Learning with image guidance for digital elevation model super-resolution
2024 International Conference on Machine Intelligence for GeoAnalytics and Remote Sensing (MIGARS)(2024)
摘要
Acquiring higher-resolution Digital Elevation Model (DEM) data is important for many land-surface applications. Creating high-resolution DEMs from freely available low-resolution satellite-derived DEMs, along with supplementary data about the land surface, is a possible solution that may provide the data needed, particularly in areas without high-resolution DEMs. This work investigates sparse-to-dense depth completion approaches for DEM super-resolution. It proposes a neural network based on joint image filtering and spatial propagation networks, reconstructing high-resolution DEMs deriving from low-resolution DEMs and guidance images. Experiments demonstrate that our results are improved by 56%/38% in accuracy and 9%/4% in reconstruction quality compared to Bicubic/EDSR methods.
更多查看译文
关键词
Digital elevation model,super-resolution,depth estimation,convolutional neural networks
AI 理解论文
溯源树
样例
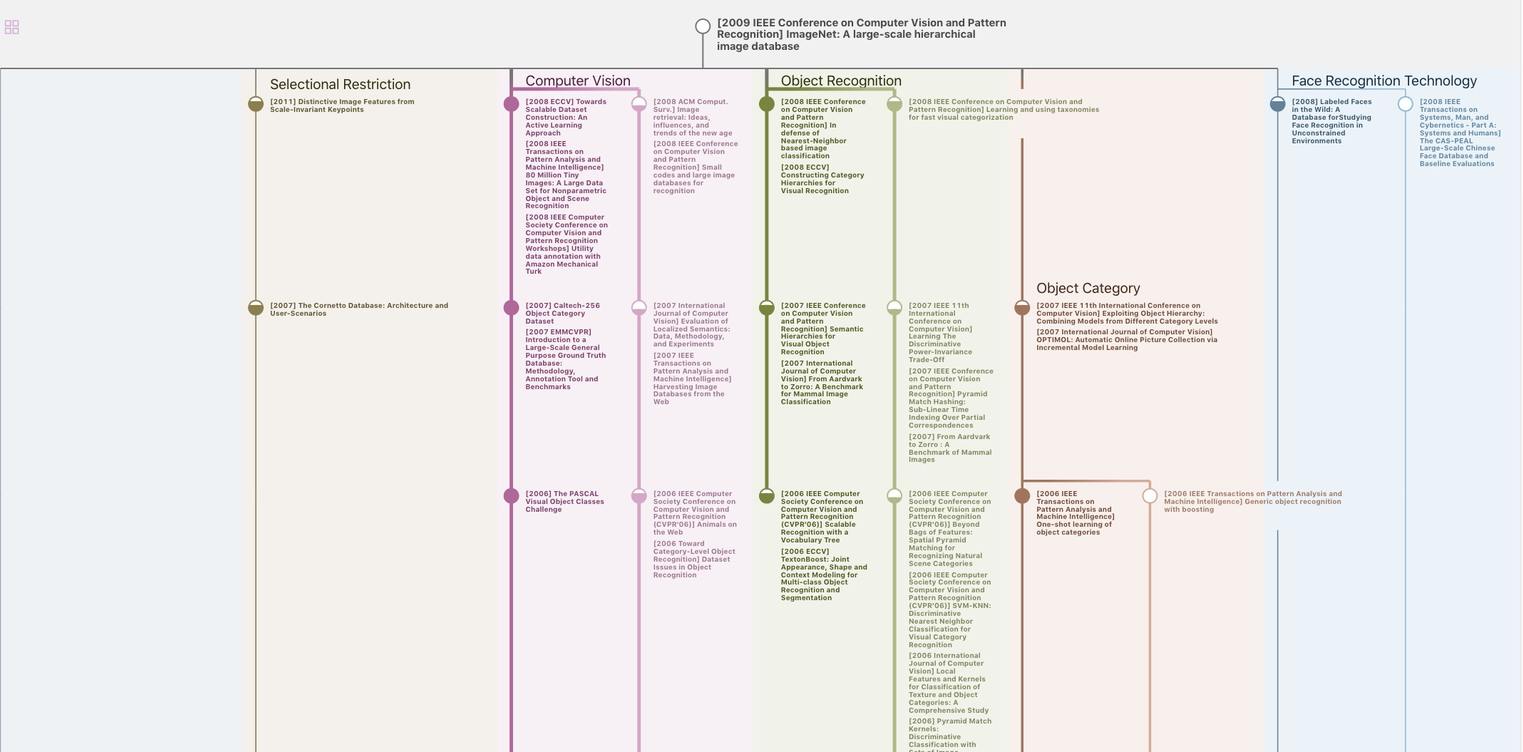
生成溯源树,研究论文发展脉络
Chat Paper
正在生成论文摘要