SEP-AlgPro: An efficient allergen prediction tool utilizing traditional machine learning and deep learning techniques with protein language model features
International Journal of Biological Macromolecules(2024)
摘要
Allergy is a hypersensitive condition in which individuals develop objective symptoms when exposed to harmless substances at a dose that would cause no harm to a “normal” person. Most current computational methods for allergen identification rely on homology or conventional machine learning using limited set of feature descriptors or validation on specific datasets, making them inefficient and inaccurate. Here, we propose SEP-AlgPro for the accurate identification of allergen protein from sequence information. We analyzed 10 conventional protein-based features and 14 different features derived from protein language models to gauge their effectiveness in differentiating allergens from non-allergens using 15 different classifiers. However, the final optimized model employs top 10 feature descriptors with top seven machine learning classifiers. Results show that the features derived from protein language models exhibit superior discriminative capabilities compared to traditional feature sets. This enabled us to select the most discriminatory baseline models, whose predicted outputs were aggregated and used as input to a deep neural network for the final allergen prediction. Extensive case studies showed that SEP-AlgPro outperforms state-of-the-art predictors in accurately identifying allergens. A user-friendly web server was developed and made freely available at https://balalab-skku.org/SEP-AlgPro/, making it a powerful tool for identifying potential allergens.
更多查看译文
关键词
Allergen,Deep neural network,Machine learning,Stacking framework,Sequence analysis
AI 理解论文
溯源树
样例
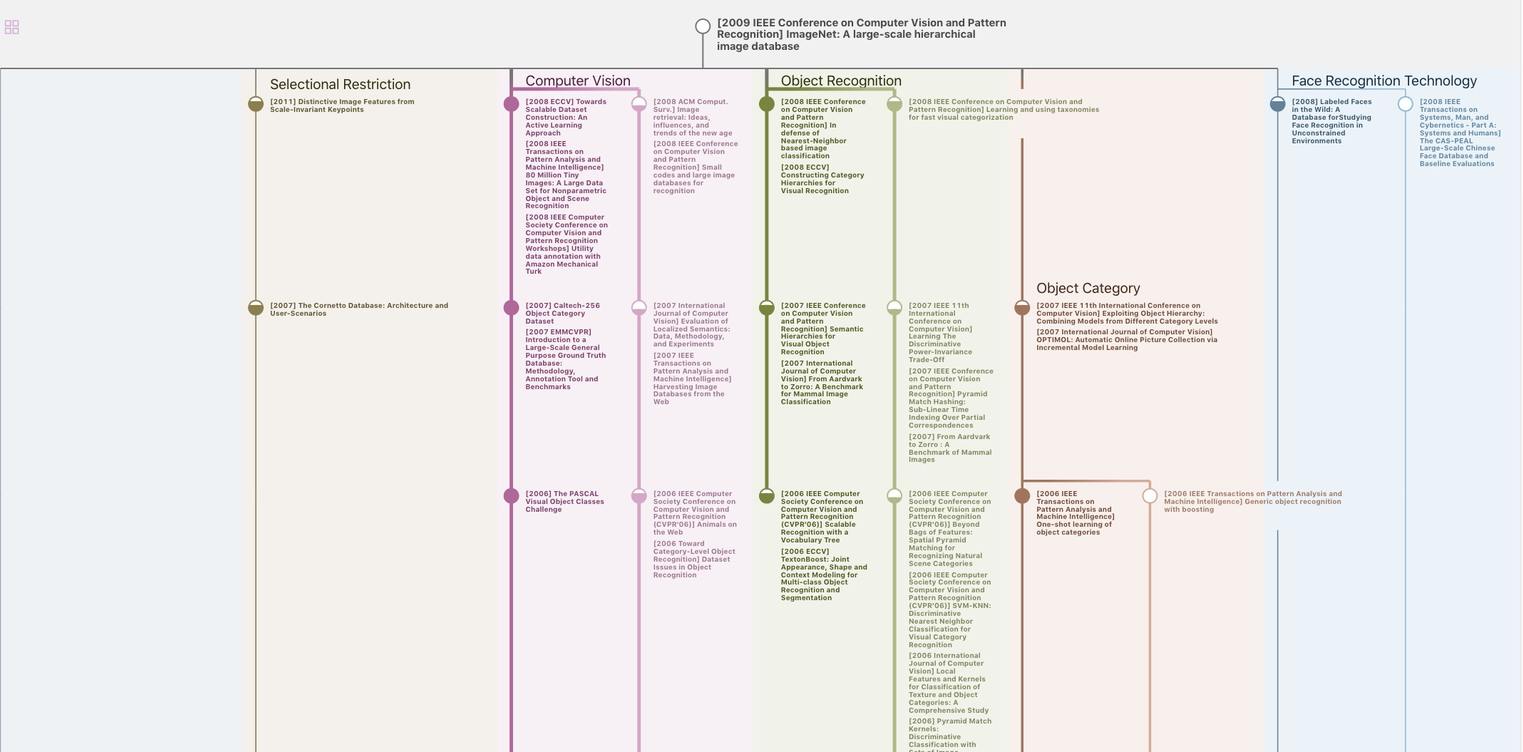
生成溯源树,研究论文发展脉络
Chat Paper
正在生成论文摘要