of entire video is not fully utilized and effectively fused, resulting in the learned context information that is not enough for the high quality reconstruction. We propose a multi high-quality frames Referenced Cross-scale compressed Video Super Resolution method (RCVSR) that wisely uses past-and-future information, to pursue higher compression efficiency. Specifically, a joint reference motion alignment module is proposed. Low resolution (LR) frame after up-sampling is separately aligned with past-and-future reference frames to preserve more spatial details; at the same time this LR frame is aligned with neighborhood frames to get continuous motion information and similar contents. Then, a reference based refinement module is applied to compensate motion and lost texture details by computing similarity matrix across channel dimensions. Finally, an attention guided dual-branch residual module is employed to enhance the reconstructed result concurrently. Compared with the HEVC anchor, the average gain of Bjontegaard Delta Rate (BD-Rate) under the Low-Delay-P (LDP) setting is 24.86%. In addition, an experimental comparison is made with the advanced SR methods and compressed video quality enhancement (VQE) methods, and the superior efficiency and generalization of the proposed algorithm are further reported.
更多查看译文
关键词
Video compression,video quality enhancement,video super resolution,deep learning
AI 理解论文
溯源树
样例
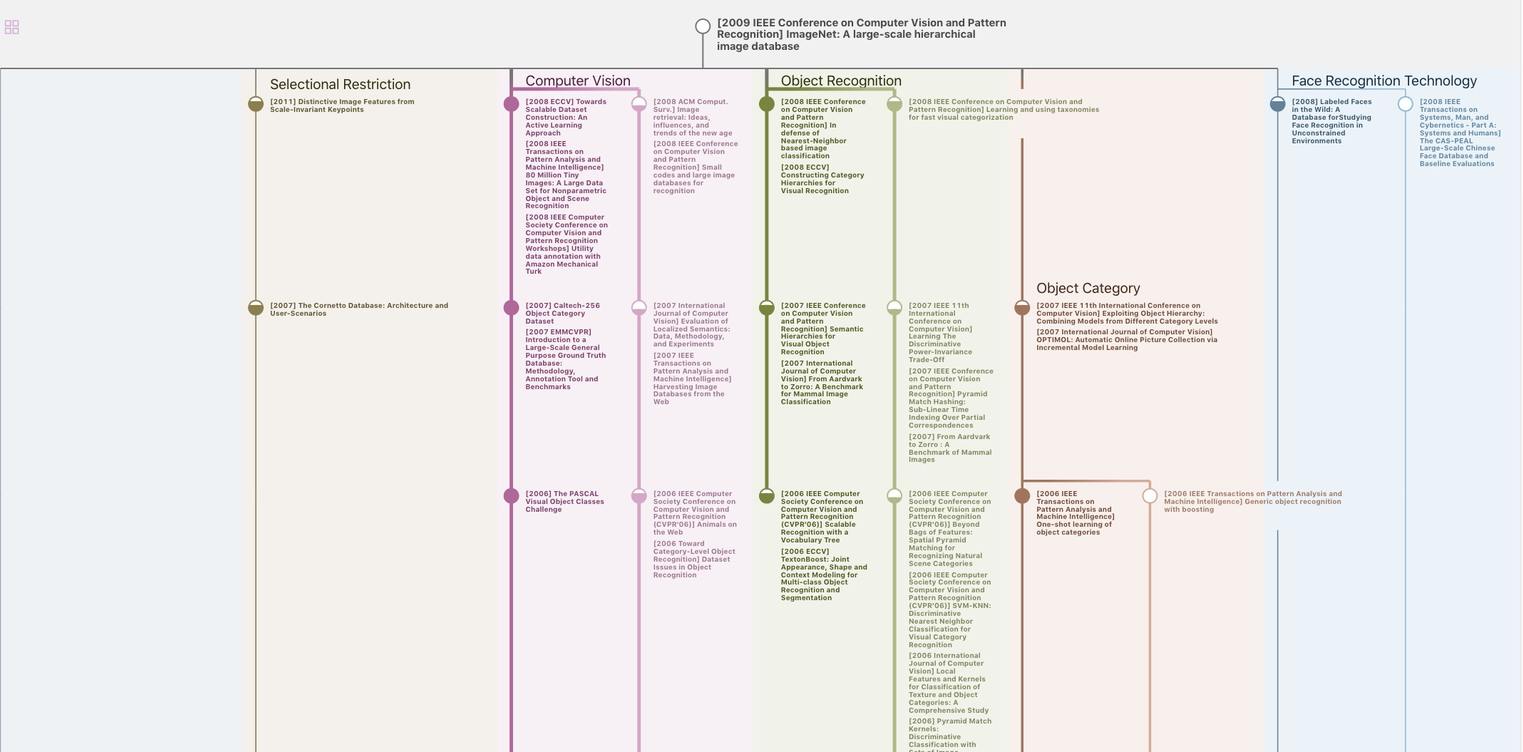
生成溯源树,研究论文发展脉络
Chat Paper
正在生成论文摘要