Graph neural network-based anomaly detection for river network systems [version 2; peer review: 1 approved, 2 approved with reservations]
F1000Research(2024)
Abstract
Background Water is the lifeblood of river networks, and its quality plays a crucial role in sustaining both aquatic ecosystems and human societies. Real-time monitoring of water quality is increasingly reliant on in-situ sensor technology. Anomaly detection is crucial for identifying erroneous patterns in sensor data, but can be a challenging task due to the complexity and variability of the data, even under typical conditions. This paper presents a solution to the challenging task of anomaly detection for river network sensor data, which is essential for accurate and continuous monitoring. Methods We use a graph neural network model, the recently proposed Graph Deviation Network (GDN), which employs graph attention-based forecasting to capture the complex spatio-temporal relationships between sensors. We propose an alternate anomaly threshold criteria for the model, GDN+, based on the learned graph. To evaluate the model’s efficacy, we introduce new benchmarking simulation experiments with highly-sophisticated dependency structures and subsequence anomalies of various types. We also introduce software called gnnad. Results We further examine the strengths and weaknesses of this baseline approach, GDN, in comparison to other benchmarking methods on complex real-world river network data. Conclusions Findings suggest that GDN+ outperforms the baseline approach in high-dimensional data, while also providing improved interpretability.
MoreTranslated text
Key words
Anomaly Detection,Graph Deviation Network,Graph Neural Network,Multivariate Time Series,Graph Attention Forecasting,Spatio-temporal Data,eng
AI Read Science
Must-Reading Tree
Example
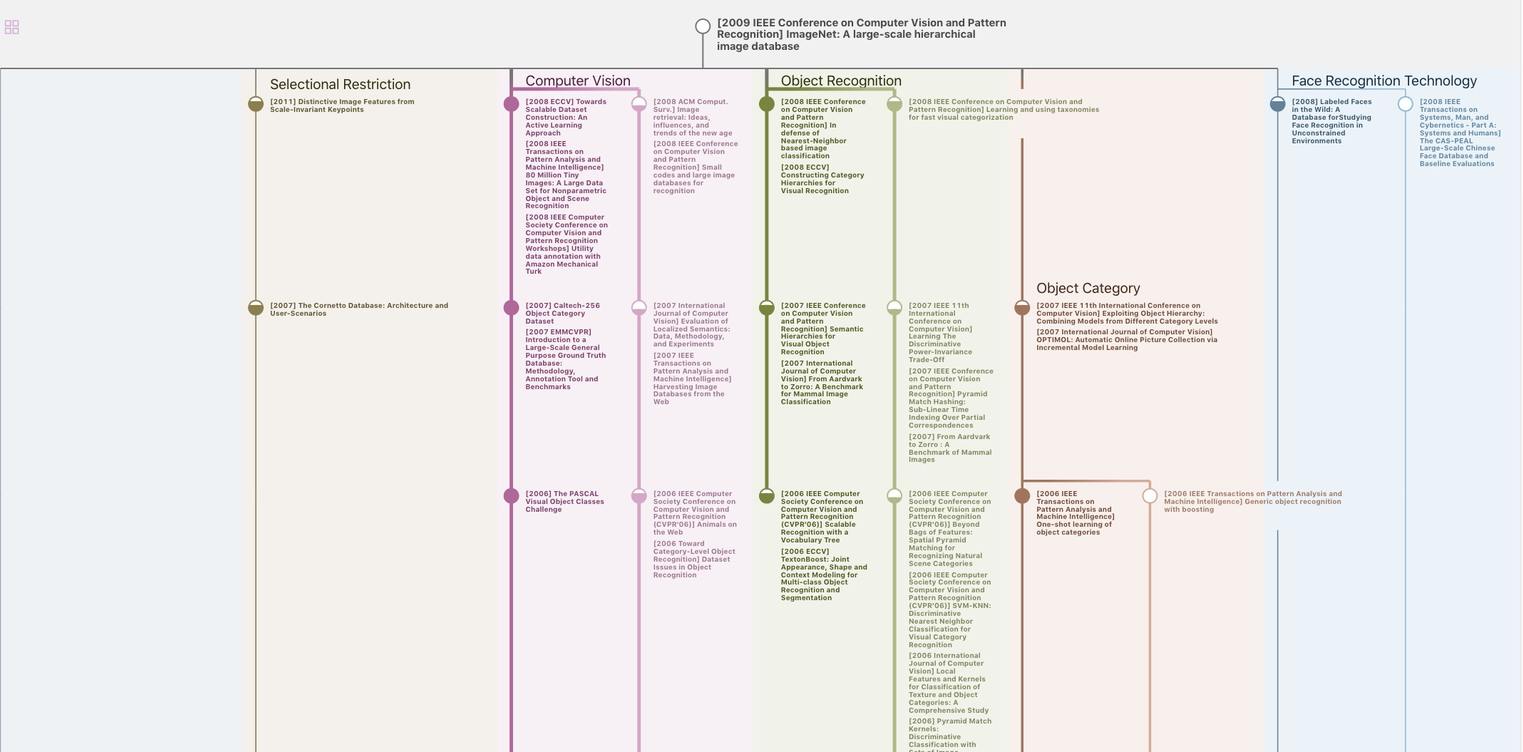
Generate MRT to find the research sequence of this paper
Chat Paper
Summary is being generated by the instructions you defined