FVLLMONTI: The 3D Neural Network Compute Cube $(N^{2}C^{2})$ Concept for Efficient Transformer Architectures Towards Speech-to-Speech Translation
2024 Design, Automation & Test in Europe Conference & Exhibition (DATE)(2024)
摘要
This multi-partner-project contribution introduces the midway results of the Horizon 2020 FVLLMONTI project. In this project we develop a new and ultra-efficient class of ANN accelerators, the neural network compute cube
$(N^{2}C^{2})$
, which is specifically designed to execute complex machine learning tasks in a 3D technology, in order to provide the high computing power and ultra-high efficiency needed for future edgeAI applications. We showcase its effectiveness by targeting the challenging class of Transformer ANNs, tailored for Automatic Speech Recognition and Machine Translation, the two fundamental components of speech-to-speech translation. To gain the full benefit of the accelerator design, we develop disruptive vertical transistor technologies and execute design-technology-co-optimization (DTCO) loops from single device, to cell and compute cube level. Further, a hardware-software-co-optimization is executed, e.g. by compressing the executed speech recognition and translation models for energy efficient executing without substantial loss in precision.
更多查看译文
关键词
ANN,translation,DTCO,emerging technologies
AI 理解论文
溯源树
样例
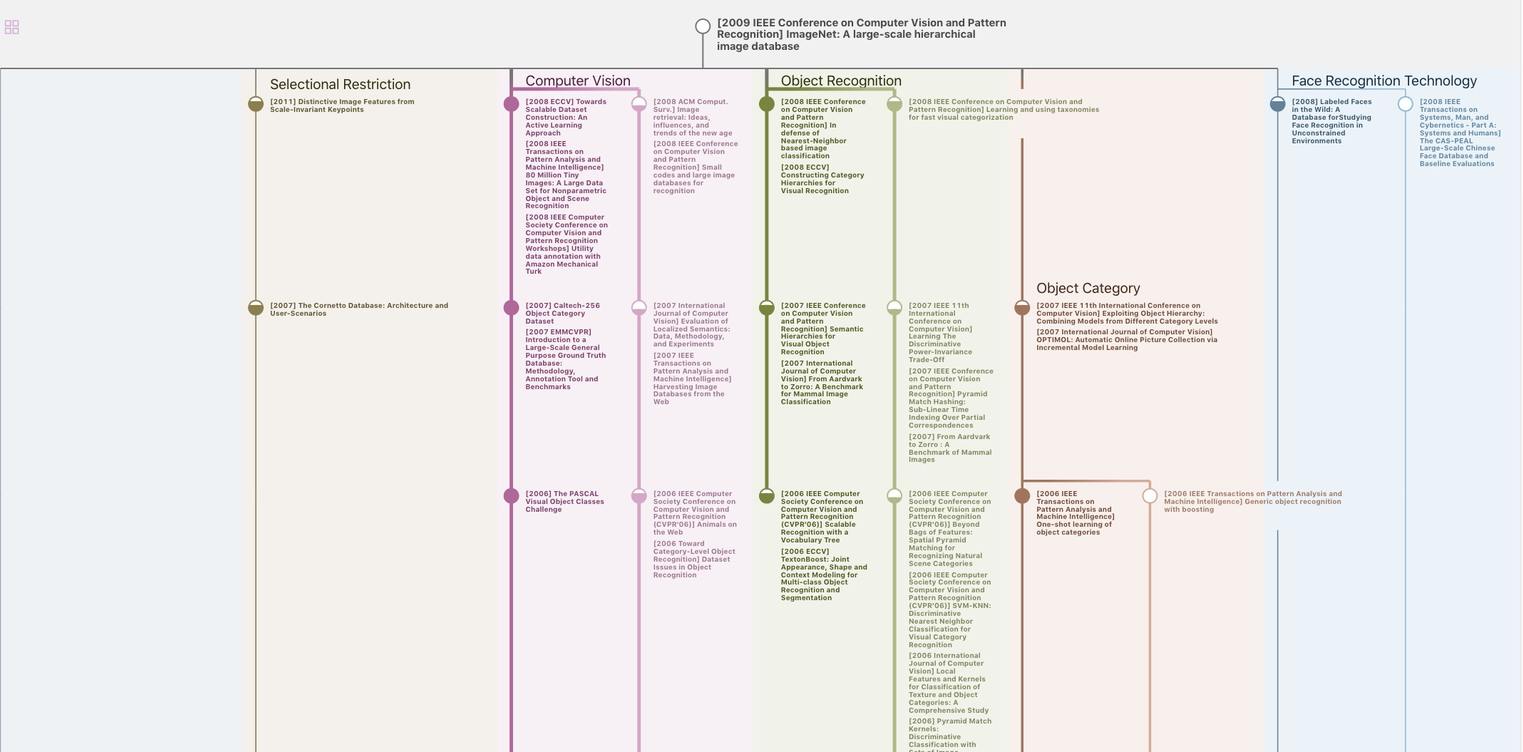
生成溯源树,研究论文发展脉络
Chat Paper
正在生成论文摘要