Energy Efficient Memristive Transiently Chaotic Neural Network for Combinatorial Optimization
IEEE Transactions on Circuits and Systems I: Regular Papers(2024)
Abstract
The utilization of memristive analog-digital mixed in-memory computing has significantly tackled the issues of massive computing resources and time delays in solving combinatorial optimization problems. However, further improvements in computing energy efficiency are still desirable for resource-constrained conditions and practical applications. Therefore, in this work, a memristive analog transiently chaotic neural network (TCNN) system is proposed to solve the traveling salesman problems (TSPs), which is consisted of 1) a memristor array to perform matrix-vector multiplication for the network iteration; 2) neuronal modules and nonlinear activation function modules to emulate the basic functions of the network; 3) chaotic simulated annealing (CSA) modules to improve the solution performance at extremely low hardware overhead. Based on these, after mapping the TSPs onto the memristor array, the proposed TCNN can self-iterate to convergence states to solve the problems with high performance. Compared to prior analog-digital mixed ones, the analog system can eliminate the extra control and data conversions during the network solving process, and achieve an 8
$\times$
reduction in the total energy consumption in consecutive solving tasks.
MoreTranslated text
Key words
Computing in-memory,memristor,analog computing,combinatorial optimization problems,transiently chaotic neural network
AI Read Science
Must-Reading Tree
Example
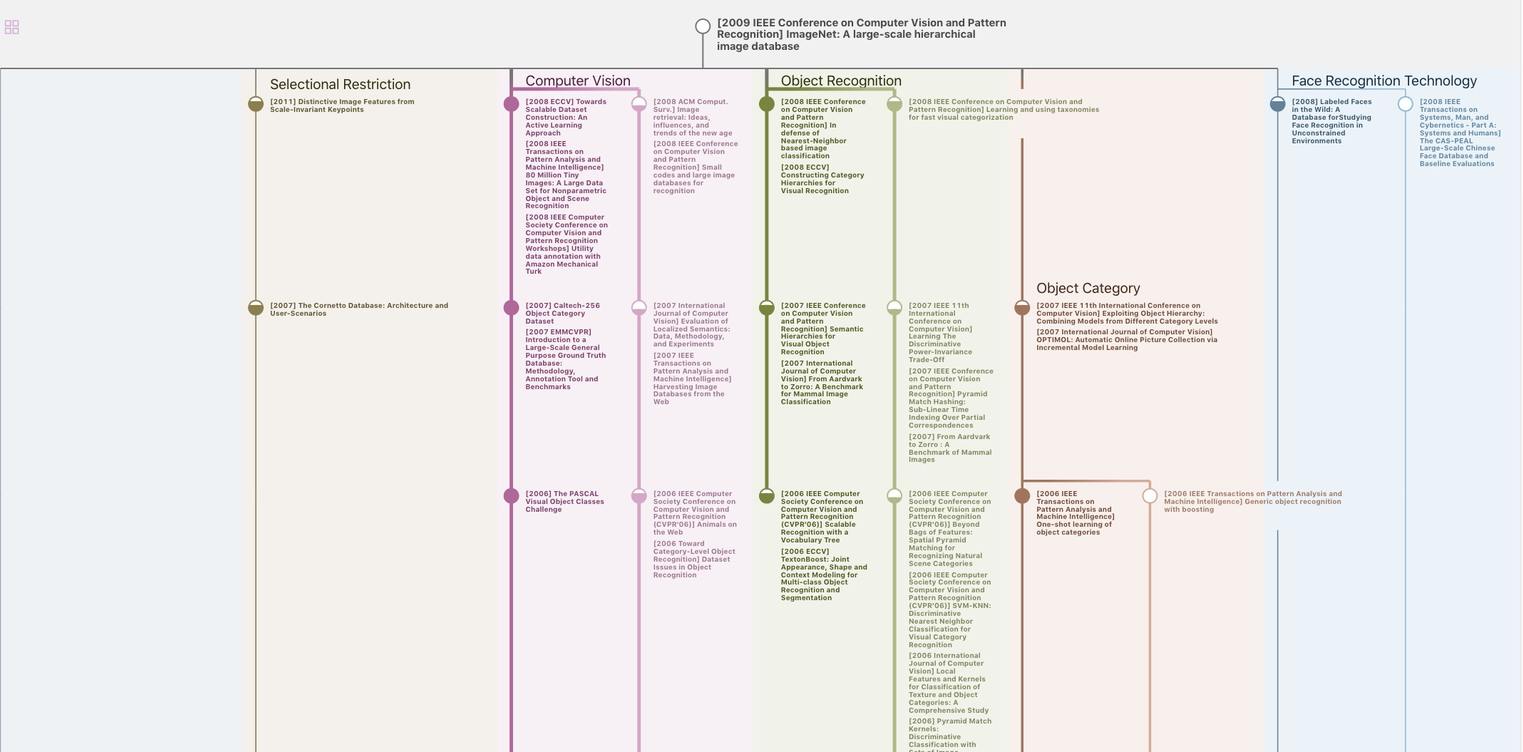
Generate MRT to find the research sequence of this paper
Chat Paper
Summary is being generated by the instructions you defined