Advance One-Shot Multispectral Instance Detection With Text's Supervision.
IEEE Signal Process. Lett.(2024)
摘要
One key issue within one-shot multispectral instance detection (OMID) is to extract features of strong instance discriminative power, domain adaptation capability, and instancewise generality. Existing methods generally only rely on visual clues. Comparatively, text is advantageous due to its structured information, high semantics, and low noise. Inspired by recent emergence of large image-text datasets and breakthrough visuallanguage models, we propose to advance OMID with text's supervision for the first time. To this end, our key idea is to establish the relationship between one-shot multispectral instance with ImageNet class labels via the CLIP model. Particularly, we retrieve, rank, and ensemble the text features of ImageNet labels via instance image feature as query. Then the resulting instance image and text features are realigned and fused to obtain a multimodal feature. Meanwhile, a multispectral contrastive learning approach is proposed to drive multimodal feature learning for OMID. Note that all the procedures are end-toend trained in a unified network. In this way, the instance discriminative power and domain adaptation capability are facilitated simultaneously. Experiments on two tailored multispectral instance detection datasets verify the effectiveness of our method. The source code will be released upon acceptance at https://github.com/ChenFengJR/OMID-multimodal.
更多查看译文
关键词
multispectral instance detection,text supervision,visual-language model,multimodal feature learning
AI 理解论文
溯源树
样例
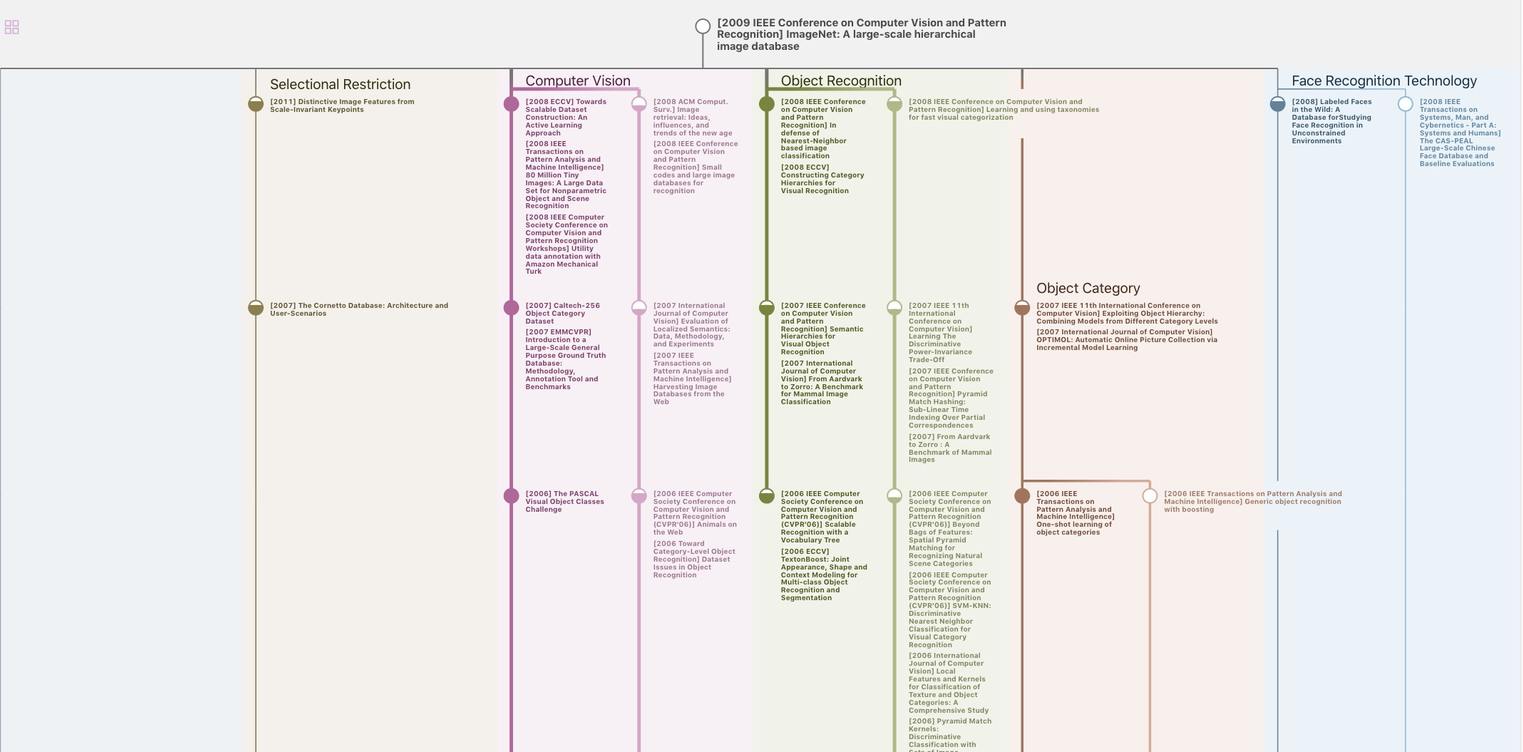
生成溯源树,研究论文发展脉络
Chat Paper
正在生成论文摘要