Buffeting reliability of high-rise bridge tower in mountain area based on CNN-BiLSTM
Applied Soft Computing(2024)
Abstract
In recent years, the wind-induced vibration of structures has received a great deal of scholarly attention due to the increasing scale of large structures. In this study, an algorithm that combines a stochastic pseudo excitation method (SPEM), a convolutional neural network (CNN) and a bidirectional long short-term memory network (BiLSTM), named as SPEM-CNN-BiLSTM (SCBL), is proposed. The SCBL is a surrogate model consisting of three different computational modules, in which the SPEM can provide training samples for CNN-BiLSTM (CBL) network architecture, a convolutional layer extracts features of stochastic parameters and a BiLSTM handles time histories of bridge response. The accuracy of the SCBL prediction results is ensured by the set error threshold. The model also accounts for structural uncertainty in the inputs and is adapted during the training step to be more suitable for reliability analysis. According to the output qualified prediction data, the dynamic reliability based on the 99% threshold is calculated by obtaining its probability density function, and the wind-induced vibration responses of uncertain bridge towers are studied. Firstly, the wind environment of the bridge tower was analyzed, and the average wind speed of 20m/s among the bridge area was obtained by field measurements, and Computational Fluid Dynamics (CFD). Secondly, the proposed artificial neural network algorithm is applied to bridge jitter reliability analysis and compared with the traditionally recognized methods: the Monte Carlo method (MCM) and the stochastic pseudo excitation method (SPEM). The results of SCBL calculations are closer to the results of MCM than those of other methods. Additionally, the computational efficiency of the method is improved by 40 times over MCM and 2 times over SPEM. Finally, the data generated by the SCBL method will be used for reliability analysis. Uncertainties in structure, wind speed, and yaw angle all contribute to the uncertainty and dispersion of the stochastic response prediction. Among them, the uncertainty in wind speed has the greatest impact on the reliability.
MoreTranslated text
Key words
Structural reliability,Deep neural networks,Uncertain,stochastic pseudo excitation method,Buffeting
AI Read Science
Must-Reading Tree
Example
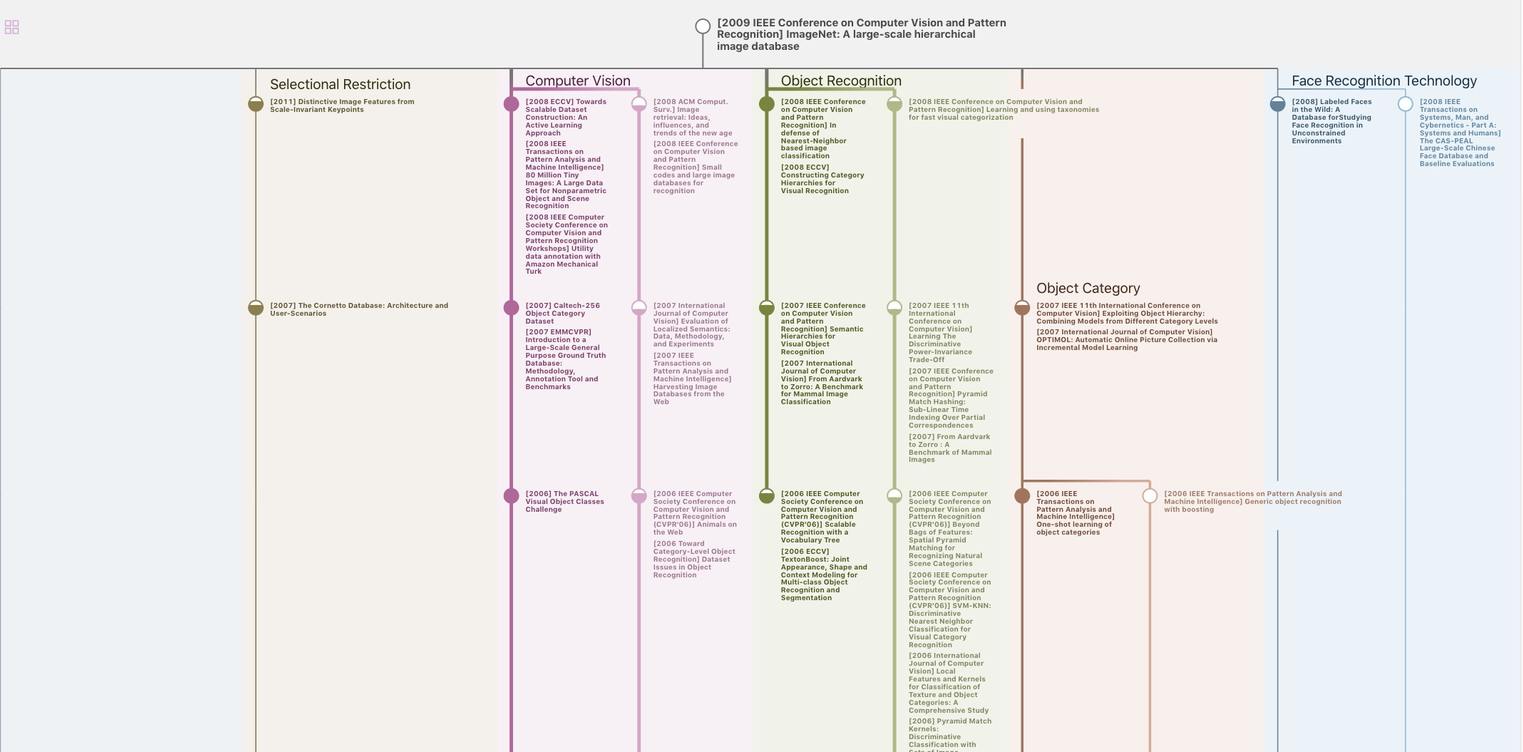
Generate MRT to find the research sequence of this paper
Chat Paper
Summary is being generated by the instructions you defined