Select-Sliced Wasserstein Distance for Point Cloud Learning.
International Conference on 3D Vision(2024)
摘要
Quantifying the discrepancy between point sets is a critical component for point cloud learning tasks. The mainstream point cloud learning tasks utilize Chamfer distance and Earth-Mover’s distance. Chamfer distance is computationally efficient but may not fully capture differences between sets of points. Earth-Mover’s distance, while precise, is computationally expensive and can be impractical to use with high-definition data. Several variants of Sliced Wasserstein distances (SW) are introduced to reduce the computation cost, but bring new problems to the situation: The vanilla SW treats sampled slices equally, resulting in redundant projections; Distributional Sliced Wasserstein distance requires gradient-based optimization, offsetting its benefits. To overcome this limitation and leverage the advantages of Sliced Wasserstein distance over EMD, we propose a novel metric, Select-Sliced Wasserstein distance. This new distance analyzes drawn samples of slices and quantifies their informativeness for each point in a single shot, which eliminates unnecessary projections as well as costly optimizations, but perpetuates the performance. Extensive experiments on various point cloud learning tasks to demonstrate the efficiency and effectiveness of the proposed distance metric. Our code is available at https://github.com/VideoProcessingLab/SSW_Distance
更多查看译文
关键词
Point Cloud,Point Cloud Learning,Computational Efficiency,Single Shot,Earth Mover’s Distance,Chamfer Distance,Training Set,Deep Learning,Learning Rate,Adam Optimizer,Semantic Segmentation,Feasible Set,Discrete Distribution,Discrete Space,Unit Sphere,Optimal Transport,Projection Angle,Point Cloud Registration,Earth Mover,Point Cloud Generation,Point Cloud Reconstruction,Radon Transform
AI 理解论文
溯源树
样例
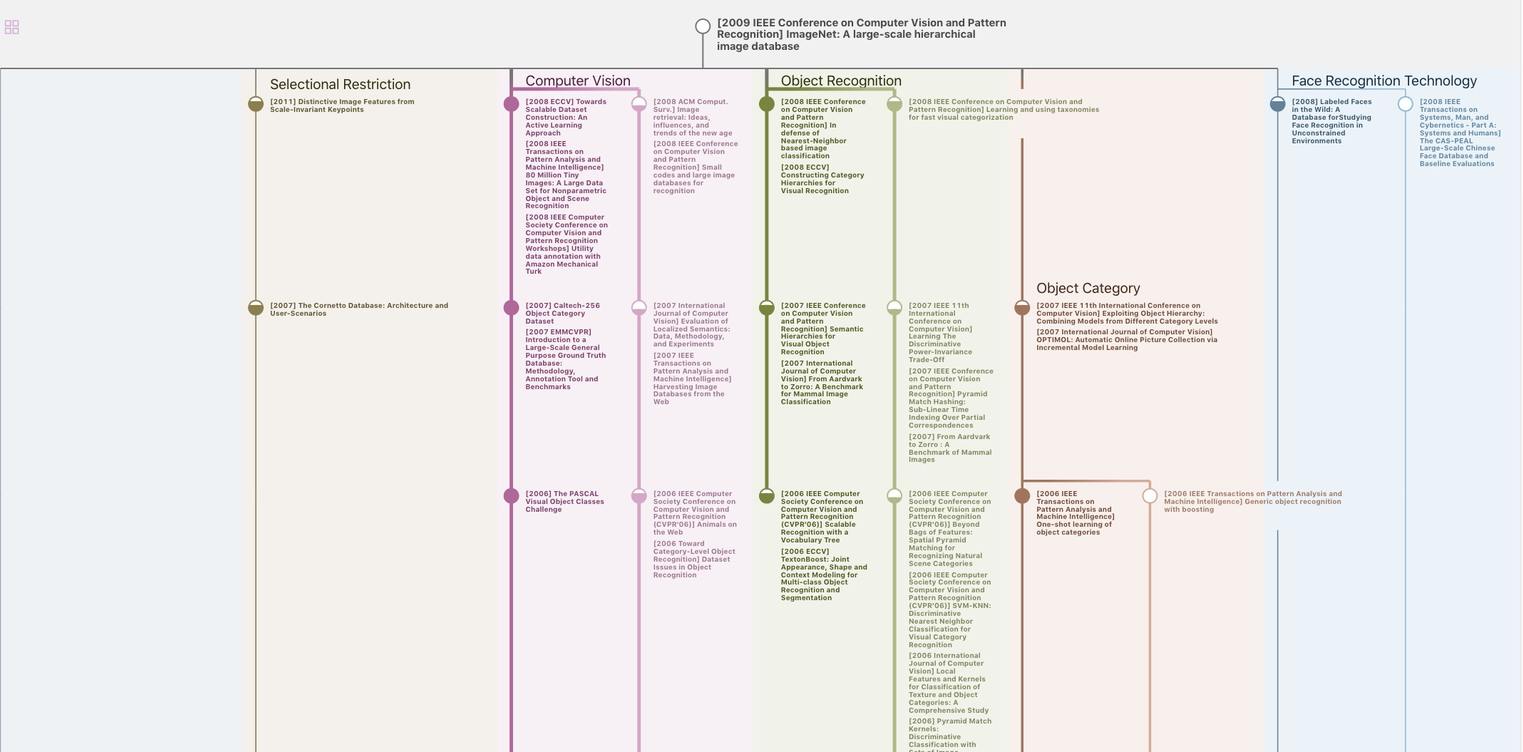
生成溯源树,研究论文发展脉络
Chat Paper
正在生成论文摘要