Parallel CNN-Deep Learning Clinical-Imaging Signature for Assessing Pathologic Grade and Prognosis of Soft Tissue Sarcoma Patients.
Journal of magnetic resonance imaging : JMRI(2024)
摘要
BACKGROUND:Traditional biopsies pose risks and may not accurately reflect soft tissue sarcoma (STS) heterogeneity. MRI provides a noninvasive, comprehensive alternative.
PURPOSE:To assess the diagnostic accuracy of histological grading and prognosis in STS patients when integrating clinical-imaging parameters with deep learning (DL) features from preoperative MR images.
STUDY TYPE:Retrospective/prospective.
POPULATION:354 pathologically confirmed STS patients (226 low-grade, 128 high-grade) from three hospitals and the Cancer Imaging Archive (TCIA), divided into training (n = 185), external test (n = 125), and TCIA cohorts (n = 44). 12 patients (6 low-grade, 6 high-grade) were enrolled into prospective validation cohort.
FIELD STRENGTH/SEQUENCE:1.5 T and 3.0 T/Unenhanced T1-weighted and fat-suppressed-T2-weighted.
ASSESSMENT:DL features were extracted from MR images using a parallel ResNet-18 model to construct DL signature. Clinical-imaging characteristics included age, gender, tumor-node-metastasis stage and MRI semantic features (depth, number, heterogeneity at T1WI/FS-T2WI, necrosis, and peritumoral edema). Logistic regression analysis identified significant risk factors for the clinical model. A DL clinical-imaging signature (DLCS) was constructed by incorporating DL signature with risk factors, evaluated for risk stratification, and assessed for progression-free survival (PFS) in retrospective cohorts, with an average follow-up of 23 ± 22 months.
STATISTICAL TESTS:Logistic regression, Cox regression, Kaplan-Meier curves, log-rank test, area under the receiver operating characteristic curve (AUC),and decision curve analysis. A P-value <0.05 was considered significant.
RESULTS:The AUC values for DLCS in the external test, TCIA, and prospective test cohorts (0.834, 0.838, 0.819) were superior to clinical model (0.662, 0.685, 0.694). Decision curve analysis showed that the DLCS model provided greater clinical net benefit over the DL and clinical models. Also, the DLCS model was able to risk-stratify patients and assess PFS.
DATA CONCLUSION:The DLCS exhibited strong capabilities in histological grading and prognosis assessment for STS patients, and may have potential to aid in the formulation of personalized treatment plans.
LEVEL OF EVIDENCE: 4:
TECHNICAL EFFICACY:Stage 2.
更多查看译文
AI 理解论文
溯源树
样例
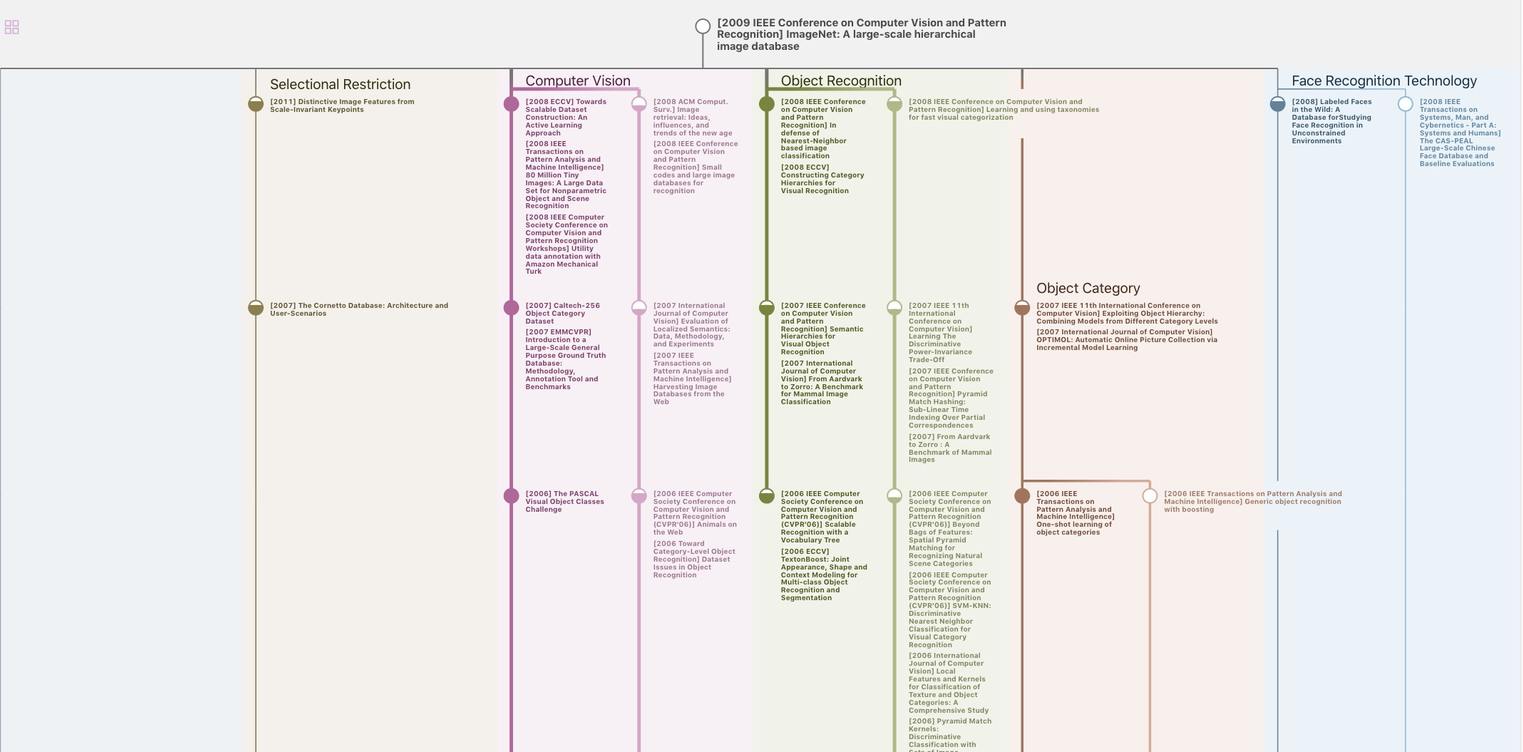
生成溯源树,研究论文发展脉络
Chat Paper
正在生成论文摘要