Extraction of Building Construction Time Using the LandTrendr Model With Monthly Landsat Time Series Data
IEEE Journal of Selected Topics in Applied Earth Observations and Remote Sensing(2024)
摘要
Extracting building construction time is crucial for effective land resource management and sustainable urban development, particularly in fast-growing urban areas. However, acquiring building construction time remains challenging due to limited observations with multiple changes. To address this issue, we applied a monthly time series of remote sensing images and the LandTrendr change detection algorithm to extract building construction times. We identified the sensitive index of short wavelength infra-red (SWIR) from satellite observations for detecting changes in building construction, demolition, and reconstruction. Through comparing with composited results derived from satellite time series data with different temporal intervals, we found that the monthly time series can more accurately characterize the building changes than other temporal intervals (e.g., daily and yearly). Then, we improved the commonly used LandTrendr algorithm in the Google Earth Engine platform with monthly time series data and identified the maximum change time as the temporal turning point for construction at the pixel scale. Finally, we revealed Beijing's construction time from 1990 to 2020 by spatially overlaying the building footprint data and the extracted year information from Landsat time series images. Our results showed an overall agreement of 82.32% regarding the identified construction time of buildings across a wide range, using a two-year tolerance strategy and randomly collecting 560 building samples. Our results outperformed traditional methods such as annual time series composition with the same LandTrendr algorithm, historical surveying map, and building change time of social big data monitoring, with derived overall accuracies of 68.75%, 74.64%, and 67.47%, respectively, suggesting the good performance of the adopted approach. This study offered a potential avenue for detailed monitoring of urban building changes at a fine-grained spatial scale, with far-reaching implications for sustainable urban development practices.
更多查看译文
关键词
Building footprints,change detection,construction,monthly composition
AI 理解论文
溯源树
样例
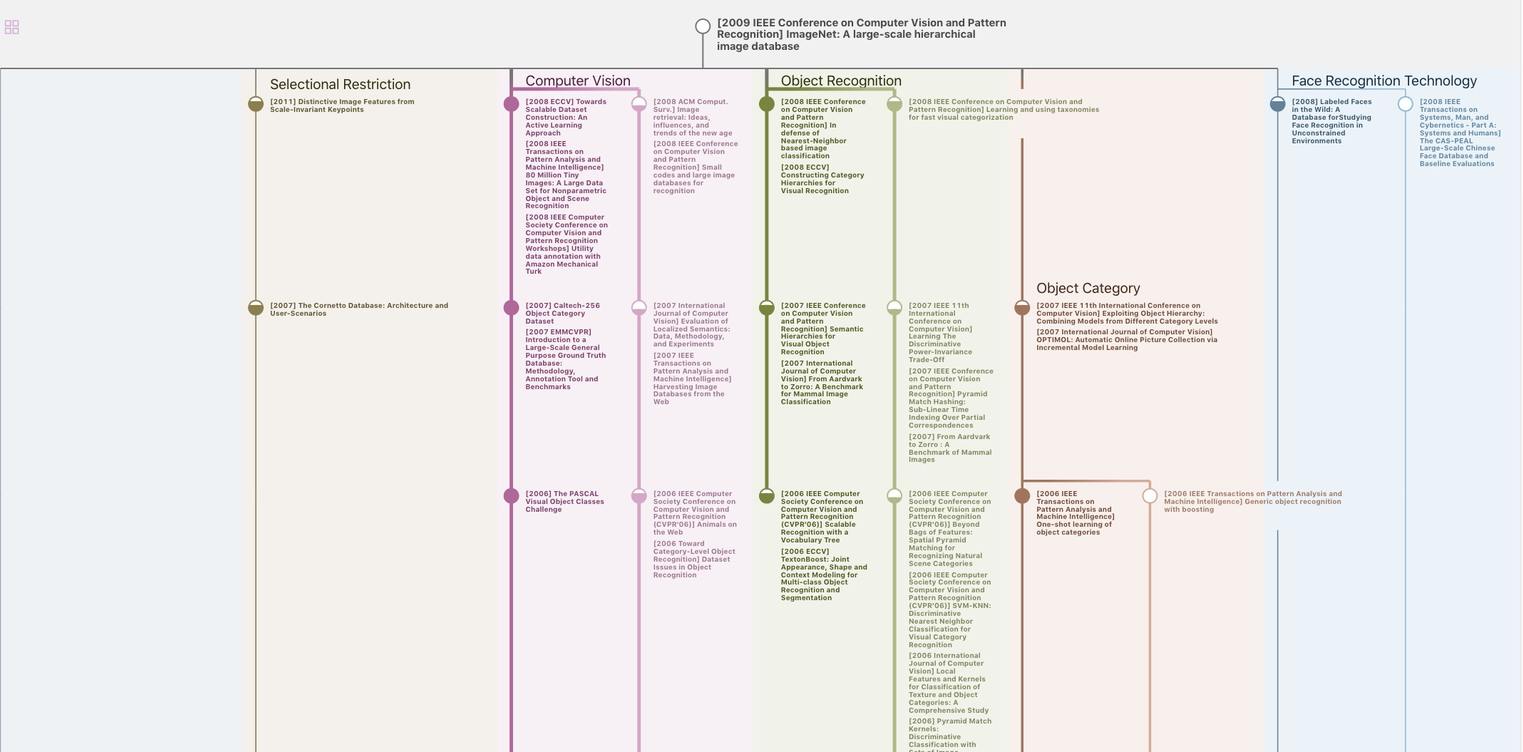
生成溯源树,研究论文发展脉络
Chat Paper
正在生成论文摘要