GrabPhisher : Phishing Scams Detection in Ethereum via Temporally Evolving GNNs
IEEE Transactions on Services Computing(2024)
Abstract
Phishing scams are one of Ethereum's most representative security risks that can defraud many transactions in a short period and severely threaten network security. Existing deep learning-based phishing scam detection methods mainly rely on constructing static transaction graphs which are assumed to be accessible before model training. However, static methods that have a high false positive rate to detect newly generated phishing scams by adding this newly generated data to existing algorithms for execution, due to new accounts and transactions constantly appearing in the real-world Ethereum network. Therefore, this paper, for the first time, proposes a novel evolve-based phishing scams detection method (named GrabPhisher) that extracts temporal features of accounts and captures information about the dynamic topology of the graph as it evolves. Specifically, GrabPhisher can build the evolutionary pattern of accounts trading on Ethereum as a diffusion network graph in continuous time. It can continue to capture new transaction features based on existing transactions, which facilitates the identification of phishing accounts. Additionally, we implement GrabPhisher on the real-world Ethereum phishing scams datasets. Extensive experimental results demonstrate that GrabPhisher can effectively extract dynamic temporal features and outperform state-of-the-art methods (95% Recall, and 88% F1-score).
MoreTranslated text
Key words
Ethereum,Phishing scams detection,Temporal evolution,Diffusion networks
AI Read Science
Must-Reading Tree
Example
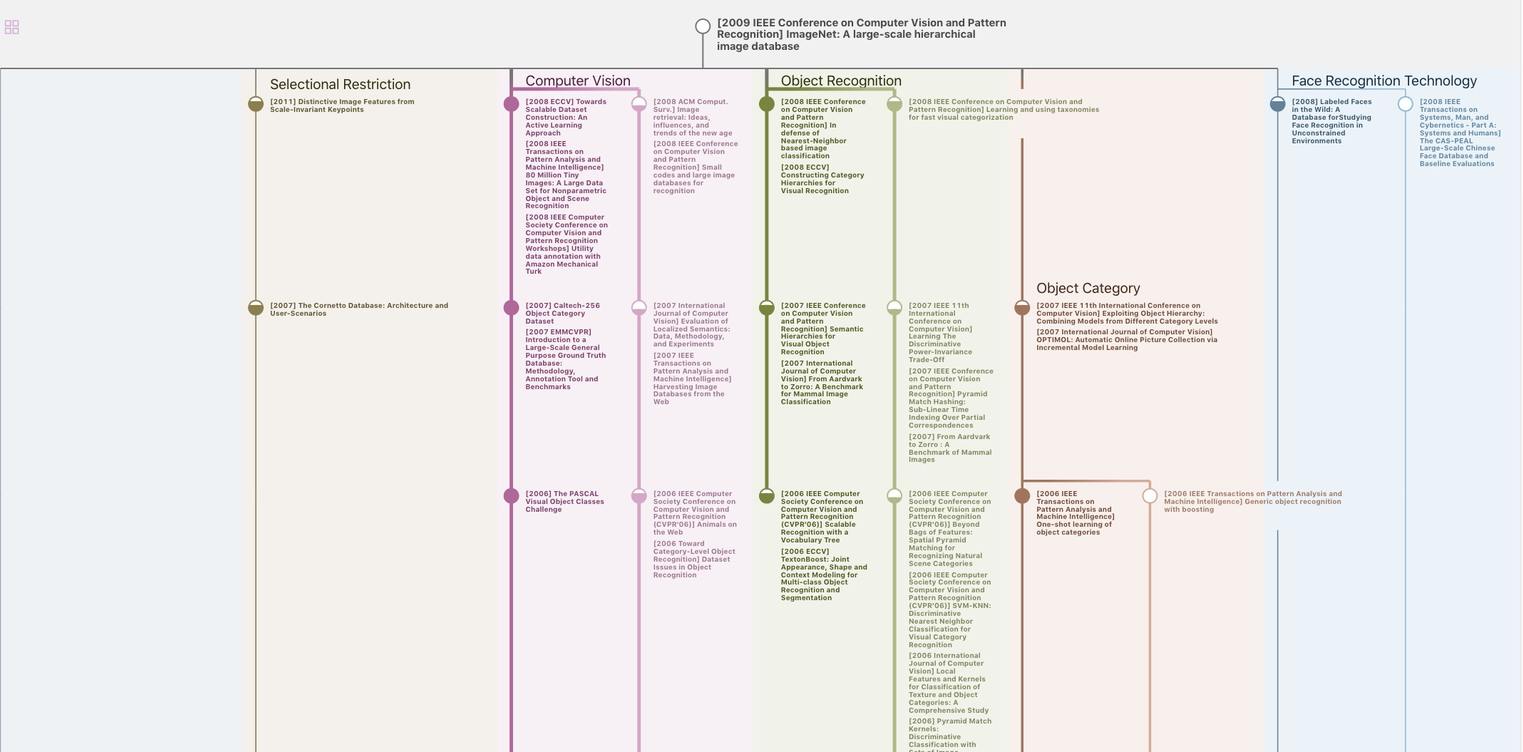
Generate MRT to find the research sequence of this paper
Chat Paper
Summary is being generated by the instructions you defined