Interference-based and Deep-Learning-Aided Physical Layer Security Enhancement in Uplink NOMA under Cooperative Eavesdropping
IEEE Transactions on Vehicular Technology(2024)
Abstract
Physical Layer Security (PLS) for Uplink NonOrthogonal Multiple Access (UL-NOMA) is investigated, under cooperative multi-eavesdropping. Particular attention is paid to the effect that Successive Interference Cancellation (SIC) decoding order has over the system secrecy rate. Each NOMA user is subject to an individual minimum bit rate requirement, whereas multiple eavesdroppers cooperate among themselves to enhance their repercussion on the system. We demonstrate the impact SIC decoding order has on the secrecy performance, and the importance to look for an optimal solution to the decoding order selection problem. We show how this problem is indeed a very challenging combinatorial one with a factorial complexity related to the number of users and eavesdroppers involved in the system. Given the high computational power demand to find the optimal solution, we approach the challenge in two ways. First, we propose a near-optimal interference-based analytical approach to find a secure decoding order. The idea is to take advantage of the inherent NOMA characteristic of self-interfere other legitimate users, to also act as an interference source over the eavesdroppers. Secondly, we introduce deep learning as another option to achieve a near-optimal solution with a much lower complexity. We see how a deep neural network is able to improve the performance of the interference-based analytical solution, being robuster to users' and eavesdroppers' increment. Finally, we demonstrate the accuracy of the two proposed solutions through numerical simulations and highlight their importance and advantages.
MoreTranslated text
Key words
Non-Orthogonal Multiple Access,NOMA,Security,Secrecy Rate,Eavesdropping,Deep Learning,Physical Layer Security
AI Read Science
Must-Reading Tree
Example
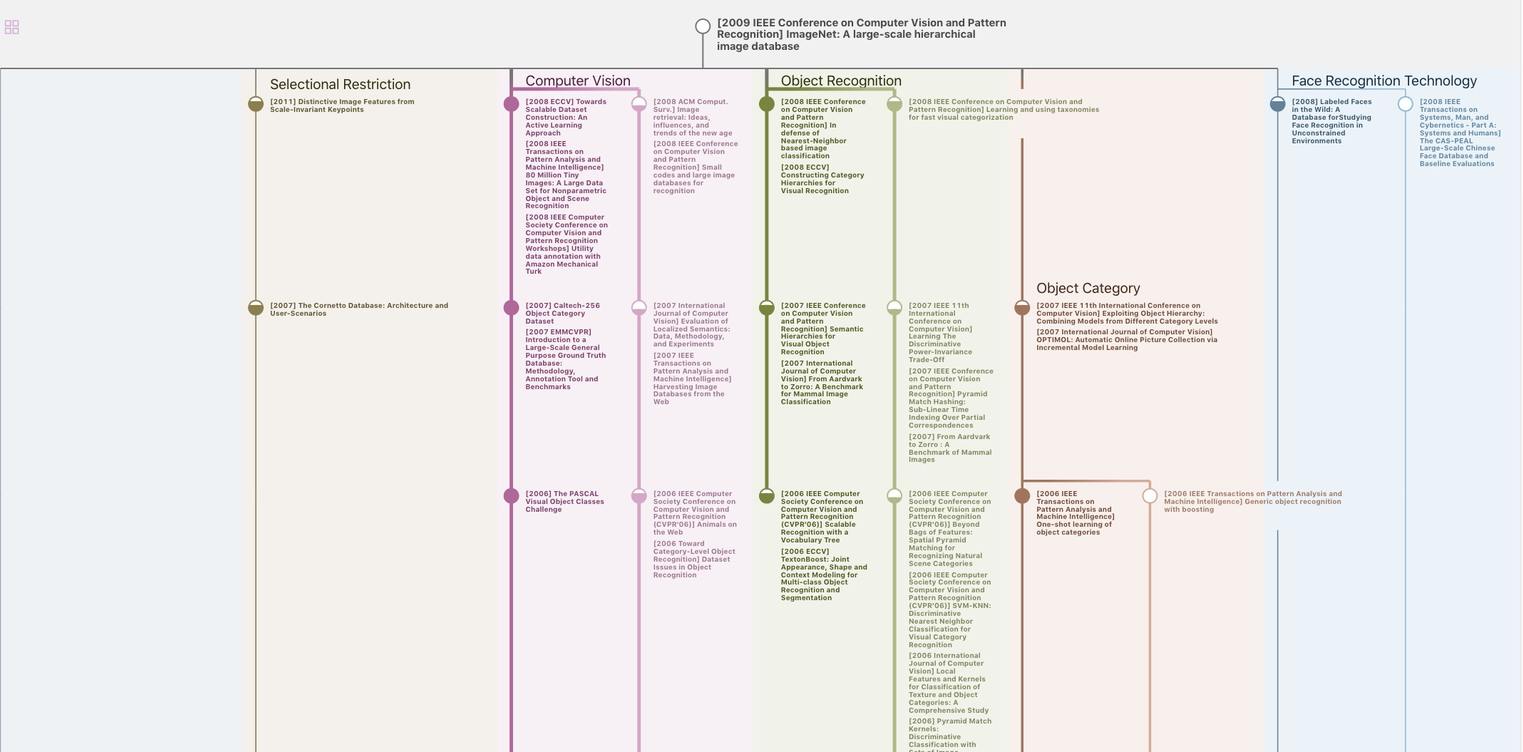
Generate MRT to find the research sequence of this paper
Chat Paper
Summary is being generated by the instructions you defined