Disrupting Bipartite Trading Networks: Matching for Revenue Maximization
CoRR(2024)
Abstract
We model the role of an online platform disrupting a market with unit-demand
buyers and unit-supply sellers. Each seller can transact with a subset of the
buyers whom she already knows, as well as with any additional buyers to whom
she is introduced by the platform. Given these constraints on trade, prices and
transactions are induced by a competitive equilibrium. The platform's revenue
is proportional to the total price of all trades between platform-introduced
buyers and sellers.
In general, we show that the platform's revenue-maximization problem is
computationally intractable. We provide structural results for revenue-optimal
matchings and isolate special cases in which the platform can efficiently
compute them. Furthermore, in a market where the maximum increase in social
welfare that the platform can create is Δ W, we prove that the platform
can attain revenue Ω(Δ W/log(min{n,m})), where n and m are
the numbers of buyers and sellers, respectively. When Δ W is large
compared to welfare without the platform, this gives a polynomial-time
algorithm that guarantees a logarithmic approximation of the optimal welfare as
revenue. We also show that even when the platform optimizes for revenue, the
social welfare is at least an O(log(min{n,m}))-approximation to the
optimal welfare. Finally, we prove significantly stronger bounds for revenue
and social welfare in homogeneous-goods markets.
MoreTranslated text
AI Read Science
Must-Reading Tree
Example
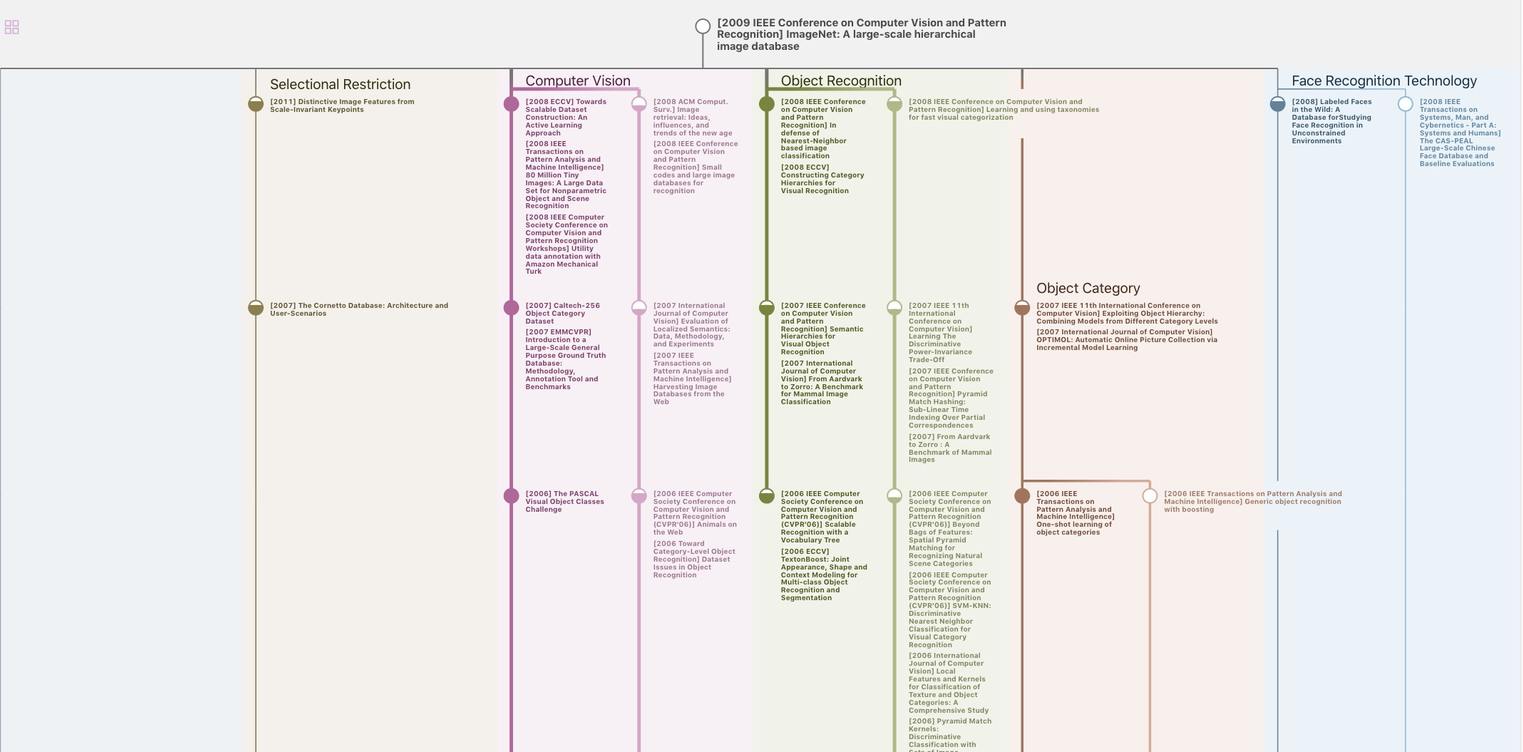
Generate MRT to find the research sequence of this paper
Chat Paper
Summary is being generated by the instructions you defined