Column generation for multistage stochastic mixed-integer nonlinear programs with discrete state variables
arxiv(2024)
摘要
Stochastic programming provides a natural framework for modeling sequential
optimization problems under uncertainty; however, the efficient solution of
large-scale multistage stochastic programs remains a challenge, especially in
the presence of discrete decisions and nonlinearities. In this work, we
consider multistage stochastic mixed-integer nonlinear programs (MINLPs) with
discrete state variables, which exhibit a decomposable structure that allows
its solution using a column generation approach. Following a Dantzig-Wolfe
reformulation, we apply column generation such that each pricing subproblem is
an MINLP of much smaller size, making it more amenable to global MINLP solvers.
We further propose a method for generating additional columns that satisfy the
nonanticipativity constraints, leading to significantly improved convergence
and optimal or near-optimal solutions for many large-scale instances in a
reasonable computation time. The effectiveness of the tailored column
generation algorithm is demonstrated via computational case studies on a
multistage blending problem and a problem involving the routing of mobile
generators in a power distribution network.
更多查看译文
AI 理解论文
溯源树
样例
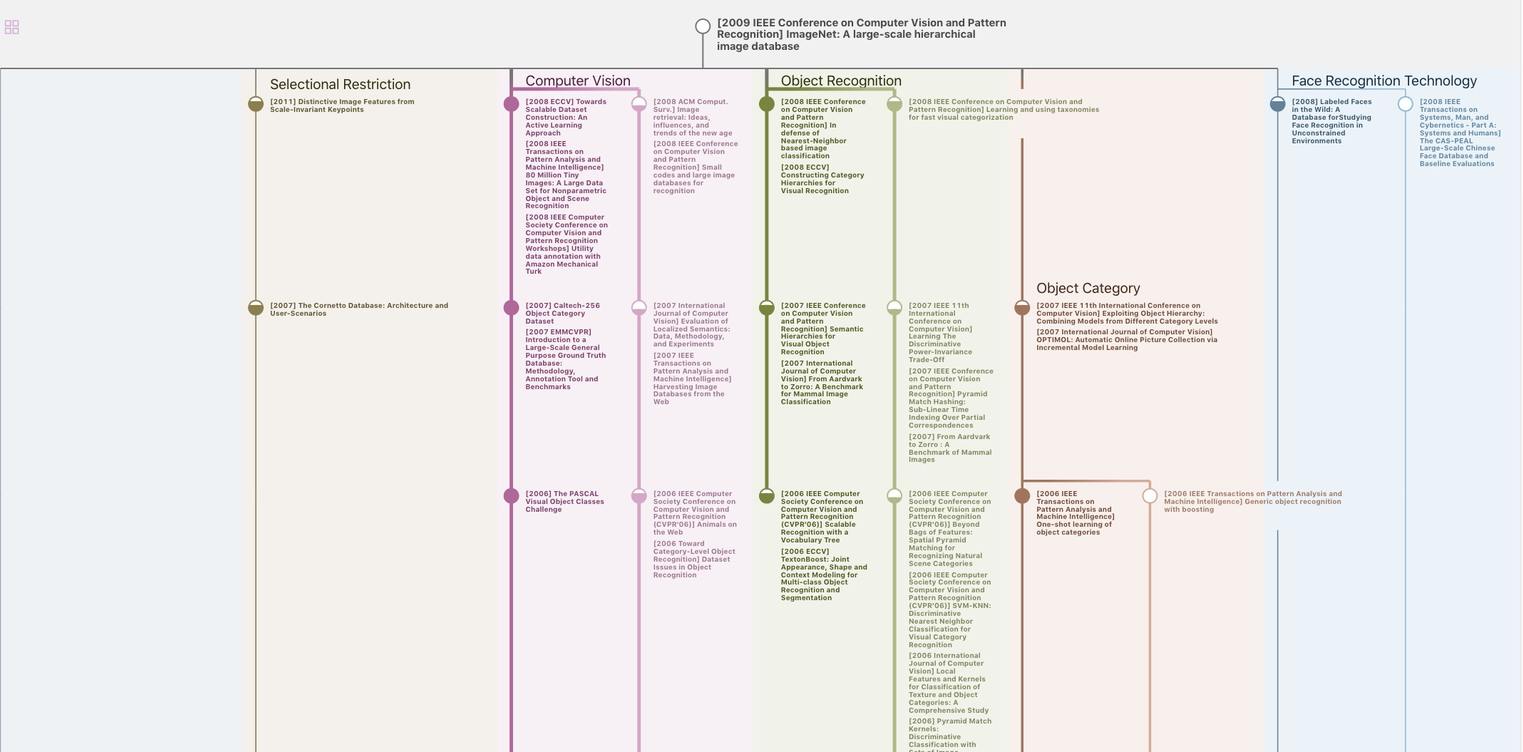
生成溯源树,研究论文发展脉络
Chat Paper
正在生成论文摘要