Risk-Aware Framework Development for Disruption Prediction: Alcator C-Mod and DIII-D Survival Analysis
Journal of Fusion Energy(2024)
Abstract
Survival regression models can achieve longer warning times at similar receiver operating characteristic performance than previously investigated models. Survival regression models are also shown to predict the time until a disruption will occur with lower error than other predictors. Time-to-event predictions from time-series data can be obtained with a survival analysis statistical framework, and there have been many tools developed for this task which we aim to apply to disruption prediction. Using the open-source Auton-Survival package we have implemented disruption predictors with the survival regression models Cox Proportional Hazards, Deep Cox Proportional Hazards, and Deep Survival Machines. To compare with previous work, we also include predictors using a Random Forest binary classifier, and a conditional Kaplan-Meier formalism. We benchmarked the performance of these five predictors using experimental data from the Alcator C-Mod and DIII-D tokamaks by simulating alarms on each individual shot. We find that developing machine-relevant metrics to evaluate models is an important area for future work. While this study finds cases where disruptive conditions are not predicted, there are instances where the desired outcome is produced. Giving the plasma control system the expected time-to-disruption will allow it to determine the optimal actuator response in real time to minimize risk of damage to the device.
MoreTranslated text
Key words
Tokamak plasma,Disruption prediction,Machine learning,Survival analysis
AI Read Science
Must-Reading Tree
Example
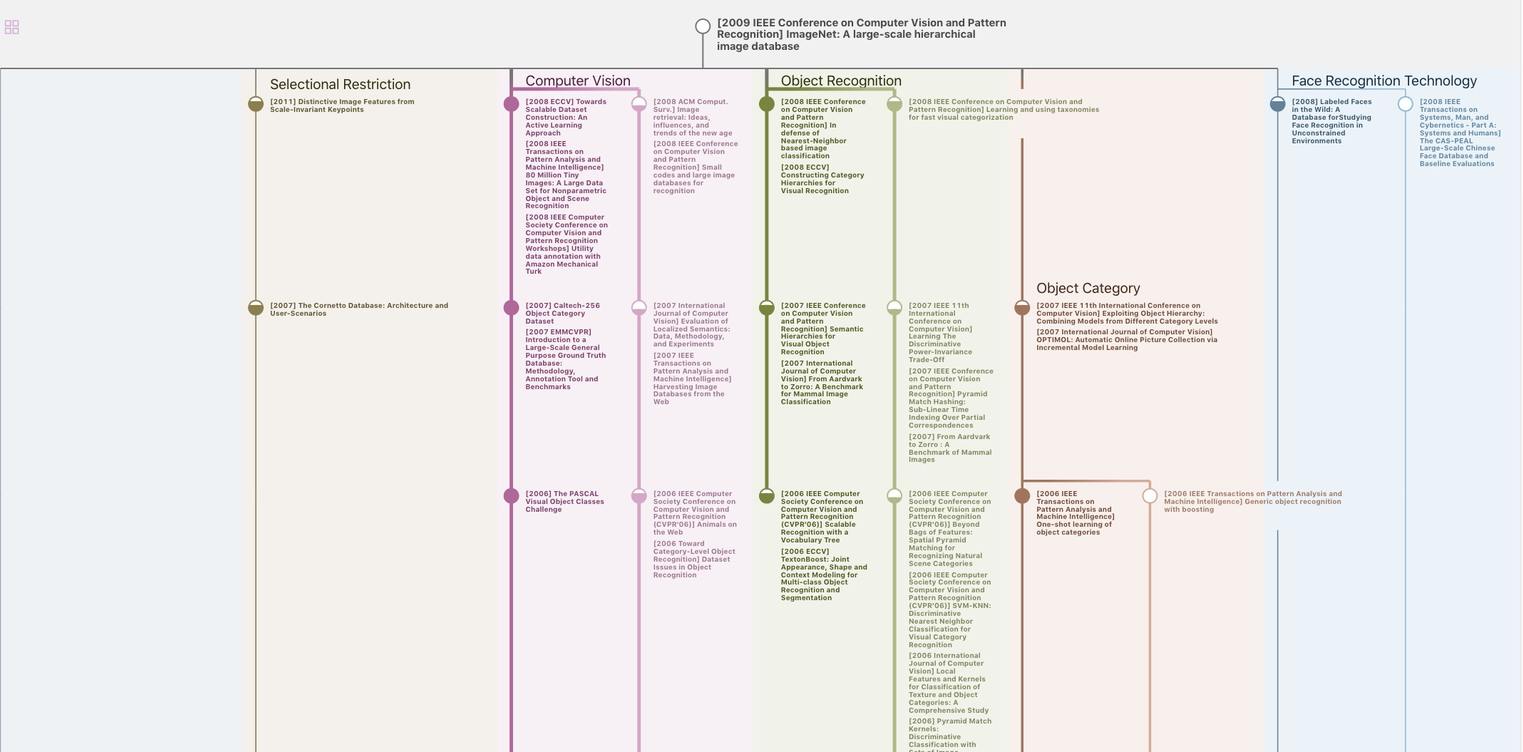
Generate MRT to find the research sequence of this paper
Chat Paper
Summary is being generated by the instructions you defined