Improved Hierarchical Bayesian Optimization Algorithm with LSTM for Crude Oil Price Forecasting
2024 Third International Conference on Distributed Computing and Electrical Circuits and Electronics (ICDCECE)(2024)
Abstract
Accurate oil price forecasting enables economists, entrepreneurs, and policymakers to foresee market trends and make well-informed decisions. The issue statement of current approaches is unable to handle stopping parameter changes, overfitting, underfitting, and the model's learning rate. More data that has been tested and trained upon is present in the West Texas Intermediate (WTI) dataset utilized in the suggested methodology. The suggested technique uses the Long Short-Term Memory Algorithm (LSTM) to forecast the price of crude oil in the future and the Improved Hierarchical Bayesian Optimization Algorithm for hyperparameter tuning. Initially, the raw data is normalized using Variational Mode Decomposition (VMD), representative nonlinear feature information is extracted using Phase Space Reconstruction (PSR), and the chaotic character of the data is confirmed using the maximal Lyapunov exponent. This approach's numerical validation yielded a Coefficient of Determination (R
2
) of 1.79566, a Mean Square Error (MSE) of 0.00010, a Mean Absolute Error (MAE) of 0.00720, and a Root Mean Square Error (RMSE) of 0.01075. The suggested method outperforms all the current methods, as demonstrated by a comparison of LSTM Approach Tuned by Salp Swarm Algorithm (V-LSTM-SSA-DO) and Forecasting Crude Oil Prices with a WT-FNN Model (WT-FNN).
MoreTranslated text
Key words
bayesian optimization,crude oil,forecasting,hierarchical,long short-term memory
AI Read Science
Must-Reading Tree
Example
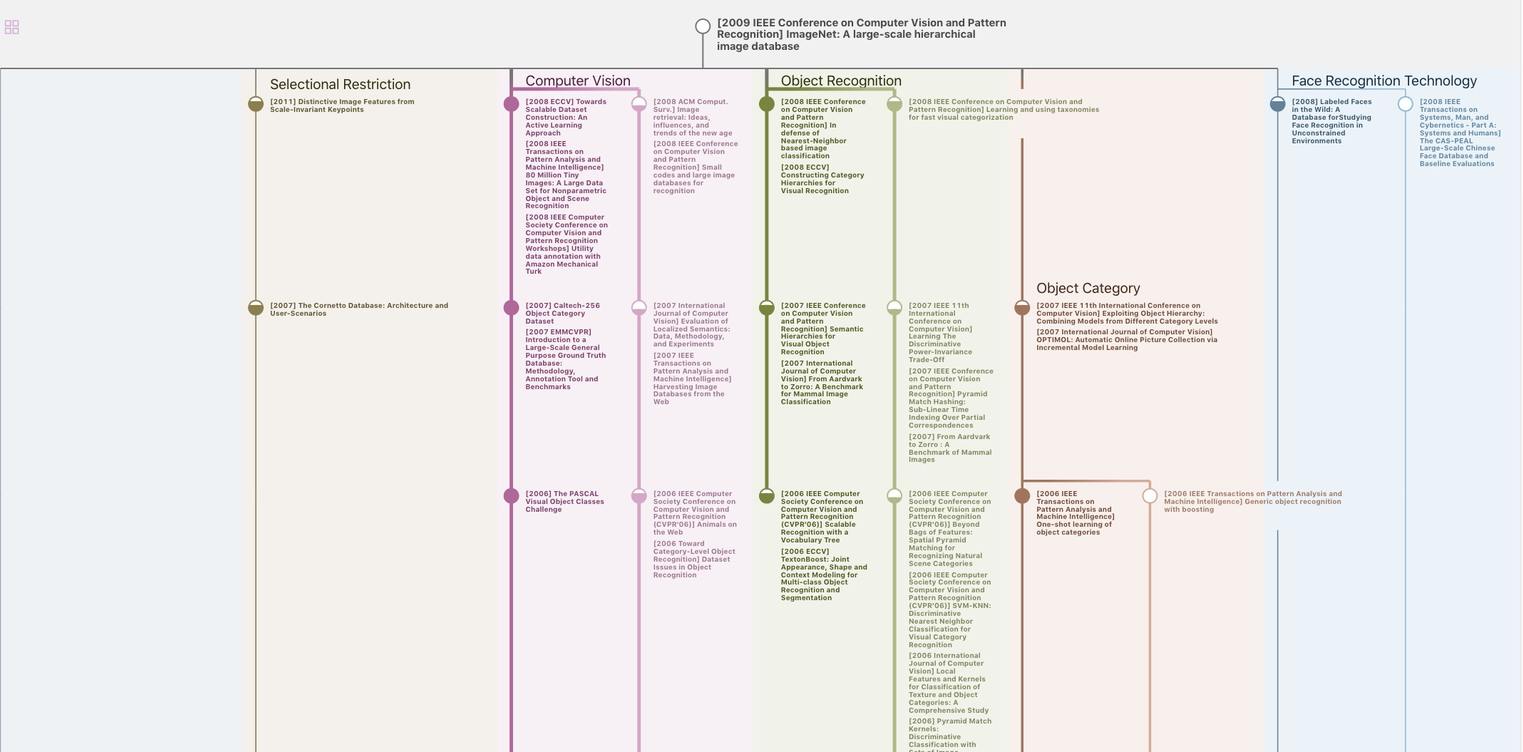
Generate MRT to find the research sequence of this paper
Chat Paper
Summary is being generated by the instructions you defined