Time-frequency-space EEG decoding model based on dense graph convolutional network for stroke.
IEEE journal of biomedical and health informatics(2024)
摘要
Stroke, a sudden cerebrovascular ailment resulting from brain tissue damage, has prompted the use of motor imagery (MI)-based Brain-Computer Interface (BCI) systems in stroke rehabilitation. However, analyzing EEG signals from stroke patients is challenging because of their low signal-to-noise ratio and high variability. Therefore, we propose a novel approach that combines the modified S-transform (MST) and a dense graph convolutional network (DenseGCN) algorithm to enhance the MI-BCI performance across time, frequency, and space domains. MST is a time-frequency analysis method that efficiently concentrates energy in EEG signals, while DenseGCN is a deep learning model that uses EEG feature maps from each layer as inputs for subsequent layers, facilitating feature reuse and hyper-parameters optimization. Our approach outperforms conventional networks, achieving a peak classification accuracy of 90.22% and an average information transfer rate (ITR) of 68.52 bits per minute. Moreover, we conduct an in-depth analysis of the event-related desynchronization/event-related synchronization (ERD/ERS) phenomenon in the deep-level EEG features of stroke patients. Our experimental results confirm the feasibility and efficacy of the proposed approach for MI-BCI rehabilitation systems.
更多查看译文
关键词
Electroencephalogram,motor imagery,brain-computer interface,dense graph convolutional network,stroke
AI 理解论文
溯源树
样例
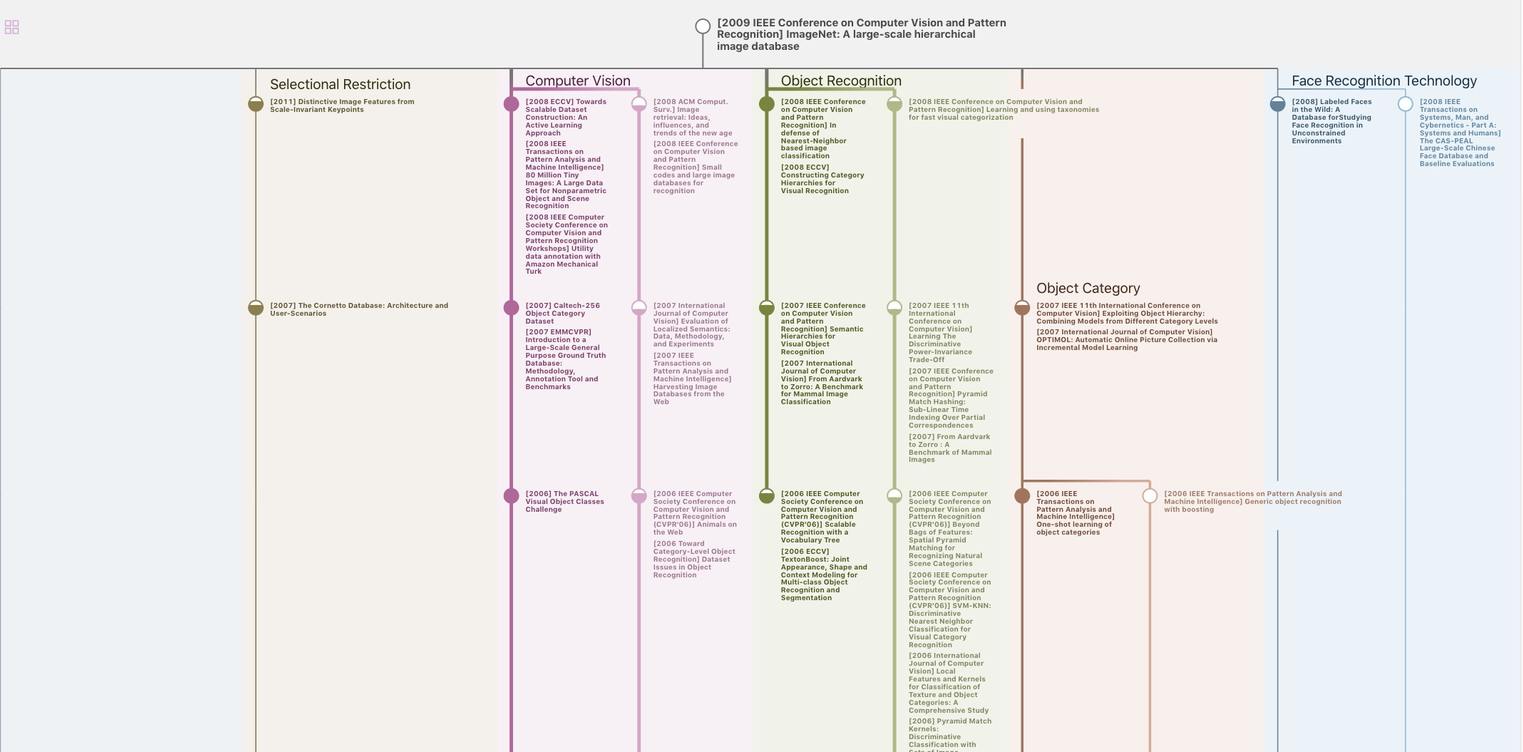
生成溯源树,研究论文发展脉络
Chat Paper
正在生成论文摘要