ScalableTrack: Scalable One-Stream Tracking via Alternating Learning
IEEE TRANSACTIONS ON NEURAL NETWORKS AND LEARNING SYSTEMS(2024)
摘要
Transformer-based one-stream trackers are widely used to extract features and interact information for visual object tracking. However, the current one-stream tracker has fixed computational dimensions between different stages, which limits the network's ability to learn context clues and global representations, resulting in a decrease in the ability to distinguish between targets and backgrounds. To address this issue, a new scalable one-stream tracking framework, ScalableTrack, is proposed. It unifies feature extraction and information integration by intrastage mutual guidance, leveraging the scalability of target-oriented features to enhance object sensitivity and obtain discriminative global representations. In addition, we bridge interstage contextual cues by introducing an alternating learning strategy and solve the arrangement problem of the two modules. The alternating learning strategy uses alternate stacks of feature extraction and information interaction to focus on tracked objects and prevent catastrophic forgetting of target information between different stages. Experiments on eight challenging benchmarks (TrackingNet, GOT-10k, VOT2020, UAV123, LaSOT, LaSOText , OTB100, and TC128) show that ScalableTrack outperforms state-of-the-art (SOTA) methods with better generalization and global representation ability.
更多查看译文
关键词
Target tracking,Feature extraction,Transformers,Visualization,Task analysis,Sensitivity,Learning systems,Alternating learning,interstage mechanism,intrastage mechanism,scalable feature,visual tracking
AI 理解论文
溯源树
样例
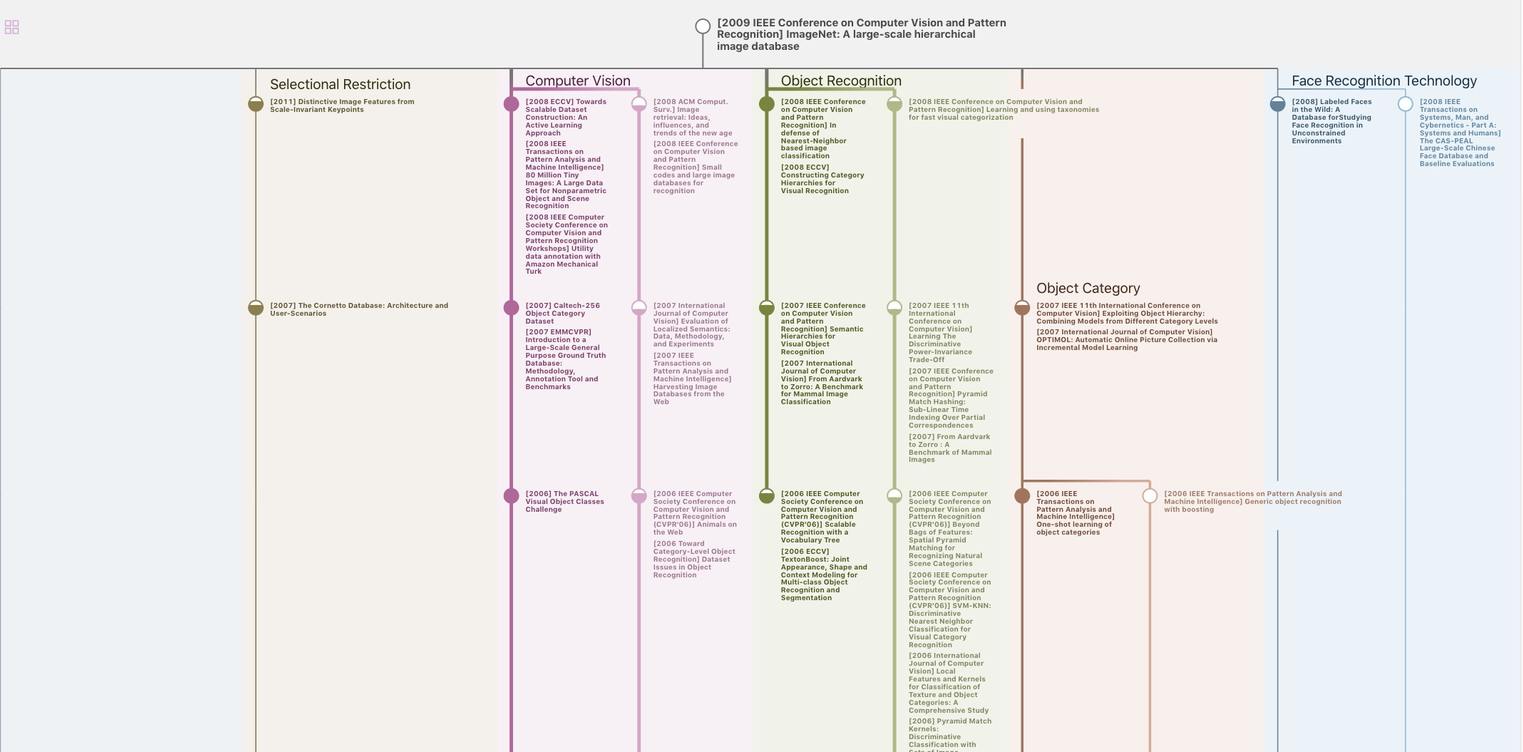
生成溯源树,研究论文发展脉络
Chat Paper
正在生成论文摘要