A System and Method for Fruit Ripeness Prediction Using Transfer Learning and CNN
2024 International Conference on Communication, Computing and Internet of Things (IC3IoT)(2024)
摘要
Accurate fruit ripeness prediction is critical for farmers, distributors, and retailers to optimize the food supply chain. Traditional visual inspection methods are subjective, inconsistent, and labor-intensive. This paper proposes a novel system that leverages transfer learning and Convolutional Neural Networks (CNNs) to achieve automated and objective fruit ripeness prediction. Transfer learning significantly reduces training time by utilizing a pre-trained CNN model, which has already learned valuable features from a massive image dataset. CNNs, particularly adept at image recognition, automatically extract relevant features like color, texture, and shapes from fruit images. These features allow the CNN to learn the telltale signs of ripeness and accurately predict the maturity of new fruit images. The proposed system offers several advantages: improved accuracy compared to subjective human assessments, reduced reliance on expert labor, and scalability to handle different fruit types by fine-tuning the model with specific fruit image datasets. Overall, this system utilizing transfer learning and CNNs presents a promising approach for efficient, objective, and cost-effective fruit ripeness prediction in the food supply chain.
更多查看译文
关键词
Transfer Learning,SoftMax Classifiers,Normalization,Convolutional Neural Networks (CNN),Deep Learning
AI 理解论文
溯源树
样例
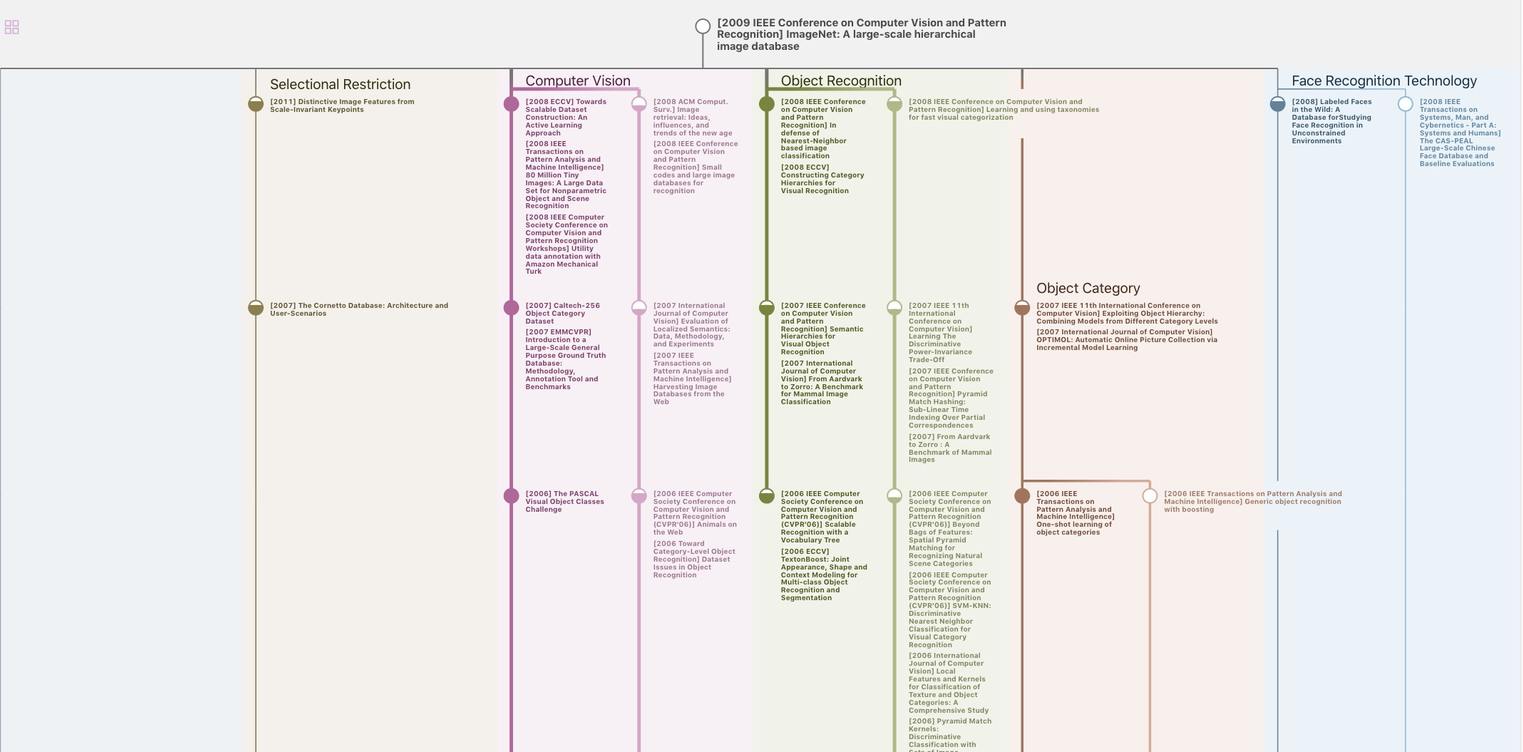
生成溯源树,研究论文发展脉络
Chat Paper
正在生成论文摘要