InfoUCL: Learning Informative Representations for Unsupervised Continual Learning
IEEE Transactions on Multimedia(2024)
摘要
Unsupervised continual learning (UCL) has made remarkable progress over the past two years, significantly expanding the application of continual learning (CL). However, existing UCL approaches have only focused on transferring continual strategies from supervised to unsupervised. They have overlooked the relationship issue between visual features and representational continuity. This work draws attention to the texture bias problem in existing UCL methods. To address this problem, we propose a new UCL framework called InfoUCL, in which we develop InfoDrop contrastive loss to guide continual learners to extract more informative shape features of objects and discard useless texture features simultaneously. The proposed InfoDrop contrastive loss is general and can be combined with various UCL methods. Extensive experiments on various benchmarks have demonstrated that our InfoUCL framework can lead to higher classification accuracy and superior robustness to catastrophic forgetting. Our code is available at https://github.com/learninginvision/InfoUCL
更多查看译文
关键词
Unsupervised continual learning,informative dropout,self-supervised learning
AI 理解论文
溯源树
样例
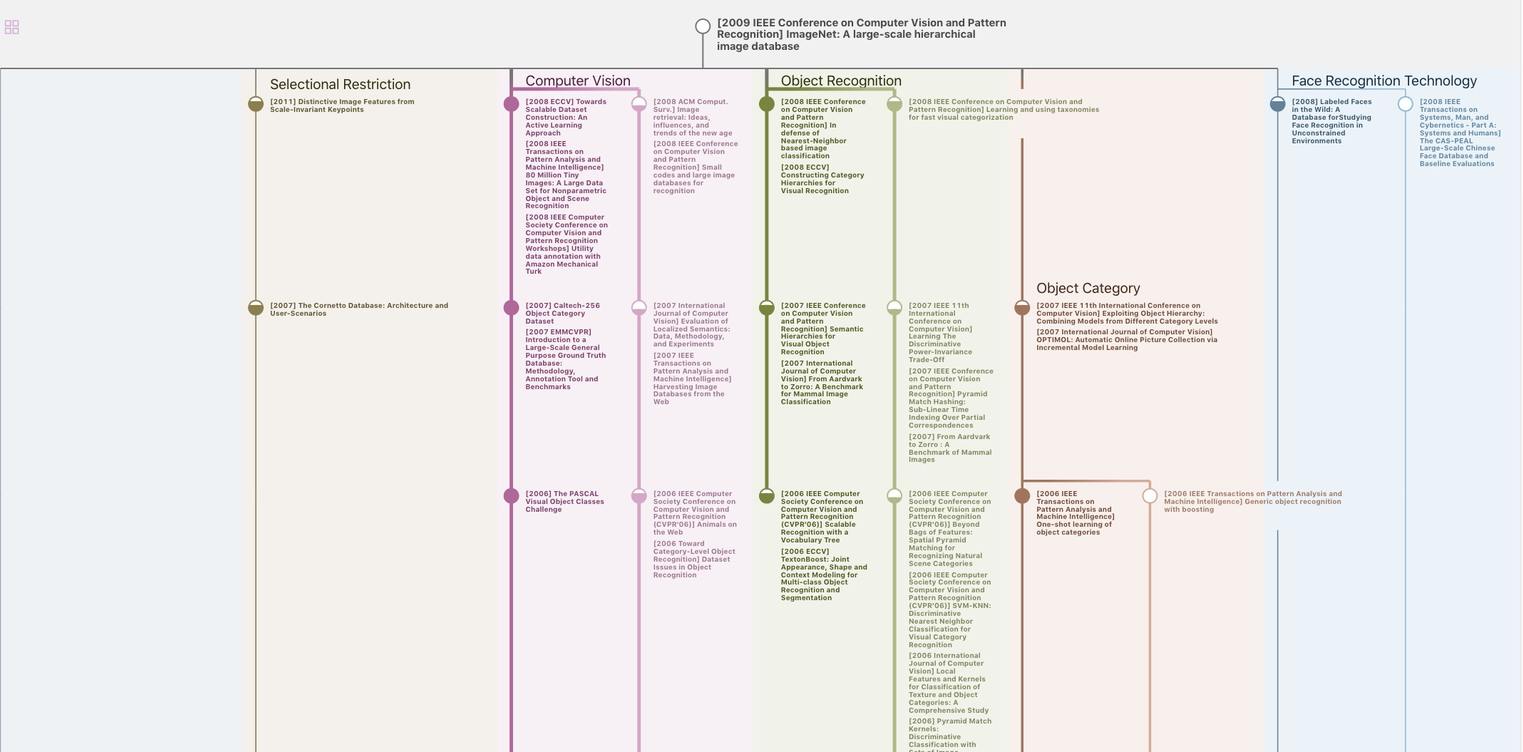
生成溯源树,研究论文发展脉络
Chat Paper
正在生成论文摘要