Reinforced Compressive Neural Architecture Search for Versatile Adversarial Robustness
CoRR(2024)
Abstract
Prior neural architecture search (NAS) for adversarial robustness works have
discovered that a lightweight and adversarially robust neural network
architecture could exist in a non-robust large teacher network, generally
disclosed by heuristic rules through statistical analysis and neural
architecture search, generally disclosed by heuristic rules from neural
architecture search. However, heuristic methods cannot uniformly handle
different adversarial attacks and "teacher" network capacity. To solve this
challenge, we propose a Reinforced Compressive Neural Architecture Search
(RC-NAS) for Versatile Adversarial Robustness. Specifically, we define task
settings that compose datasets, adversarial attacks, and teacher network
information. Given diverse tasks, we conduct a novel dual-level training
paradigm that consists of a meta-training and a fine-tuning phase to
effectively expose the RL agent to diverse attack scenarios (in meta-training),
and making it adapt quickly to locate a sub-network (in fine-tuning) for any
previously unseen scenarios. Experiments show that our framework could achieve
adaptive compression towards different initial teacher networks, datasets, and
adversarial attacks, resulting in more lightweight and adversarially robust
architectures.
MoreTranslated text
AI Read Science
Must-Reading Tree
Example
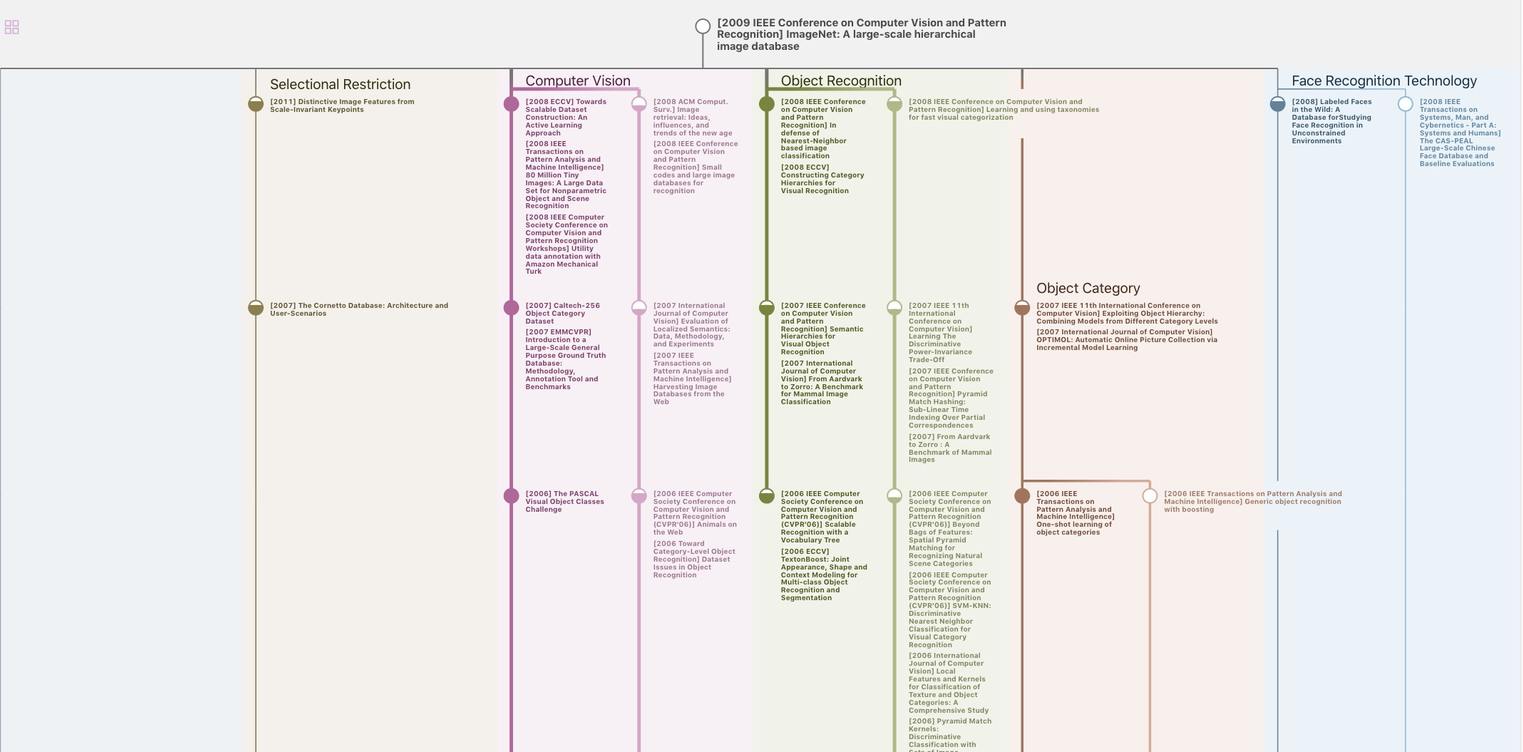
Generate MRT to find the research sequence of this paper
Chat Paper
Summary is being generated by the instructions you defined