Novel Confined Attention-Enabled Hierarchical Bi-LSTM and Bi-GRU Fusion: A Multiscale Traffic Flow Prediction Application
IEEE Transactions on Computational Social Systems(2024)
摘要
Traffic flow prediction is essential for alleviating congestion and enhancing traffic management. While previous efforts have mainly concentrated on road-level predictions, the advancement of technology and the growing presence of automated vehicles have encouraged lane-level forecasting for more efficient driving and routing decisions. Therefore, this work presents a hierarchical model using recurrent neural networks (RNNs) that takes the lane-level information as the input and makes multiscale (lane level and road level) traffic flow predictions. Initially, the lane-level predictions are made using confined attention enabled RNN (CAERNN), built using bidirection long short-term memory (Bi-LSTM) and bidirection gated recurrent unit (Bi-GRU), and separately learning the periodic and temporal characteristics. Further, the features extracted by each lane are used to predict the traffic flow on the entire road. A novel confined attention mechanism is integrated into the Bi-LSTM module to improve the model’s performance by focusing on recent, more relevant information in the traffic flow sequence. It is observed that the confined attention mechanism performs better than the conventional attention mechanism. Furthermore, the external features are integrated to improve the model’s performance. The proposed model is evaluated on publicly available real-world data sets (in normal and COVID-19 scenarios), and the compared results with several state-of-the-art methods are evidence for the CAERNN’s effectiveness.
更多查看译文
关键词
Attention,bidirection gated recurrent unit (Bi-GRU),bidirection long short-term memory (Bi-LSTM),deep learning,external features,traffic flow prediction
AI 理解论文
溯源树
样例
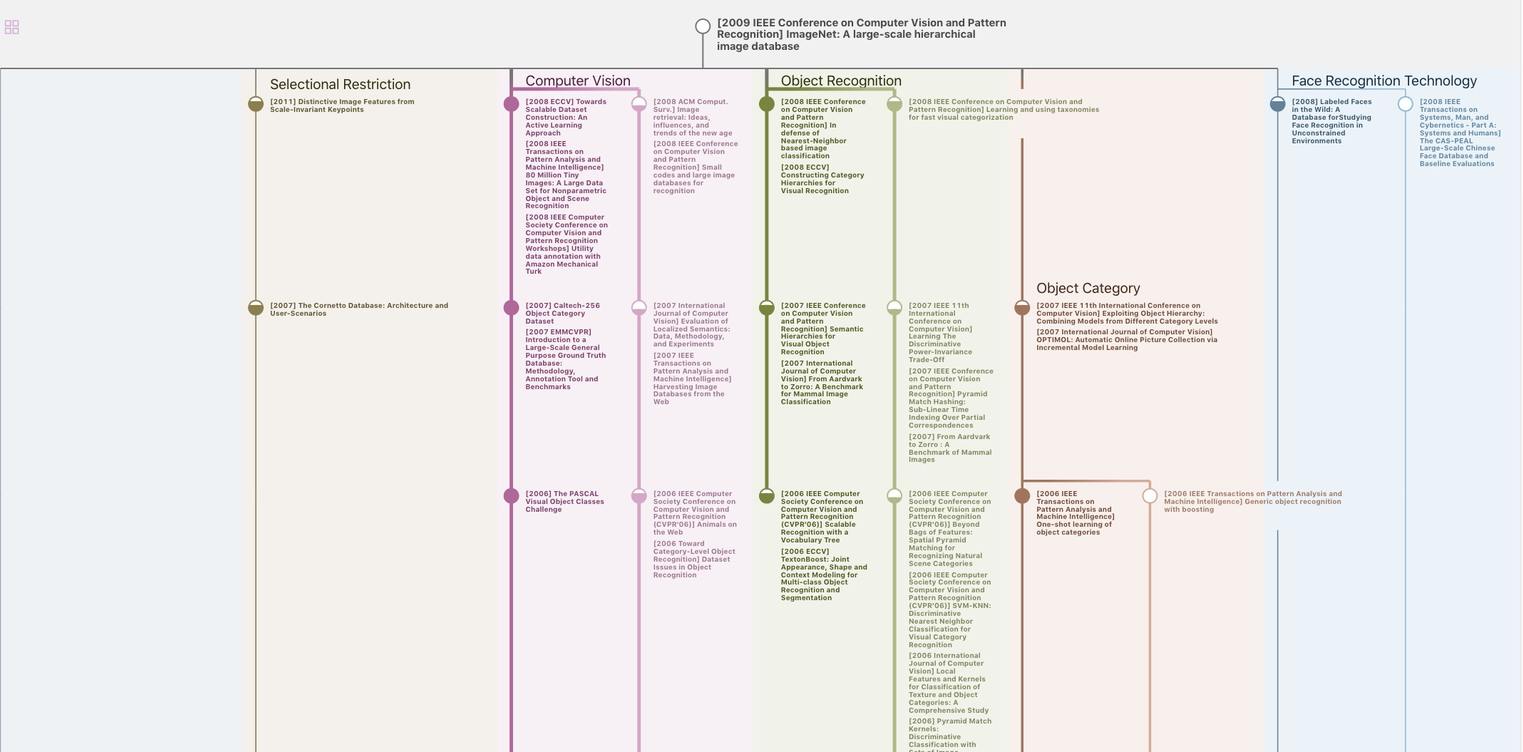
生成溯源树,研究论文发展脉络
Chat Paper
正在生成论文摘要