CRONuS: Circuit Rapid Optimization with Neural Simulator.
Design, Automation, and Test in Europe(2024)
摘要
Automation of analog circuit design is highly desirable, yet challenging. Various approaches such as deep reinforcement learning (DRL), genetic algorithms, and Bayesian optimization have been proposed and found to be effective. However, these techniques require a large number of interactions with a real simulator, leading to high computational costs. Therefore, we present a novel DRL method, CRONuS, for automatic analog circuit design that uses a surrogate for the simulator. With the help of the surrogate, our method is capable of augmenting a data set with a conservative reward design for stable policy training, without having to interact with the simulator. Regardless of the type of analog circuit, our experiment demonstrated a more than 5 × improvement in sample efficiency with varying target performance metrics.
更多查看译文
关键词
Deep Reinforcement Learning,DRL,Model-based DRL,MbDRL,Transistor sizing,Sample Efficiency,Robust Circuit Design
AI 理解论文
溯源树
样例
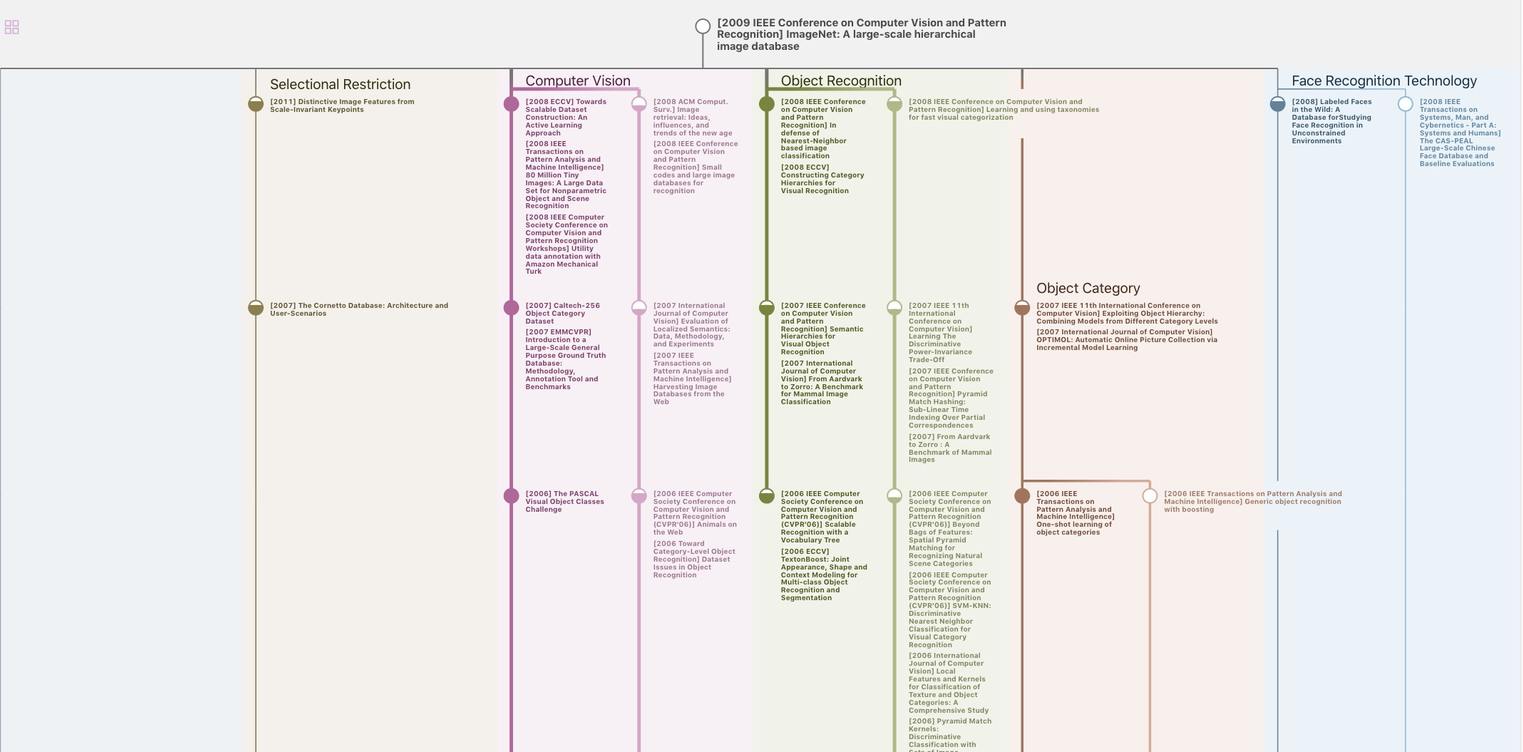
生成溯源树,研究论文发展脉络
Chat Paper
正在生成论文摘要