Mutual Knowledge Distillation based Personalized Federated Learning for Smart Edge Computing
IEEE Transactions on Consumer Electronics(2024)
Abstract
Federated Learning (FL) is a privacy-preserving machine learning paradigm that aims to train a global model using heterogeneous data across clients, which are typically consumer electronic devices such as smartphones, smart vehicles, and smart home appliances. As the global model may not be optimal for individual clients with unique behaviours, Personalized Federated Learning (PFL) was proposed to enable clients to adapt the global model to their specific needs and preferences. Nonetheless, due to the variance in data distributions across clients, the global model utilized in PFL may ‘catastrophically forget’ the knowledge gained in previous communication rounds, thereby leading to unstable performance. To address this challenge, we propose FedMKD, a novel PFL algorithm based on Mutual Knowledge Distillation (MKD) and elastic weight consolidation (EWC). FedMKD enhances the global model’s performance by addressing ‘catastrophic forgetting’ through EWC regularization, while enabling clients’ local models to effectively leverage the global model’s knowledge via MKD. Moreover, we apply uniform/exponential quantization methods to compress model parameters to decrease communication overheads. Experimental results demonstrate that FedMKD outperforms several key PFL baselines, FedMKD can also significantly reduce communication overhead while preserving its performance using suitable compression techniques, making it highly suitable for resource-constrained smart edge computing environment.
MoreTranslated text
Key words
Personalized Federated Learning,Knowledge Distillation,Deep Learning,Edge Computing
AI Read Science
Must-Reading Tree
Example
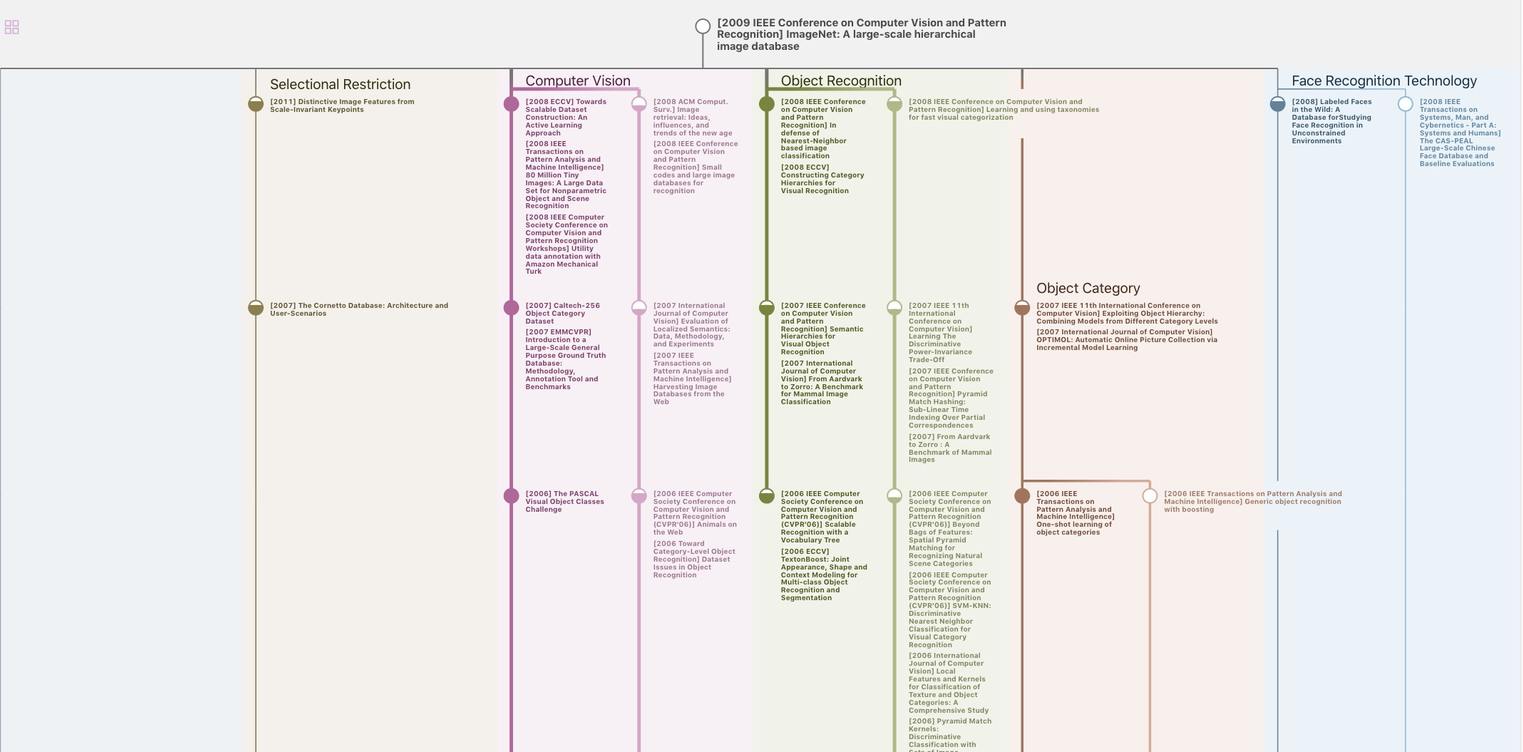
Generate MRT to find the research sequence of this paper
Chat Paper
Summary is being generated by the instructions you defined