Joint Super-resolution and Tissue Patch Classification for Whole Slide Histological Images
Programming and Computer Software(2024)
摘要
Segmentation of whole slide histological images through the classification of tissue types of small fragments is an extremely relevant task in digital pathology, necessary for the development of methods for automatic analysis of whole slide histological images. The extremely large resolution of such images also makes the task of increasing image resolution relevant, which allows storing images at a reduced resolution and increasing it if necessary. Annotating whole slide images by histologists is complex and time-consuming, so it is important to make the most efficient use of the available data, both labeled and unlabeled. In this paper we propose a novel neural network method to simultaneously solve the problems of super-resolution of histological images from ×20 optical magnification to ×40 and classifying image fragments into tissue types at ×20 magnification. The use of a single encoder as well as the proposed neural network training scheme allows to achieve better results on both tasks compared to existing approaches. The PATH-DT-MSU WSS2v2 dataset presented for the first time in this paper was used for training and testing the method. On the test sample, an accuracy value of 0.971 and a balanced accuracy value of 0.916 were achieved in the classification task on 5 tissue types, for the super-resolution task, values of PSNR = 32.26 and SSIM = 0.89 were achieved. The source code of the proposed method is available at: https://github.com/Kukty/WSI_SR_CL .
更多查看译文
关键词
histology,deep learning,convolutional neural networks,super-resolution,tissue type classification,wholeslide images
AI 理解论文
溯源树
样例
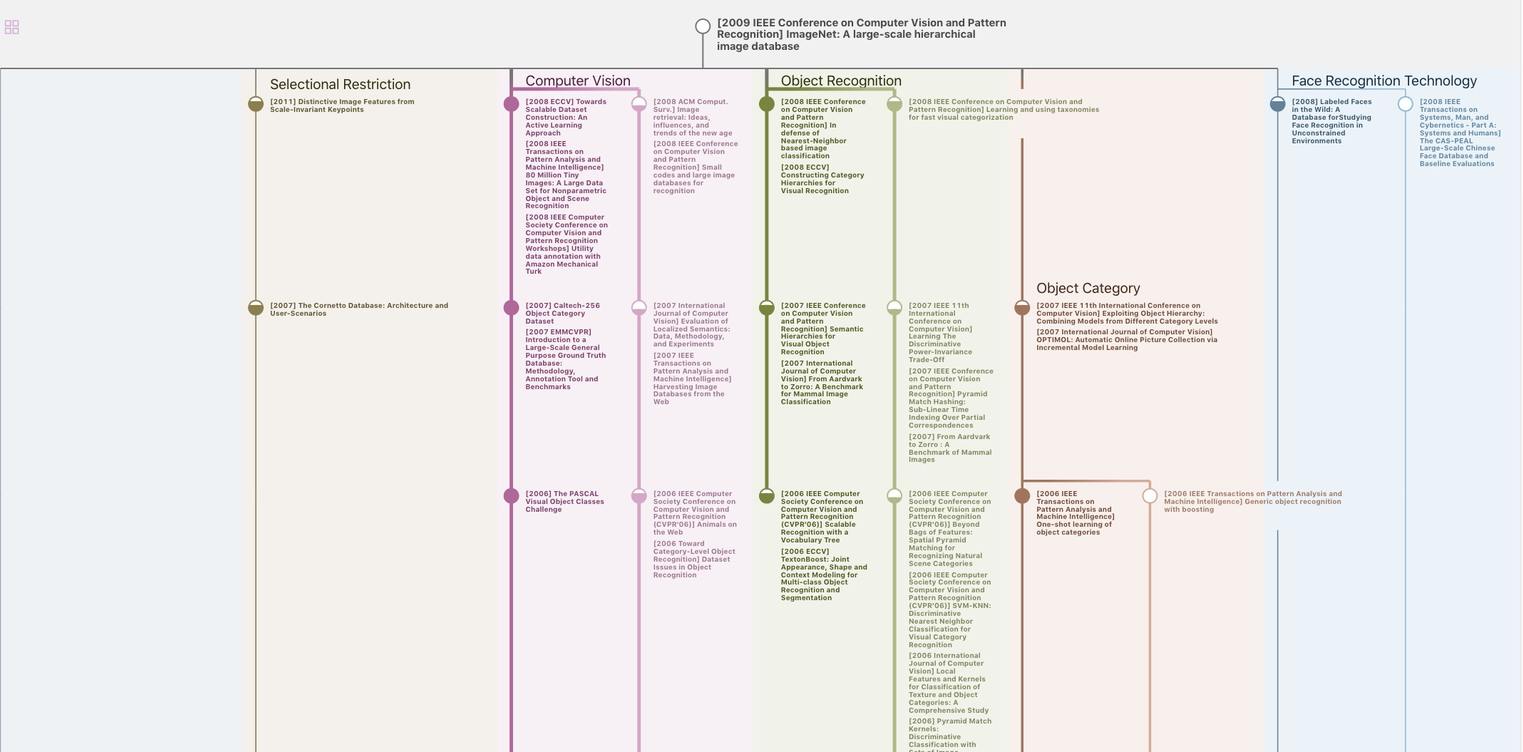
生成溯源树,研究论文发展脉络
Chat Paper
正在生成论文摘要