Model Updating for Nonlinear Systems with Stability Guarantees
CoRR(2024)
摘要
To improve the predictive capacity of system models in the input-output
sense, this paper presents a framework for model updating via learning of
modeling uncertainties in locally (and thus also in globally) Lipschitz
nonlinear systems. First, we introduce a method to extend an existing known
model with an uncertainty model so that stability of the extended model is
guaranteed in the sense of set invariance and input-to-state stability. To
achieve this, we provide two tractable semi-definite programs. These programs
allow obtaining optimal uncertainty model parameters for both locally and
globally Lipschitz nonlinear models, given uncertainty and state trajectories.
Subsequently, in order to extract this data from the available input-output
trajectories, we introduce a filter that incorporates an approximated internal
model of the uncertainty and asymptotically estimates uncertainty and state
realizations. This filter is also synthesized using semi-definite programs with
guaranteed robustness with respect to uncertainty model mismatches,
disturbances, and noise. Numerical simulations for a large data-set of a roll
plane model of a vehicle illustrate the effectiveness and practicality of the
proposed methodology in improving model accuracy, while guaranteeing stability.
更多查看译文
AI 理解论文
溯源树
样例
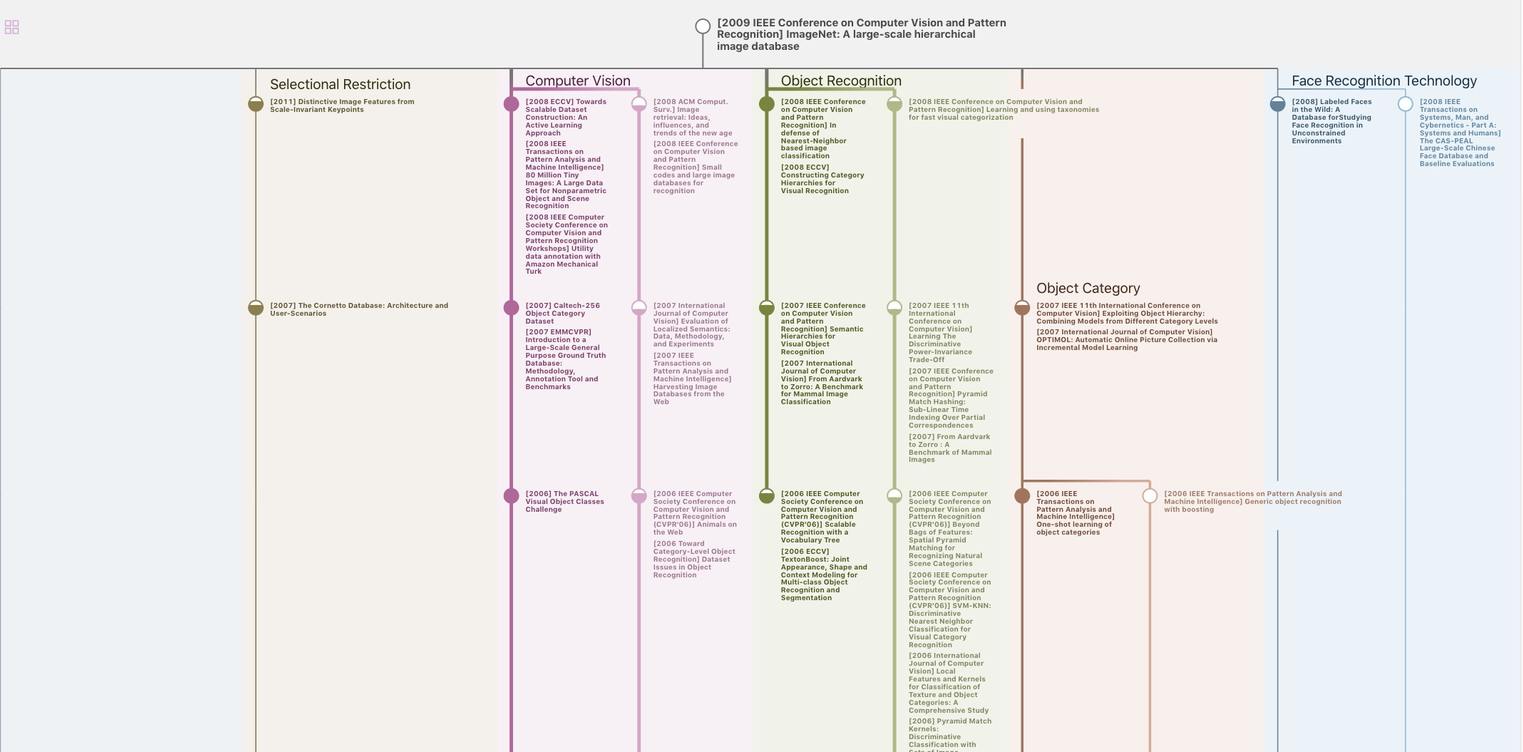
生成溯源树,研究论文发展脉络
Chat Paper
正在生成论文摘要