A generic and robust quantum agent inspired by deep meta-reinforcement learning
arxiv(2024)
摘要
Deep reinforcement learning (deep RL) has enabled human- or superhuman-
performances in various applications. Recently, deep RL has also been adopted
to improve the performance of quantum control. However, a large volume of data
is typically required to train the neural network in deep RL, making it
inefficient compared with the traditional optimal quantum control method. Here,
we thus develop a new training algorithm inspired by the deep
meta-reinforcement learning (deep meta-RL), which requires significantly less
training data. The trained neural network is adaptive and robust. In addition,
the algorithm proposed by us has been applied to design the Hadamard gate and
show that for a wide range of parameters the infidelity of the obtained gate
can be made of the order 0.0001. Our algorithm can also automatically adjust
the number of pulses required to generate the target gate, which is different
from the traditional optimal quantum control method which typically fixes the
number of pulses a-priory. The results of this paper can pave the way towards
constructing a universally robust quantum agent catering to the different
demands in quantum technologies.
更多查看译文
AI 理解论文
溯源树
样例
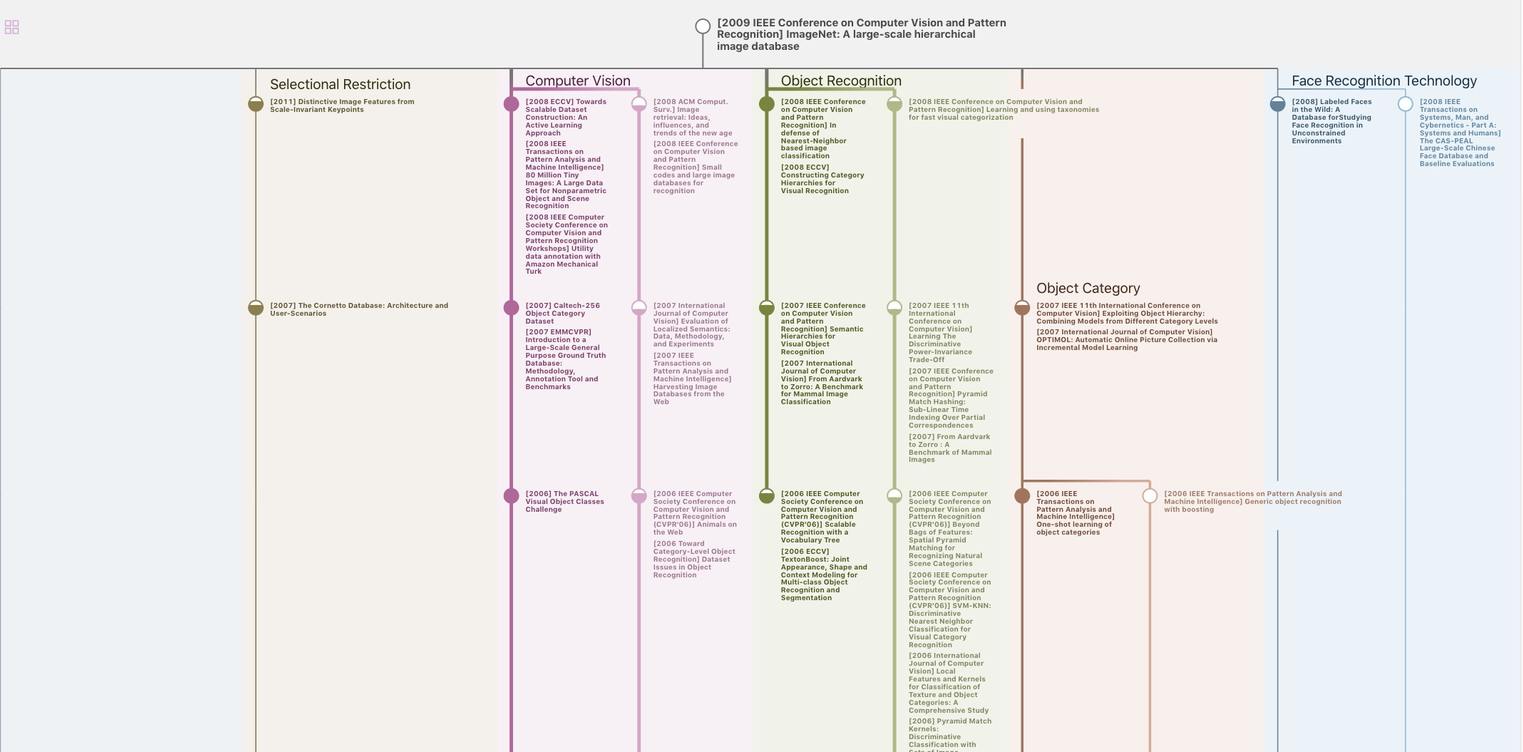
生成溯源树,研究论文发展脉络
Chat Paper
正在生成论文摘要