A novel Bayesian deep learning method for fast wake field prediction of the DARPA SUBOFF
Applied Ocean Research(2024)
Abstract
The accurate and rapid prediction of wake flow characteristics is of great significance for the design of underwater vehicles. This paper develops a data driven Bayesian deep learning framework, named WakeNet, to accurately and quickly predict the wake velocity field on the paddle disk surface of the DARPA SUBOFF model under varying geometry configurations. Specifically, (i) the proposed WakeNet presents a coordinate affine transformation technique to address the issue of handling unstructured wake flow data with varying geometries; (ii) it develops a residual modeling strategy built upon a modern version of the U-net architecture to capture the multi-scale wake flow characteristics; and finally, (iii) it exploits the MC dropout method to achieve uncertainty quantification of the prediction, and builds the uncertainty based active learning framework to effectively update the model. Numerical experiments have verified the capability of the proposed WakeNet model for the accurate prediction and uncertainty quantification of the wake flow field with varying geometries. Besides, the proposed model yields a speed up factor of 7000× compared to the conventional numerical simulation solver.
MoreTranslated text
Key words
SUBOFF,Paddle disk surface,Wake field,Bayesian deep learning,Convolutional neural network,Uncertainty quantification
AI Read Science
Must-Reading Tree
Example
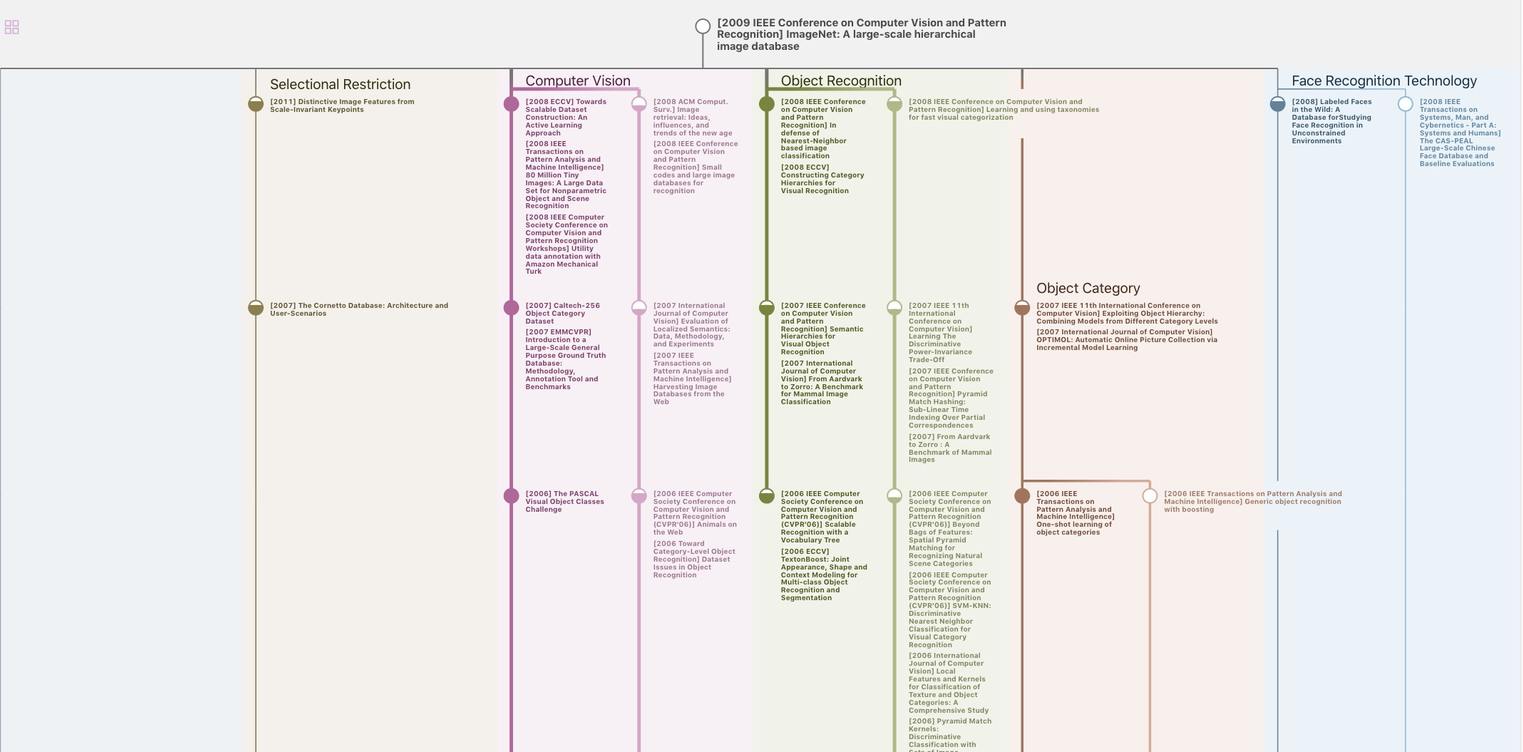
Generate MRT to find the research sequence of this paper
Chat Paper
Summary is being generated by the instructions you defined