Machine learning-based prediction approach for ranging resolution enhancement of FMCW LiDAR system with LSTM networks
OPTICS AND LASER TECHNOLOGY(2024)
摘要
Ranging resolution is one of the most important factors of frequency-modulated continuous wave (FMCW) light detection and ranging (LiDAR) systems, which is determined by the effective sweep bandwidth and observation time of the ranging signal used for Fourier transform. Conventional methods for resolution enhancement either require expensive devices or complicated operation with human intervention, which is high-cost and inefficient. For the first time, a machine learning-based prediction approach is proposed for resolution improvement. A long short-term memory (LSTM) network with a special architecture, which is driven by a theoretical model of FMCW ranging signals, is established for signal prediction. A resampling-based preprocessing block is then designed to denoise the original ranging data and enhance its feature. Finally, multi-step prediction of the input time-series signal is performed using the well-trained LSTM network to increase effective data length so as to enhance the resolution. The effectiveness of the proposed method is verified through several experiments. The results show that the proposed method not only has the advantages of low-cost and high-efficiency, but also enables the FMCW LiDAR system to possess larger equivalent bandwidth, better ranging resolution, and higher signal-tonoise ratio (SNR), enlightening the development of machine learning in high-precision ranging systems.
更多查看译文
关键词
Long short-term memory (LSTM),Light detection and ranging (LiDAR) system,Machine learning,Resolution enhancement
AI 理解论文
溯源树
样例
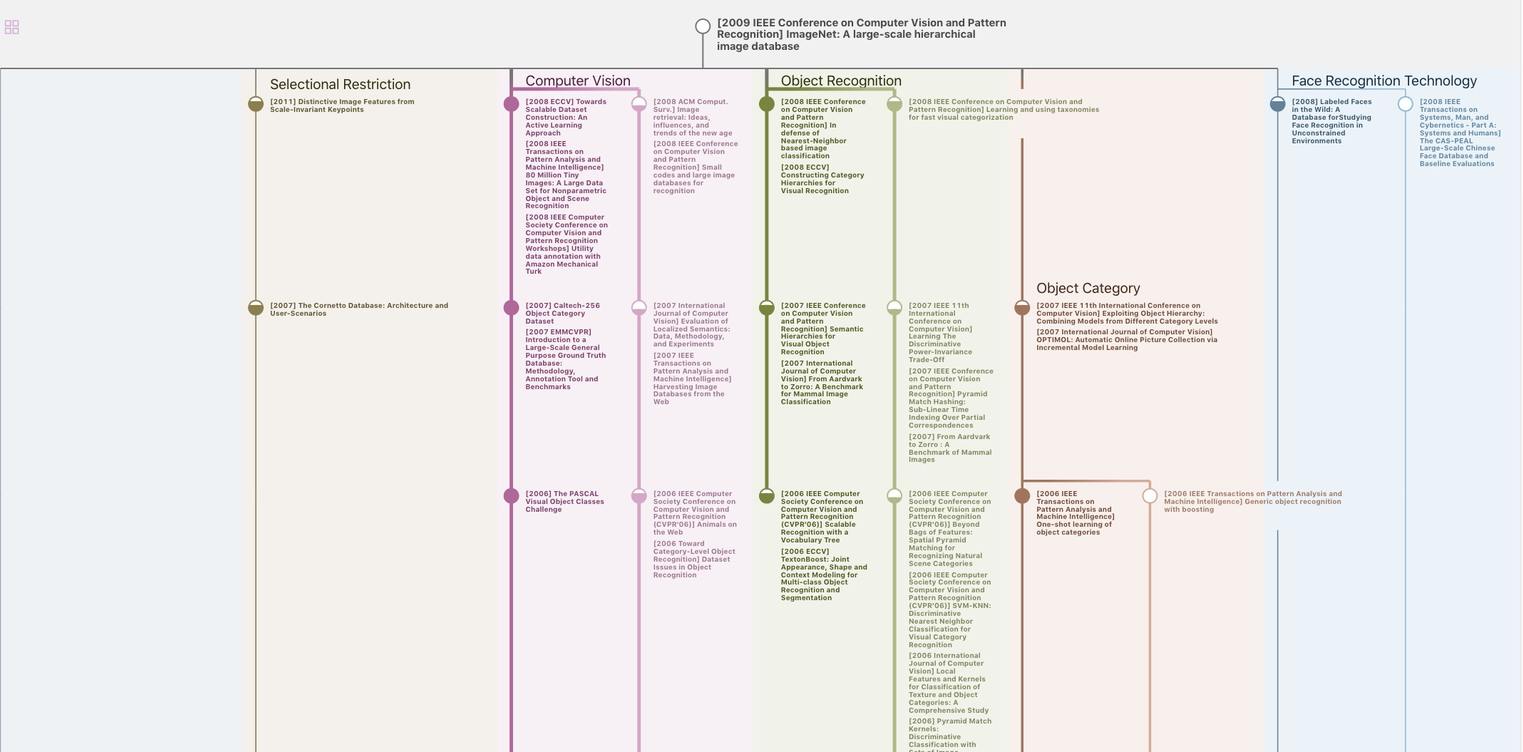
生成溯源树,研究论文发展脉络
Chat Paper
正在生成论文摘要