When Do Skills Help Reinforcement Learning? A Theoretical Analysis of Temporal Abstractions
ICML 2024(2024)
Abstract
Skills are temporal abstractions that are intended to improve reinforcement learning (RL) performance through hierarchical RL. Despite our intuition about the properties of an environment that make skills useful, a precise characterization has been absent. We provide the first such characterization, focusing on the utility of deterministic skills in deterministic sparse-reward environments with finite action spaces. We show theoretically and empirically that RL performance gain from skills is worse in environments where solutions to states are less compressible. Additional theoretical results suggest that skills benefit exploration more than they benefit learning from existing experience, and that using unexpressive skills such as macroactions may worsen RL performance. We hope our findings can guide research on automatic skill discovery and help RL practitioners better decide when and how to use skills.
MoreTranslated text
AI Read Science
Must-Reading Tree
Example
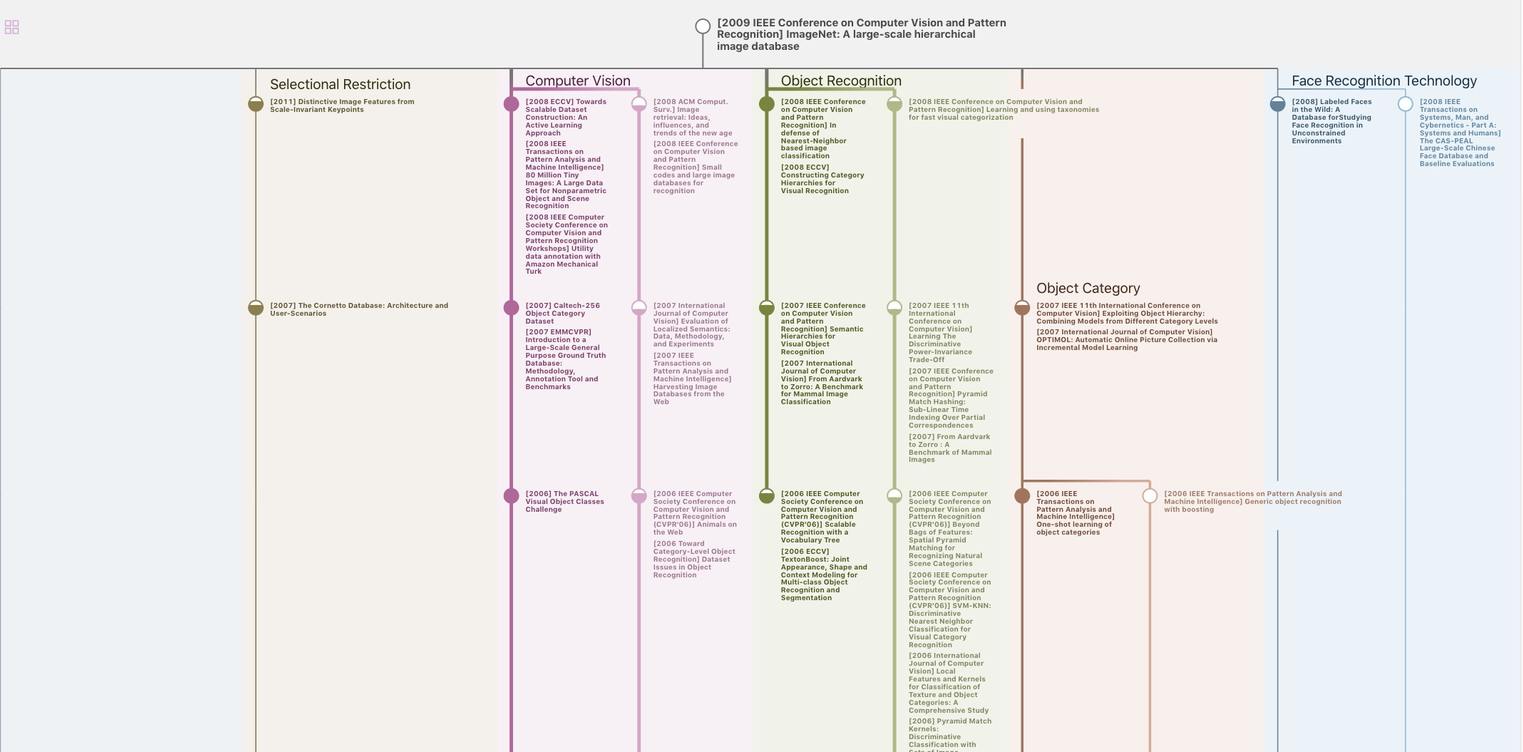
Generate MRT to find the research sequence of this paper
Chat Paper
Summary is being generated by the instructions you defined