Unguided structure learning of DAGs for count data
arxiv(2024)
Abstract
Mainly motivated by the problem of modelling directional dependence
relationships for multivariate count data in high-dimensional settings, we
present a new algorithm, called learnDAG, for learning the structure of
directed acyclic graphs (DAGs). In particular, the proposed algorithm tackled
the problem of learning DAGs from observational data in two main steps: (i)
estimation of candidate parent sets; and (ii) feature selection. We
experimentally compare learnDAG to several popular competitors in recovering
the true structure of the graphs in situations where relatively moderate sample
sizes are available. Furthermore, to make our algorithm is stronger, a
validation of the algorithm is presented through the analysis of real datasets.
MoreTranslated text
AI Read Science
Must-Reading Tree
Example
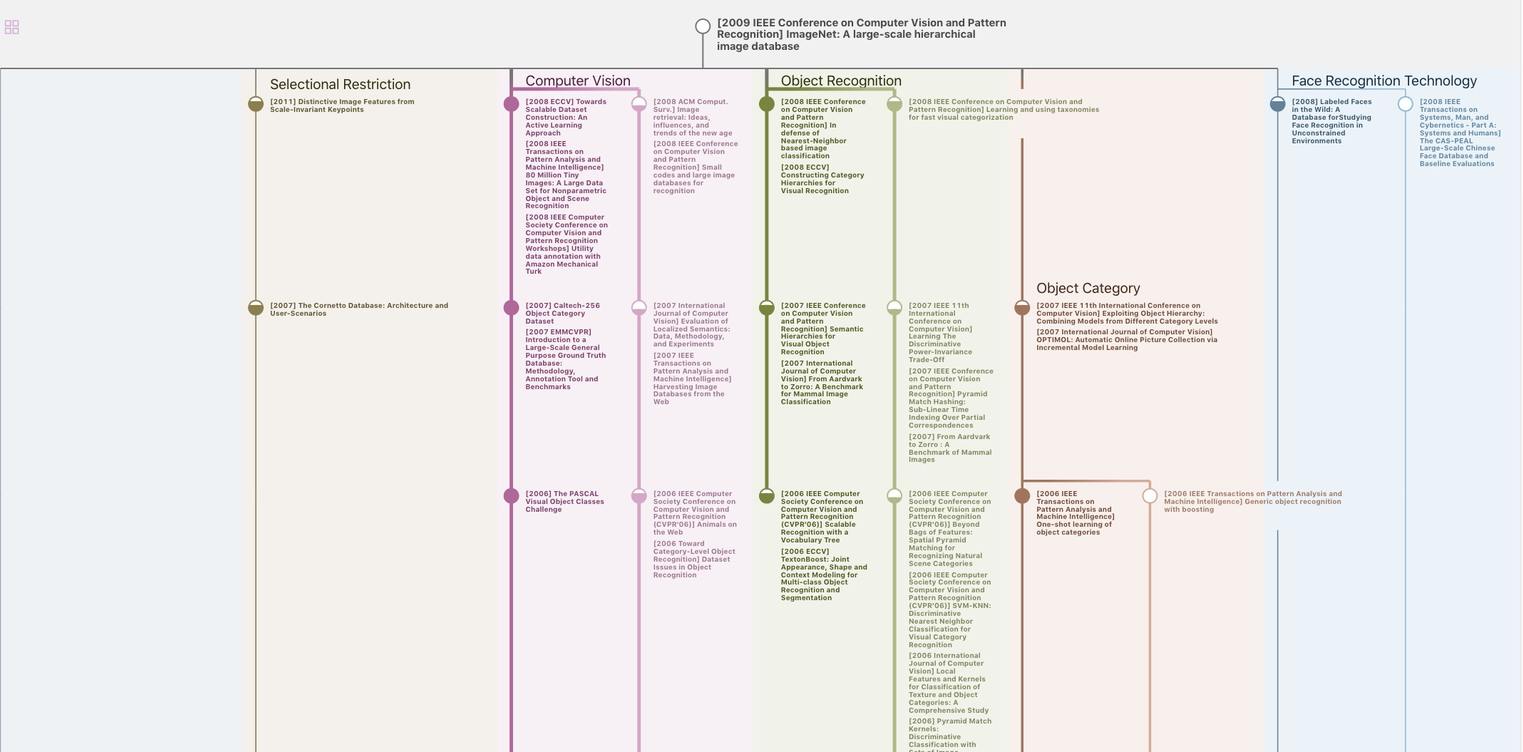
Generate MRT to find the research sequence of this paper
Chat Paper
Summary is being generated by the instructions you defined