Deep Reinforcement Learning Based Radar Parameter Adaptation for Multiple Target Tracking
IEEE Transactions on Aerospace and Electronic Systems(2024)
摘要
The field of cognitive radar seeks to improve performance in different scenarios using adaptive means based on environmental information. This work focuses on the problem of radar parameter adaptation to ensure that targets are not lost and improve tracking accuracy in multiple target tracking (MTT) scenarios with variable target numbers and multiple target types. The dimensionality increase due to multiple targets and the complexity due to multi-dimensional parameter space are the difficulties of the MTT scenario optimization problem, whereas the variation of the number of targets in a specific scenario is also a problem to be investigated. A deep reinforcement learning (DRL) based radar parameter adaptation approach is proposed to solve the problem. The complexity is reduced by dividing the parameter space and using two agents in cooperation to perform parameter selection. We design the reward functions for each agent separately and adopt additional information for training to enhance the training efficiency. An improved DRL agent using long short-term memory (LSTM) network and self-attention mechanism is proposed to deal with the problem of target number variation and multiple target types. Simulation results demonstrate the performance of the proposed approach, achieving almost no target tracking loss in simulation scenarios, optimal performance compared with the criterion-based methods and the fixed parameter method, and obtaining about 90% improvement in range and velocity accuracy compared with the fixed parameter method, whereas the processing time is only 0.48% of that of the grid search criterion-based methods.
更多查看译文
AI 理解论文
溯源树
样例
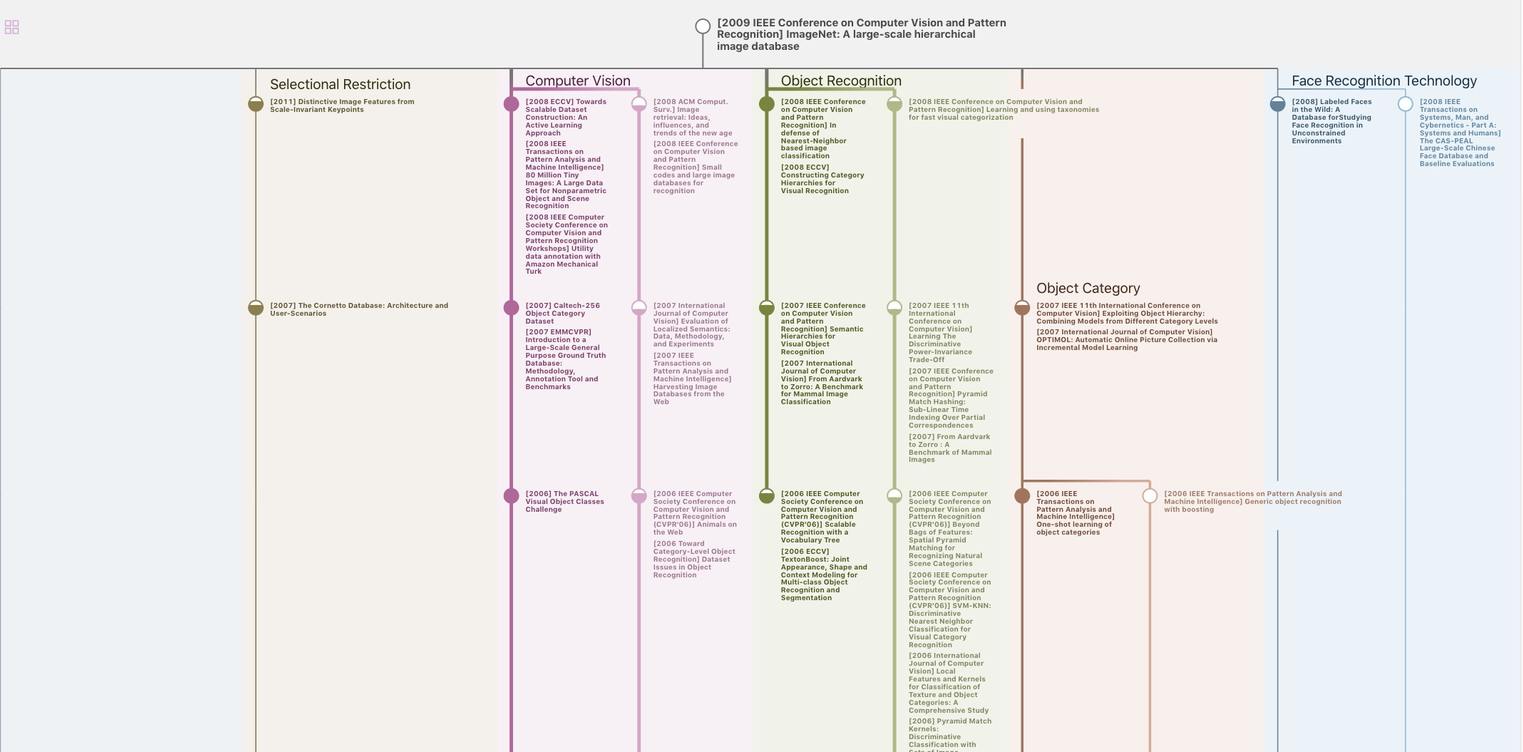
生成溯源树,研究论文发展脉络
Chat Paper
正在生成论文摘要