Deep Quasi-Periodic Priors: Signal Separation in Wearable Systems with Limited Data.
Design, Automation, and Test in Europe(2024)
Abstract
Quasi-periodic signal separation poses a significant challenge in wearable systems with limited data, particularly when the measured signal, influenced by multiple physiological sources, is under-represented. Addressing this issue, we introduce Deep Quasi-Periodic Priors (DQPP), a signal separation method for non-stationary, single-detector, quasi-periodic signals using an isolated input data. This approach incorporates masking and in-painting of the time-frequency spectrogram, while integrating prior harmonic and temporal patterns within the deep neural network structure. Moreover, a pattern alignment unit transforms the input signal's time-frequency patterns to closely align with the deep harmonic neural structure. The efficacy of DQPP is demonstrated in non-invasive fetal oxygen monitoring, using both synthetic and in vivo data, underscoring its applicability and potential in wearable technology.
MoreTranslated text
Key words
Signal Separation,Wearable Systems,Deep Prior Learning,Quasi-Periodic
AI Read Science
Must-Reading Tree
Example
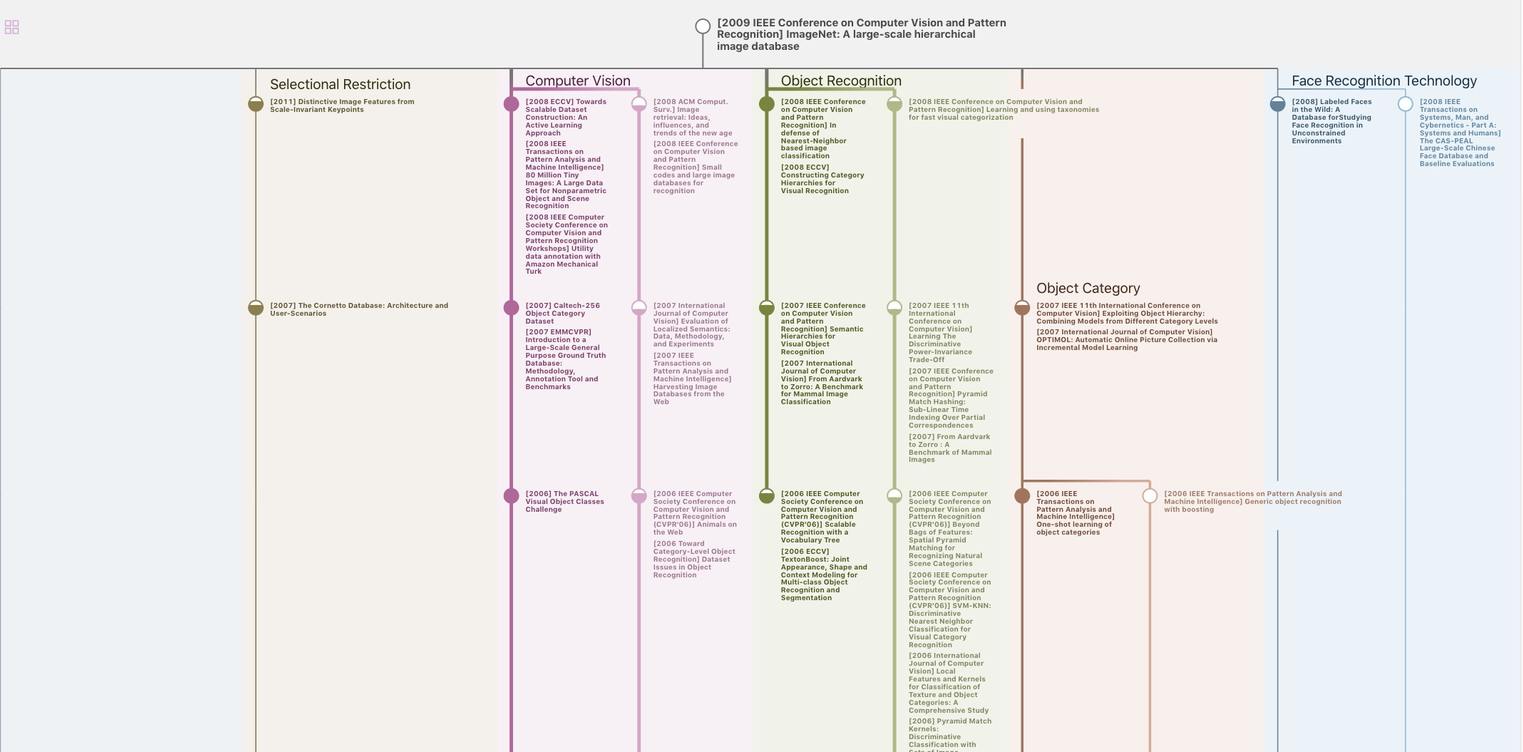
Generate MRT to find the research sequence of this paper
Chat Paper
Summary is being generated by the instructions you defined