Enhancement and optimisation of human pose estimation with multi-scale spatial attention and adversarial data augmentation
Information Fusion(2024)
Abstract
Human pose estimation, a vital pursuit in the realm of computer vision, aims to predict the spatial coordinates of key points within images. Despite the advancements achieved by employing a Convolution Neural Network (CNN), this task still faces considerable challenges, especially in handling occlusion and overfitting issues. This paper introduces a new human pose estimation network designed to address the challenges posed by occluded and blurred images. It features a multi-scale spatial attention mechanism that zeroes in on the human body, significantly improving feature extraction for complex images. Moreover, this versatile attention module is compatible with a wide range of convolutional neural network-based pose estimation frameworks, unlike other mechanisms restricted to particular networks. Addressing the overfitting issue in human pose estimation models, this paper introduces an adversarial network-based data augmentation technique. A generator specifically tailored for pose estimation is adversarially trained to produce optimal augmentation samples, thereby reducing model overfitting. Experimental validation confirms that this augmentation method notably enhances the prediction accuracy of the pose estimation model without incurring extra computational costs. In addition, this paper introduces a streamlined Feature Pyramid Network (FPN) that enables shallow networks to assimilate extensive-scale data, addressing the issue of excessive model size. The experimental validation on the benchmark datasets MPII and MSCOCO demonstrates the efficacy of this integrated approach, showcasing significant improvements in the accuracy and the overall performance of human pose estimation and surpassing the existing methodologies. This approach effectively enhances the performance of the baseline model, achieving the best accuracy of 92.2% and 80.4% on the MPII and MSCOCO, respectively.
MoreTranslated text
Key words
Human pose,Attention mechanism,Adversarial training,Pose estimation
AI Read Science
Must-Reading Tree
Example
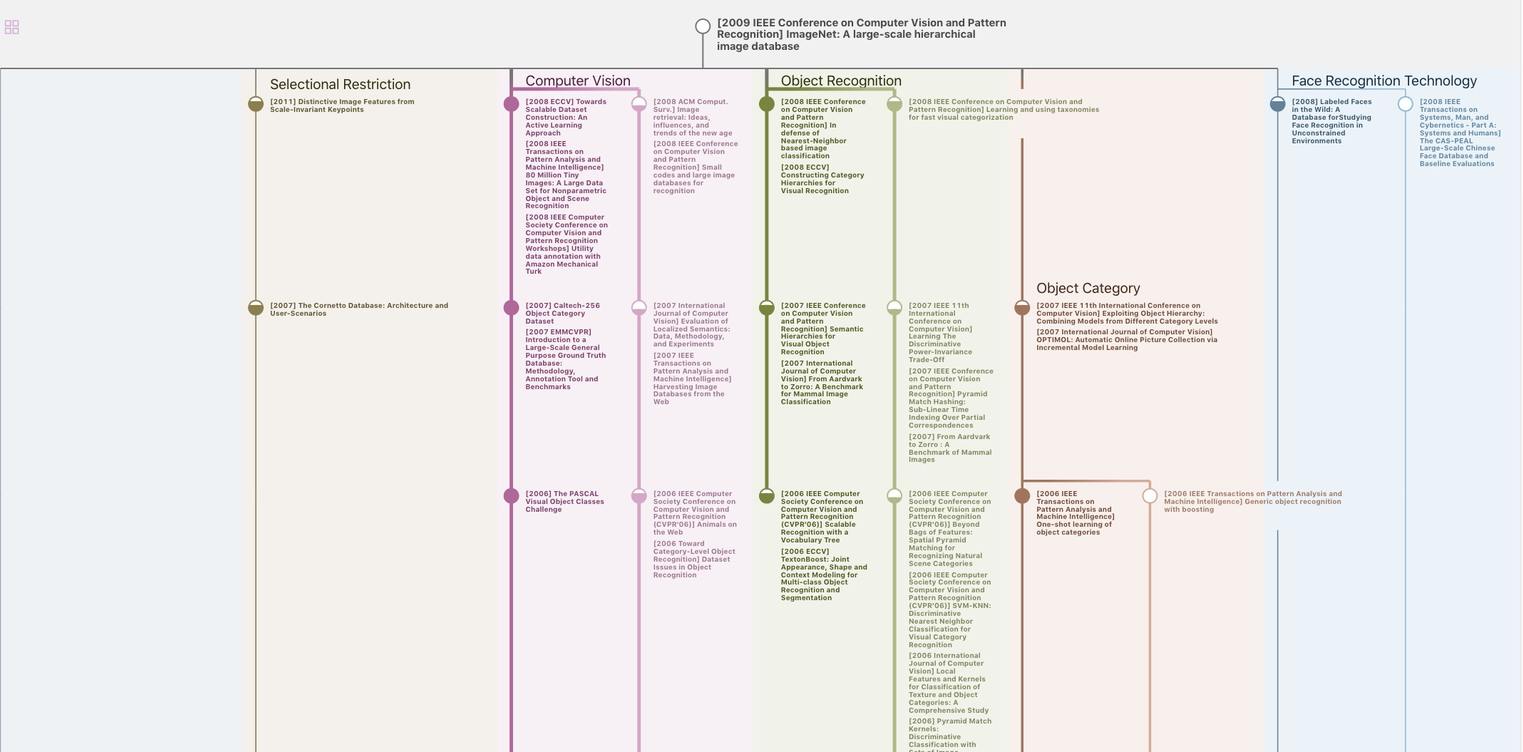
Generate MRT to find the research sequence of this paper
Chat Paper
Summary is being generated by the instructions you defined