Annotation alignment: Comparing LLM and human annotations of conversational safety
CoRR(2024)
摘要
To what extent to do LLMs align with human perceptions of safety? We study
this question via *annotation alignment*, the extent to which LLMs and humans
agree when annotating the safety of user-chatbot conversations. We leverage the
recent DICES dataset (Aroyo et al., 2023), in which 350 conversations are each
rated for safety by 112 annotators spanning 10 race-gender groups. GPT-4
achieves a Pearson correlation of r = 0.59 with the average annotator rating,
higher than the median annotator's correlation with the average (r=0.51). We
show that larger datasets are needed to resolve whether GPT-4 exhibits
disparities in how well it correlates with demographic groups. Also, there is
substantial idiosyncratic variation in correlation *within* groups, suggesting
that race gender do not fully capture differences in alignment. Finally, we
find that GPT-4 cannot predict when one demographic group finds a conversation
more unsafe than another.
更多查看译文
AI 理解论文
溯源树
样例
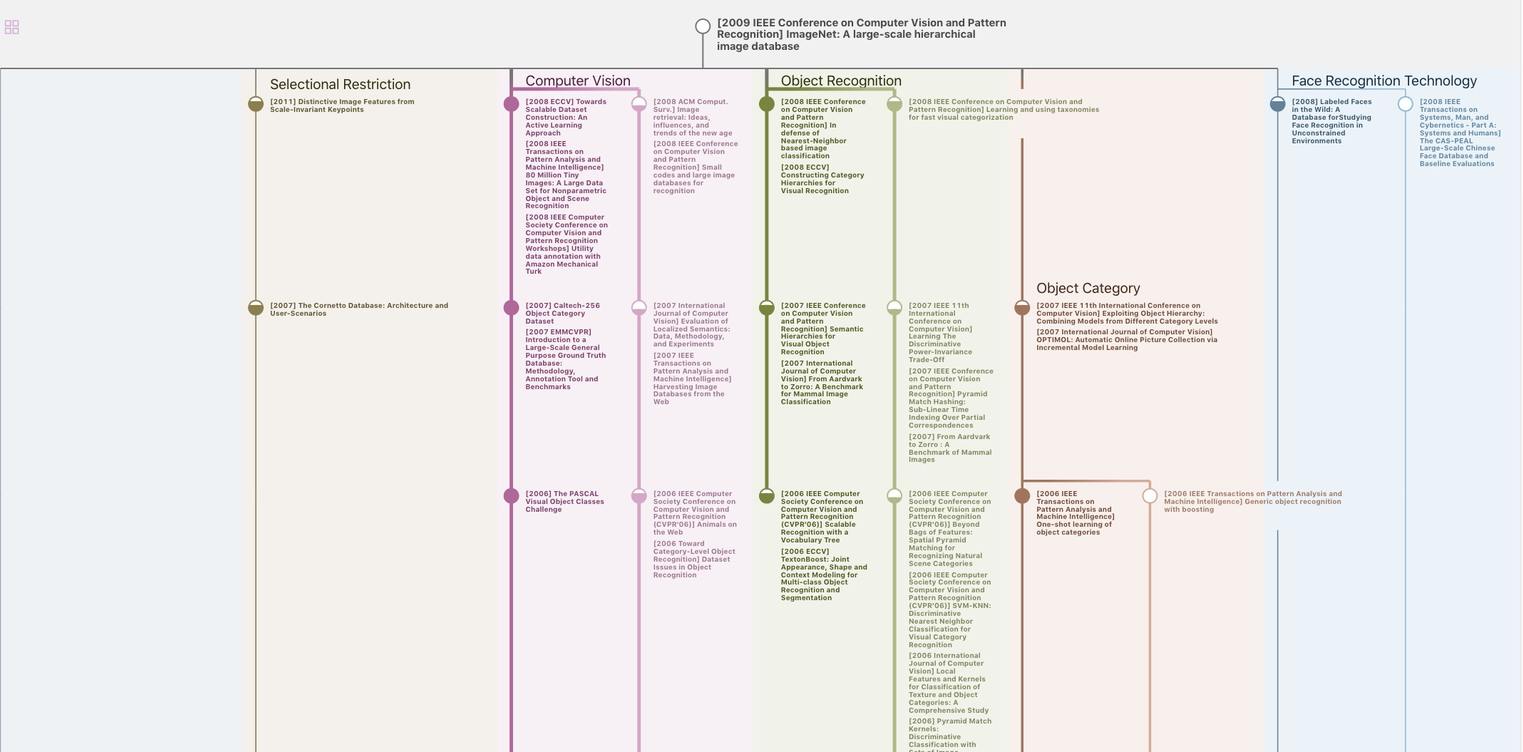
生成溯源树,研究论文发展脉络
Chat Paper
正在生成论文摘要