F sign-Net: Depth Sensor Aggregated Frame-based Fourier Network for Sign Word Recognition
IEEE Sensors Journal(2024)
Abstract
Hand-tracking is a challenging problem during hand gesture recognition due to abnormal hand patterns across depth signs and errors between normal pixels and backgrounds. In this article, we propose
F
sign-Net: Fourier pixel-wise approach based on the Fourier Convolution Neural Network (FCNN) and Time-distributed-based Bi-directional Long-short-term Memory (BiLSTM). FCNNs have been widely researched, reaching state-of-the-art performance on spatial recognition tasks. However, it is still difficult for the Fourier model to learn the temporal patterns due to the chaotic nature of the hand motion data.
F
sign-Net aggregates time-based information from spatial and temporal modules to a given Fourier convolution in three stages: (1) Each depth frame is regarded as a window, and it is selected so that the aggregated sums of the pixels across the hand joints of the selected window are aligned, (2) a truncated pooling is applied that summarizes the generated featured map of the Fourier convolution to avoid over-fitting, and (3) the long-term temporal dependencies among pixels for the Fourier convolution are captured using the shared Time-based BiLSTM layers. This allows the proposed model to learn hand patterns that are temporally oriented. Finally, the proposed
F
net-Sign is evaluated on depth sign language public data sets and demonstrates state-of-the-art performance. Simulation results proved that improved Fourier features are good features for the proposed hand-tracking approach.
MoreTranslated text
Key words
Depth sensing,Fourier domain,Hand gesture,Leap motion sensor,Machine learning,Pattern recognition,3D sensor data,3D signal processing
AI Read Science
Must-Reading Tree
Example
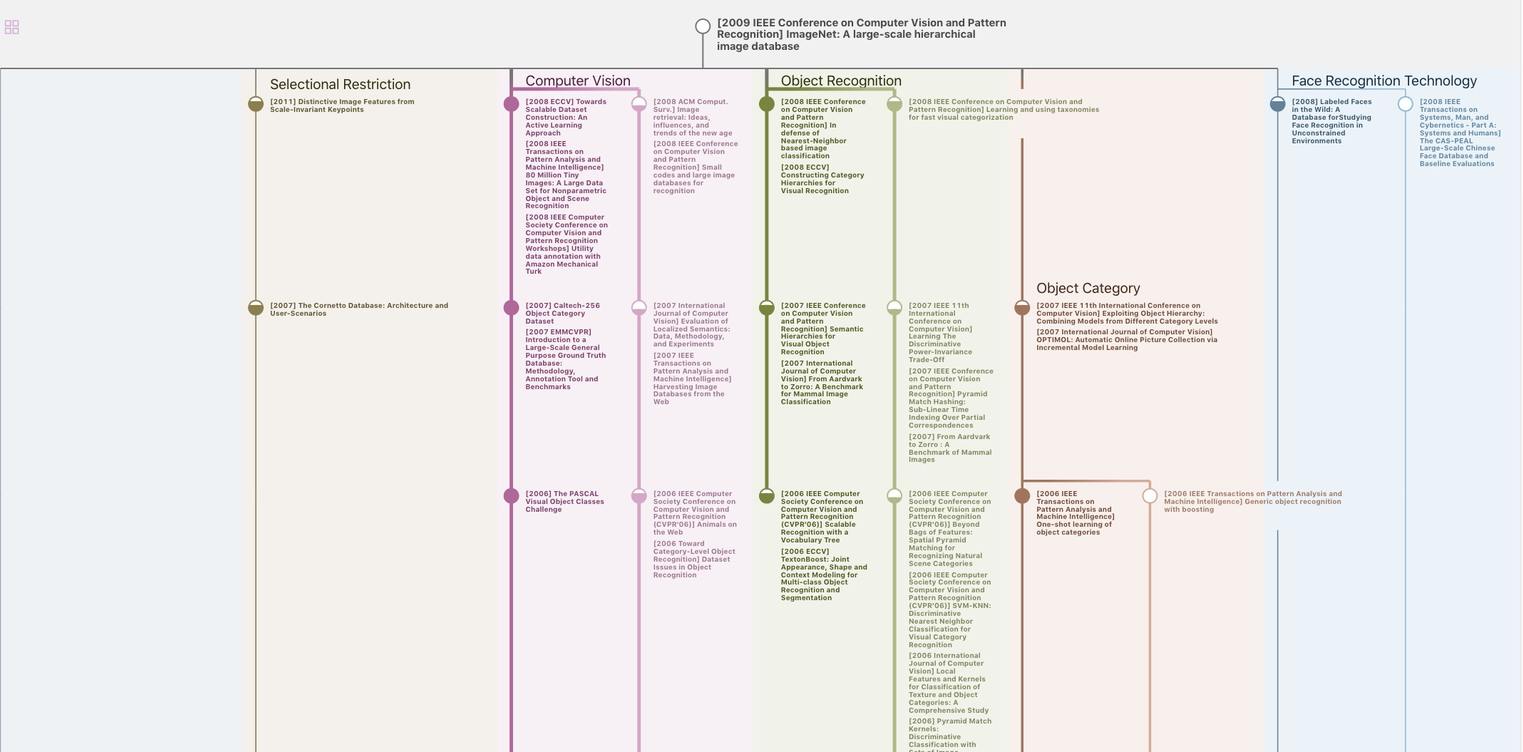
Generate MRT to find the research sequence of this paper
Chat Paper
Summary is being generated by the instructions you defined