The Gradual Resampling Ensemble for mining imbalanced data streams with concept drift.
Neurocomputing(2018)
摘要
Knowledge extraction from data streams has received increasing interest in recent years. However, most of the existing studies assume that the class distribution of data streams is relatively balanced. The reaction of concept drifts is more difficult if a data stream is class imbalanced. Current oversampling methods generally selectively absorb the previously received minority examples into the current minority set by evaluating similarities of past minority examples and the current minority set. However, the similarity evaluation is easily affected by data difficulty factors. Meanwhile, these oversampling techniques have ignored the majority class distribution, thus risking class overlapping.
更多查看译文
关键词
Concept drift,Data stream mining,Ensemble classifier,Class imbalance
AI 理解论文
溯源树
样例
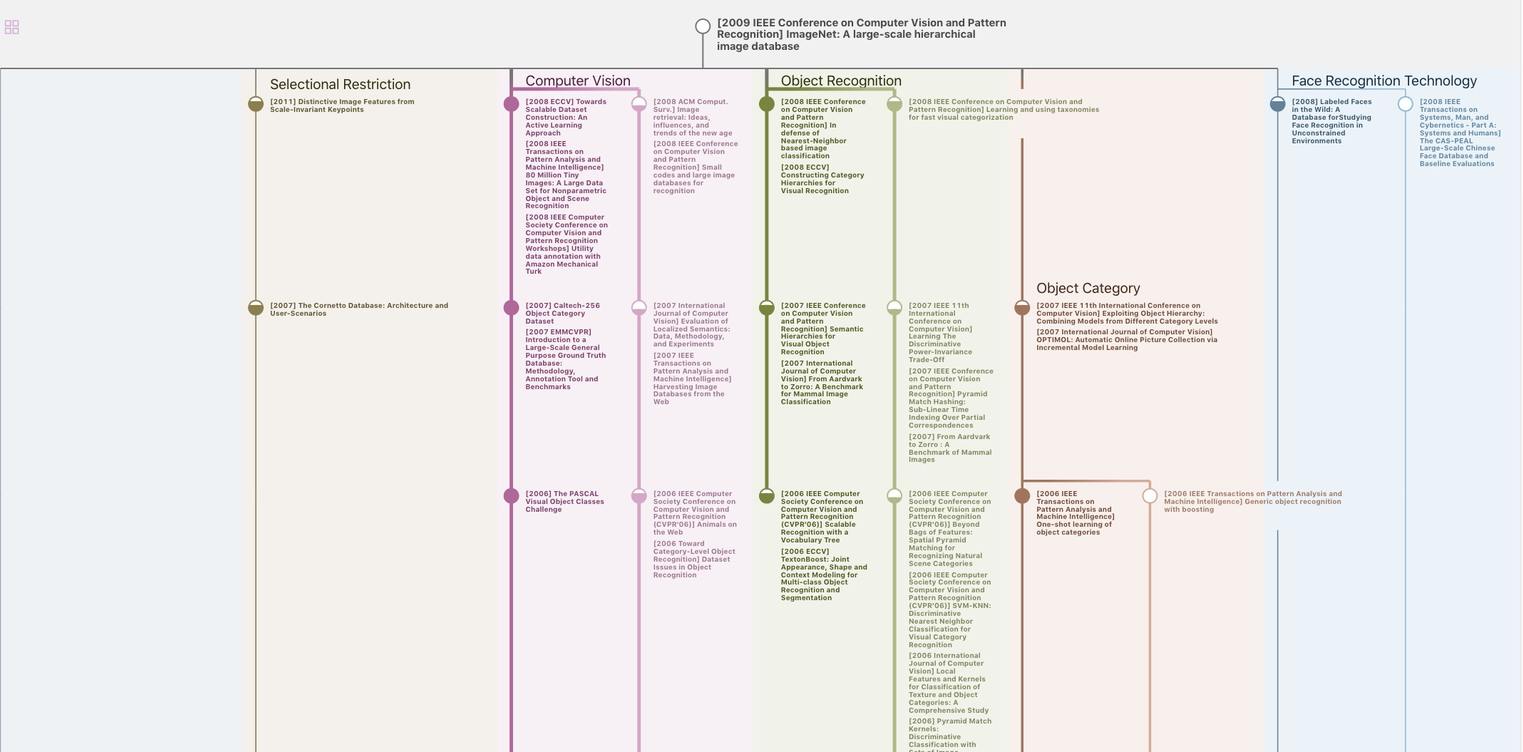
生成溯源树,研究论文发展脉络
Chat Paper
正在生成论文摘要