Ensemble of Graph Neural Networks for Enhanced Financial Fraud Detection
2024 IEEE 9th International Conference for Convergence in Technology (I2CT)(2024)
Abstract
In the growing financial services industry, fraud prevention is crucial to ensure a secure user and provider environment. For prediction, traditional fraud detection depends on rule-based techniques or manually created features. However, the information in such financial transactions between users is diverse and the relationships between users are complex. Therefore, numerous heterogeneous Graph Neural Networks (GNNs) are employed to capture complicated correlations and patterns and efficiently transform unlabeled data into labelled ones. We propose a stacking ensemble of four different Graph Neural Network (GNN) models for fraud detection: Semi Graph Neural Networks (Semi-GNN), Graph Embeddings for Malicious Accounts (GEM), Graph based Anti-Spam (GAS) and FdGars, where each model specialises in a different way of dealing with different data. At the same time, an attention mechanism is incorporated to make the model interpretable and explain why users are classified as fraudulent. Experiments show that our approach consistently outperforms baseline models over time. The use of an ensemble increases the predictive accuracy of the models and shows encouraging results that demonstrate the efficiency of the proposed method.
MoreTranslated text
Key words
Graph Neural Network(GNN),Ensemble,Semi-GNN,GEM,GAS,FdGars,Financial Fraud
AI Read Science
Must-Reading Tree
Example
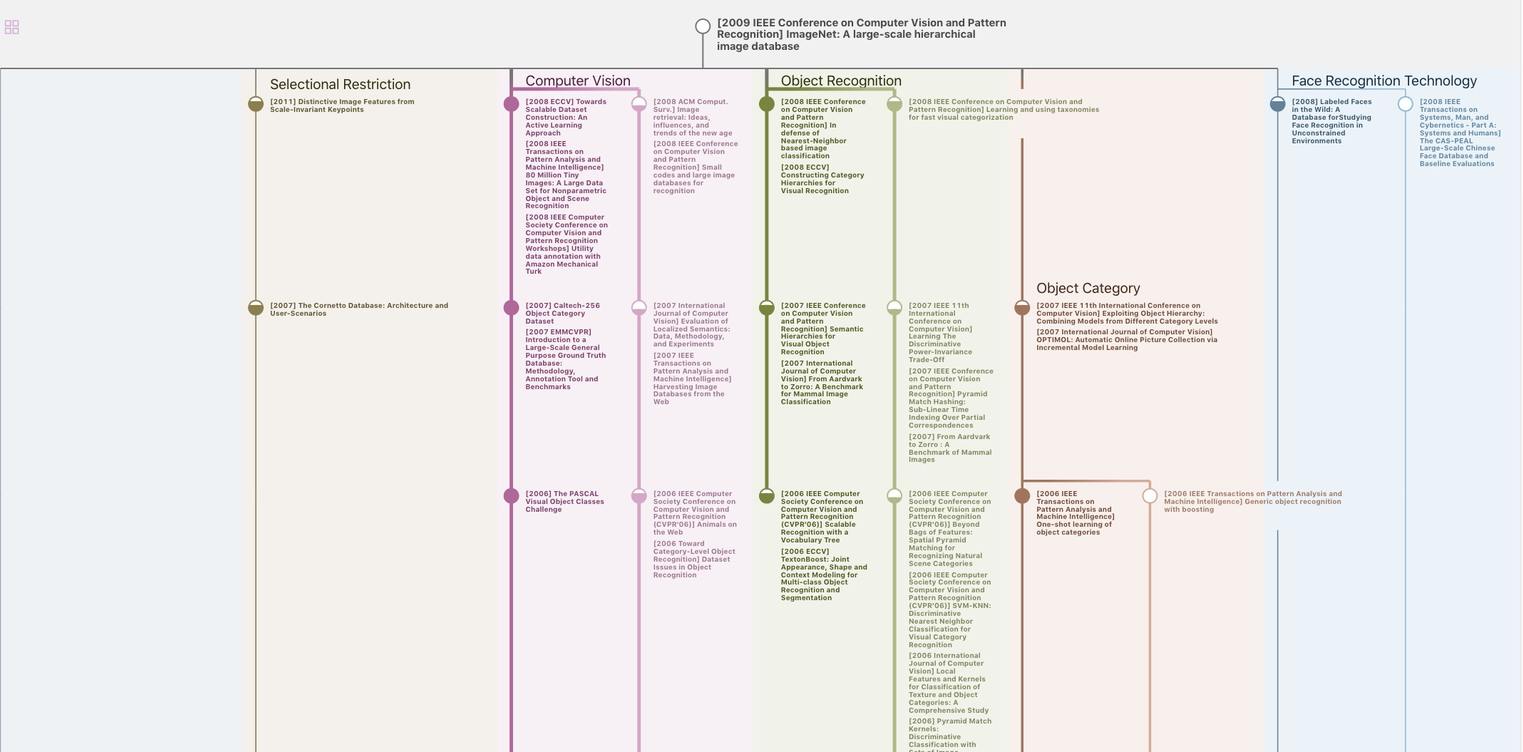
Generate MRT to find the research sequence of this paper
Chat Paper
Summary is being generated by the instructions you defined