TCN-Inception: Temporal Convolutional Network and Inception modules for sensor-based human activity recognition
Future Generation Computer Systems(2024)
Abstract
The field of Human Activity Recognition (HAR) has experienced a significant surge in interest due to its essential role across numerous areas, including human–computer interaction (HCI), healthcare, smart homes, and various Internet of Things (IoT) applications. The power of deep learning methods in performing various classification tasks, including HAR, has been well-demonstrated. In light of this, our paper presents an efficient HAR system developed using a unique deep-learning architecture called TCN-Inception, which is designed for multivariate time series tasks like HAR data, by combining Temporal Convolutional Network (TCN) and Inception modules. The network starts with an Inception module that uses parallel convolution layers with various kernel sizes for feature extraction. It then includes a TCN module with dilated convolutions to grasp extended temporal patterns. Features are merged from different channels, and the use of residual connections and batch normalization improves training and deepens the architecture. We use four public datasets, UCI-HAR, MobiAct, Daphnet, and DSADS to assess the performance of the TCN-Inception model, and it obtains an average accuracy of 96.15%, 98.86%, 92.63%, and 99.50% for each dataset, respectively. Moreover, we compare the TCN-Inception to several deep learning frameworks to verify its performance. Finally, we implement an ablation study using several architectural configurations of the TCN-Inception model.
MoreTranslated text
Key words
Human activity recognition,Smart homes,Internet of things (IoT),Deep learning,CNN,Inception
AI Read Science
Must-Reading Tree
Example
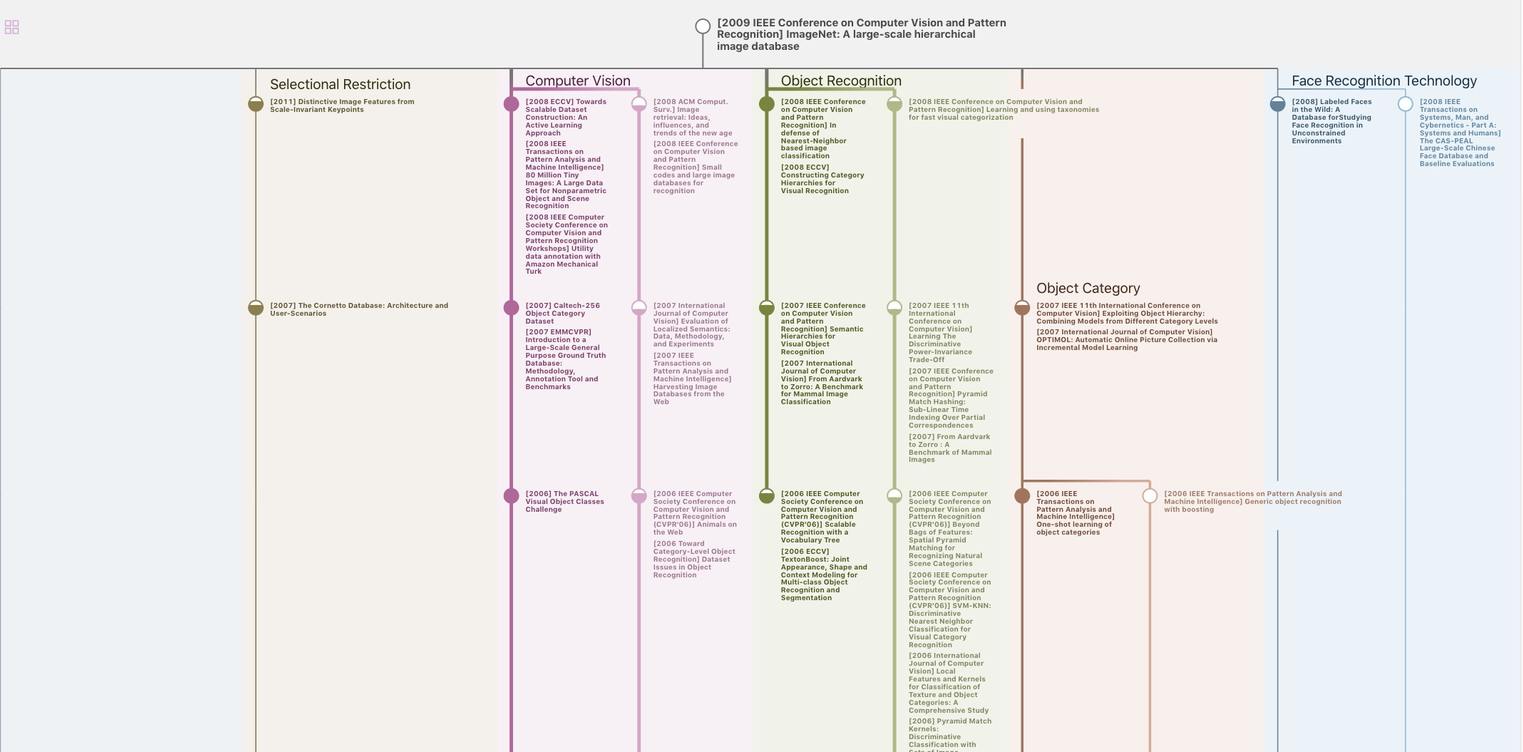
Generate MRT to find the research sequence of this paper
Chat Paper
Summary is being generated by the instructions you defined