IRCNN: A novel signal decomposition approach based on iterative residue convolutional neural network
Pattern Recognition(2024)
摘要
The decomposition of non-stationary signals is an important and challenging task in the field of signal time-frequency analysis. In the recent two decades, many decomposition methods have been proposed, inspired by the empirical mode decomposition method, first published by Huang et al. in 1998. However, they still have some limitations. For example, they are generally prone to boundary and mode mixing effects and are not very robust to noise. Inspired by the successful applications of deep learning, and given the lack in the literature of works in which deep learning techniques are used directly to decompose non-stationary signals into simple oscillatory components, we use the convolutional neural network, residual structure and nonlinear activation function to compute in an innovative way the local average of the signal, and study a new non-stationary signal decomposition method under the framework of deep learning. We discuss the training process of the proposed model and study the convergence analysis of the learning algorithm. In the experiments, we evaluate the performance of the proposed model from two points of view: the calculation of the local average and the signal decomposition. Furthermore, we study the mode mixing, noise interference, and orthogonality properties of the decomposed components produced by the proposed method, and compare it with the state-of-the-art ones. All results show that the proposed model allows for better handling boundary effect, mode mixing effect, robustness, and the orthogonality of the decomposed components than existing methods.
更多查看译文
关键词
Empirical mode decomposition,Adaptive signal decomposition,Signal local average,Convolutional neural network,Residual network
AI 理解论文
溯源树
样例
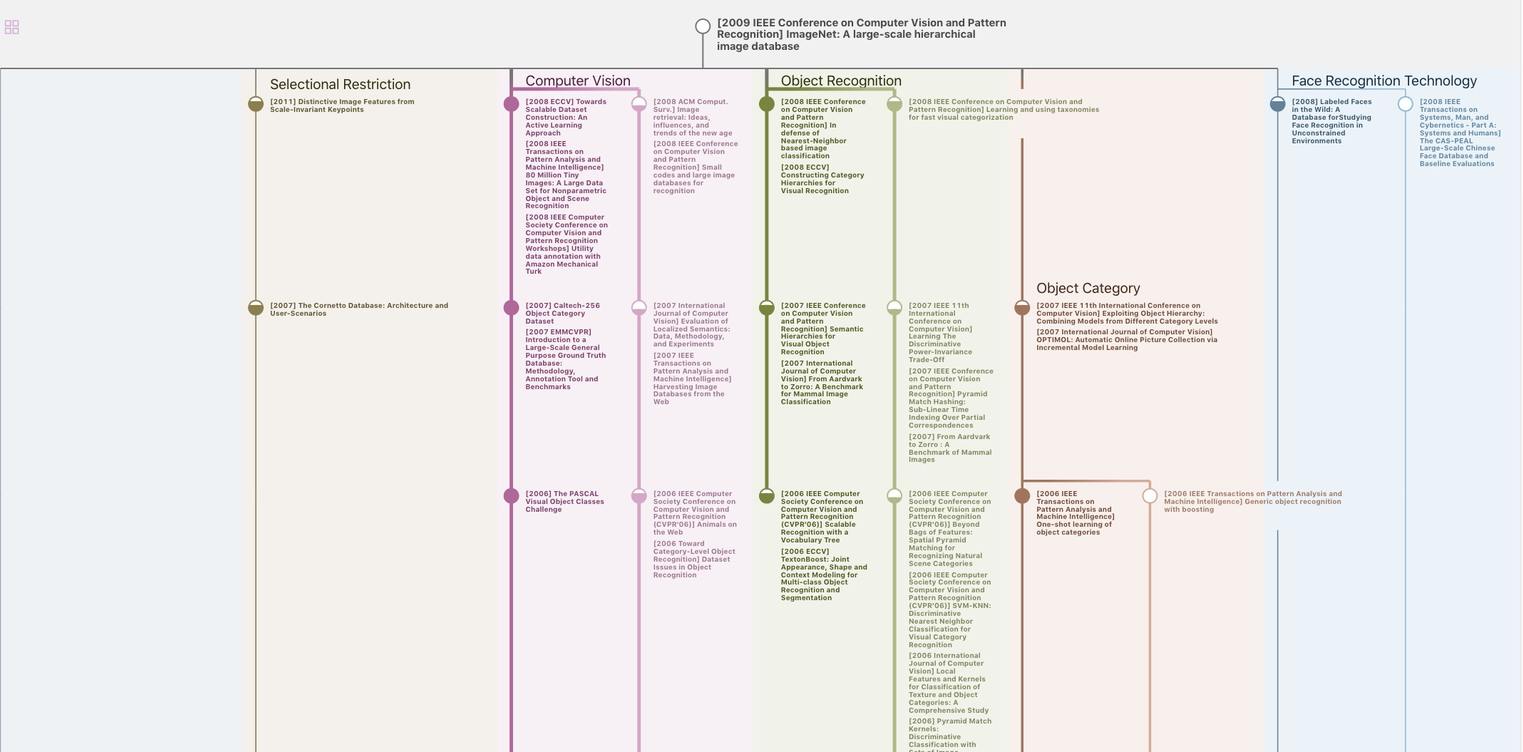
生成溯源树,研究论文发展脉络
Chat Paper
正在生成论文摘要