High Spatial Resolution and Accurate Temperature Profile Measurements in a Nuclear Reactor Core Enabled by Machine Learning
IEEE Sensors Journal(2024)
摘要
This paper reports on laser fabrication, in-pile testing, and artificial intelligence (AI) enabled data analytics of distributed fiber sensors to monitor nuclear power systems (NPS). As intense radiation, high temperature, and hysteresis induce complex and significant sensor drifts, this paper studies long short-term memory (LSTM) neural network and k-nearest neighbor algorithm (k-NN) as data analytics tools to correct sensor drift. Using three thermocouples (TC) as calibration, LSTM neural networks were used to learn radiation effects exerted on fiber sensors located at the same location of TCs during normal states and simulated incidents of a test reactor. Fiber sensor measurements of other locations were rectified using k-NN based on knowledge harnessed by fiber sensors in calibration locations. The integrated sensor fusion and AI-enabled data analytics improved the temperature accuracy of fiber sensors to 2 °C during normal conditions. In a simulated reactor anomaly with a temperature excursion to 520 °C, the fiber sensors’ measurement accuracy was within 18 °C. This paper presents an integrated approach to fully harness the 1-cm-high spatial resolution measurement capabilities of distributed fiber sensors for nuclear energy applications. It can improve the applicability of fiber sensors as part of Instrumentation and Control (I&C) systems for future NPS.
更多查看译文
关键词
High-temperature,machine learning,neutron radiation effects,optical fiber sensors,predictive models,radiation monitoring
AI 理解论文
溯源树
样例
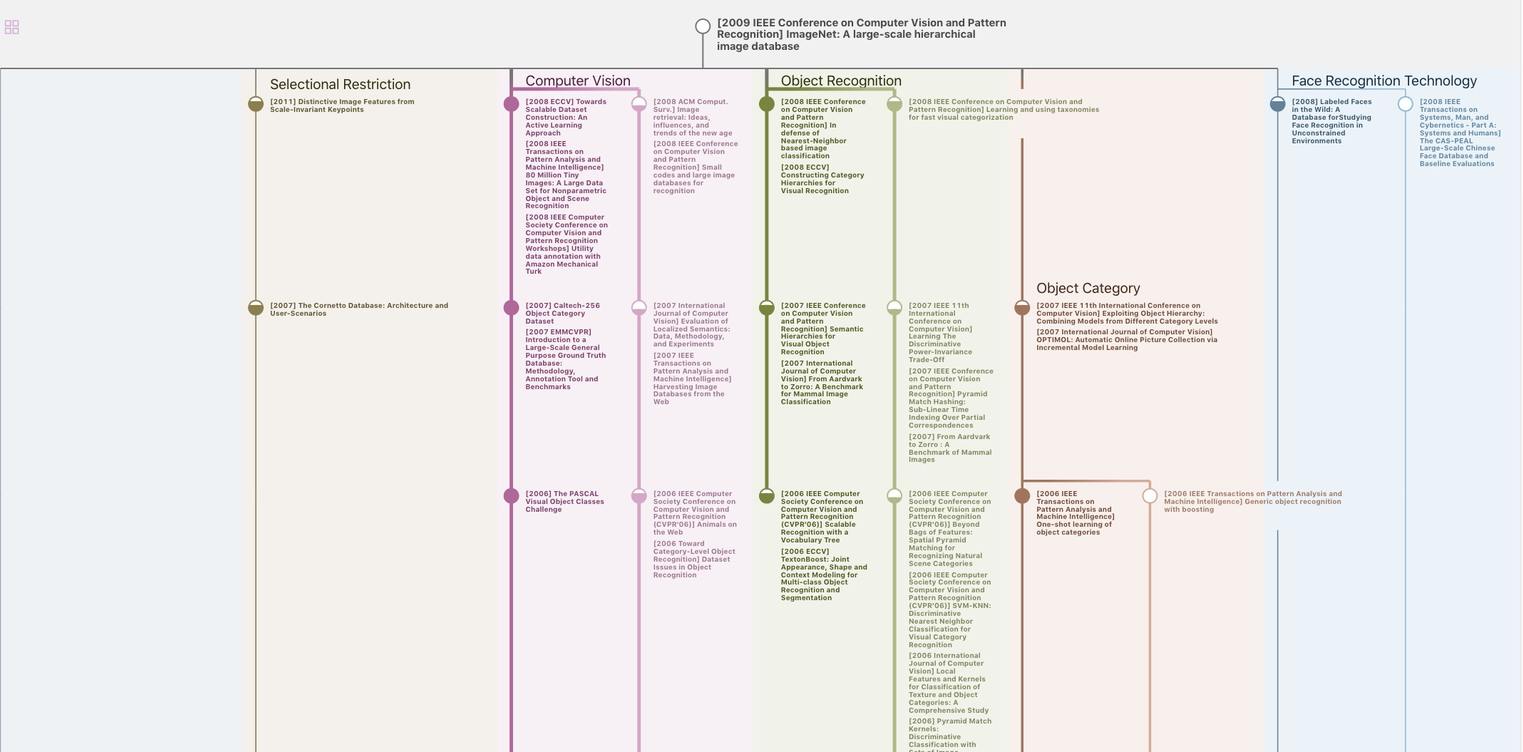
生成溯源树,研究论文发展脉络
Chat Paper
正在生成论文摘要