Prototype Correlation Matching and Class-Relation Reasoning for Few-Shot Medical Image Segmentation.
IEEE transactions on medical imaging(2024)
摘要
Few-shot medical image segmentation has achieved great progress in improving accuracy and efficiency of medical analysis in the biomedical imaging field. However, most existing methods cannot explore inter-class relations among base and novel medical classes to reason unseen novel classes. Moreover, the same kind of medical class has large intra-class variations brought by diverse appearances, shapes and scales, thus causing ambiguous visual characterization to degrade generalization performance of these existing methods on unseen novel classes. To address the above challenges, in this paper, we propose a Prototype correlation Matching and Class-relation Reasoning (i.e., PMCR) model. The proposed model can effectively mitigate false pixel correlation matches caused by large intra-class variations while reasoning inter-class relations among different medical classes. Specifically, in order to address false pixel correlation match brought by large intra-class variations, we propose a prototype correlation matching module to mine representative prototypes that can characterize diverse visual information of different appearances well. We aim to explore prototypelevel rather than pixel-level correlation matching between support and query features via optimal transport algorithm to tackle false matches caused by intra-class variations. Meanwhile, in order to explore inter-class relations, we design a class-relation reasoning module to segment unseen novel medical objects via reasoning inter-class relations between base and novel classes. Such inter-class relations can be well propagated to semantic encoding of local query features to improve few-shot segmentation performance. Quantitative comparisons illustrates the large performance improvement of our model over other baseline methods.
更多查看译文
关键词
Medical image segmentation,few-shot segmentation,intra-class variations,inter-class relations
AI 理解论文
溯源树
样例
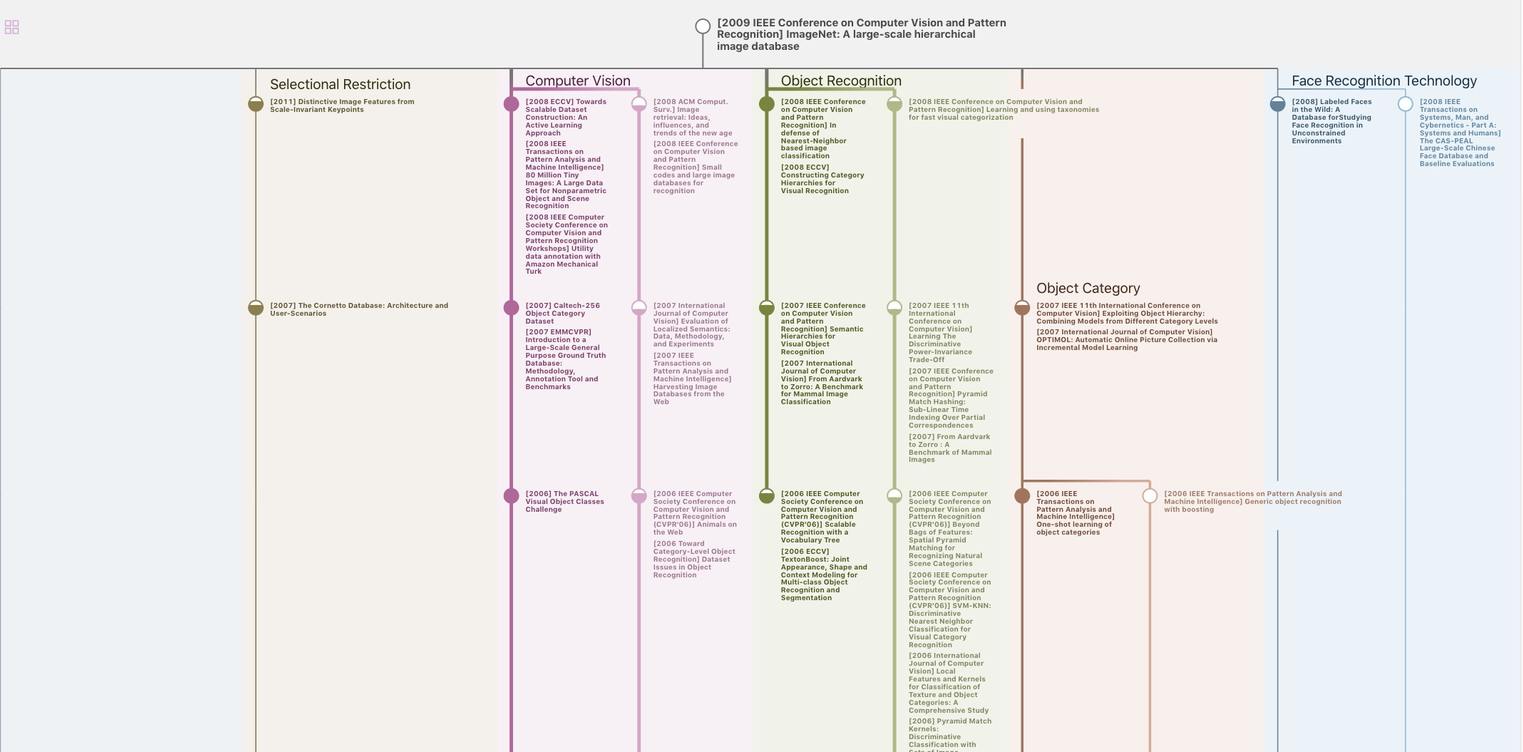
生成溯源树,研究论文发展脉络
Chat Paper
正在生成论文摘要