Revolutionizing Intelligent Reflecting Surfaces: Deep Learning-Driven Channel Estimation
2024 2nd International Conference on Advancement in Computation & Computer Technologies (InCACCT)(2024)
摘要
The low signal strength at the non line-of-sight users or edge users in the wireless communication network results in call drops and interrupted communication. Intelligent Reflecting Surface (IRS) is a transformative Sixth Generation (6G) technology that offers robust wireless transmission capabilities by enabling smart radio. But the estimation of accurate channel information is the main challenge owing to the large number of reflecting elements. This paper presents a Deep Learning (DL) driven channel estimation technique for an IRS-aided multi-user network. The setup considers a predominant proportion of IRS elements to be passive with a subset of active elements. The proposed scheme offers an efficient solution and aims to reduce the training overhead. It is shown that the proposed DL model obtains an accuracy of 94% in estimating the channel. Further, the paper conducts a comparative analysis between the proposed deep neural network approach and a conventional scheme. The performance is evaluated for achievable rate under different training samples, number of active elements and transmit power. The results reveal that the DL approach consistently outperforms the conventional method.
更多查看译文
关键词
Channel estimation,5G,deep learning,hybrid IRS,cascaded IRS,achievable rate
AI 理解论文
溯源树
样例
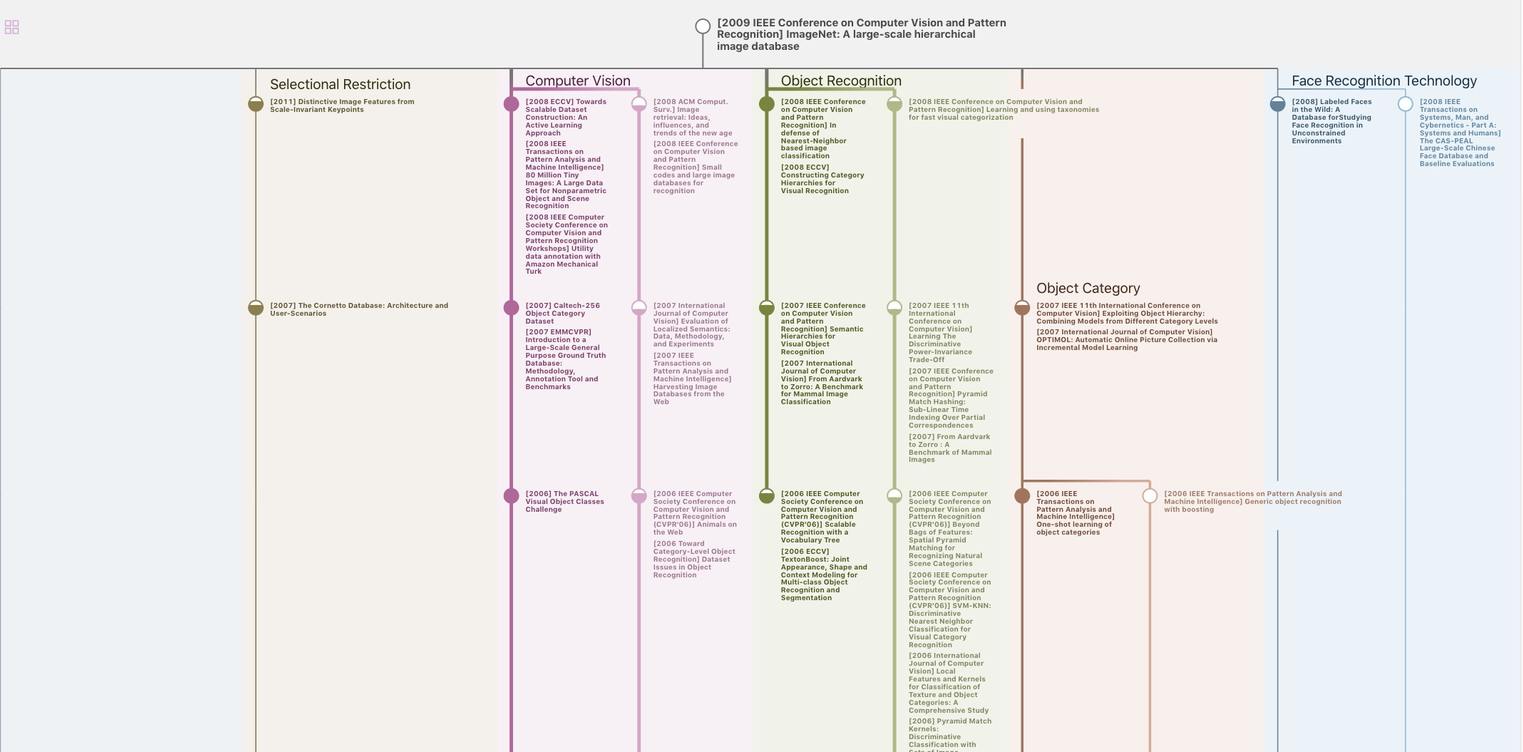
生成溯源树,研究论文发展脉络
Chat Paper
正在生成论文摘要