Approximately orthogonal non-negative Tucker decomposition with graph regularized for multiway dimensionality reduction
crossref(2024)
摘要
Abstract Non-negative Tucker decomposition (NTD) is one of the renowned technique in feature extraction and representation for non-negative high-dimensional tensor data. The main focus behind NTD is how to factorize the data to get hold of a high quality data representation from multidimensional directions. However, NTD does not conserve the geometrical structure of the data space and does not consider relationship and property among columns of the factor matrices. In this paper, by using approximately orthogonal constraint and graph regularized constraint , we manage to capture nonlinear local features of data space and further enhance expressiveness of the NTD clustering method. First, based on the uni-side and bi-side approximate orthogonality, we propose two novel approximately orthogonal non-negative Tucker decomposition with graph regularized models, which not only in part make factor matrix tend to be orthogonality, but also preserve the geometrical information from high-dimensional tensor data. Then we develop the iterative updating algorithm depended on multiplicative update rule to solve the proposed models, and provide its convergence and computational complexity. Finally, we use numerical experimental results to demonstrate the effectiveness, robustness and efficiency of the proposed new methods on the real-world image datasets.
更多查看译文
AI 理解论文
溯源树
样例
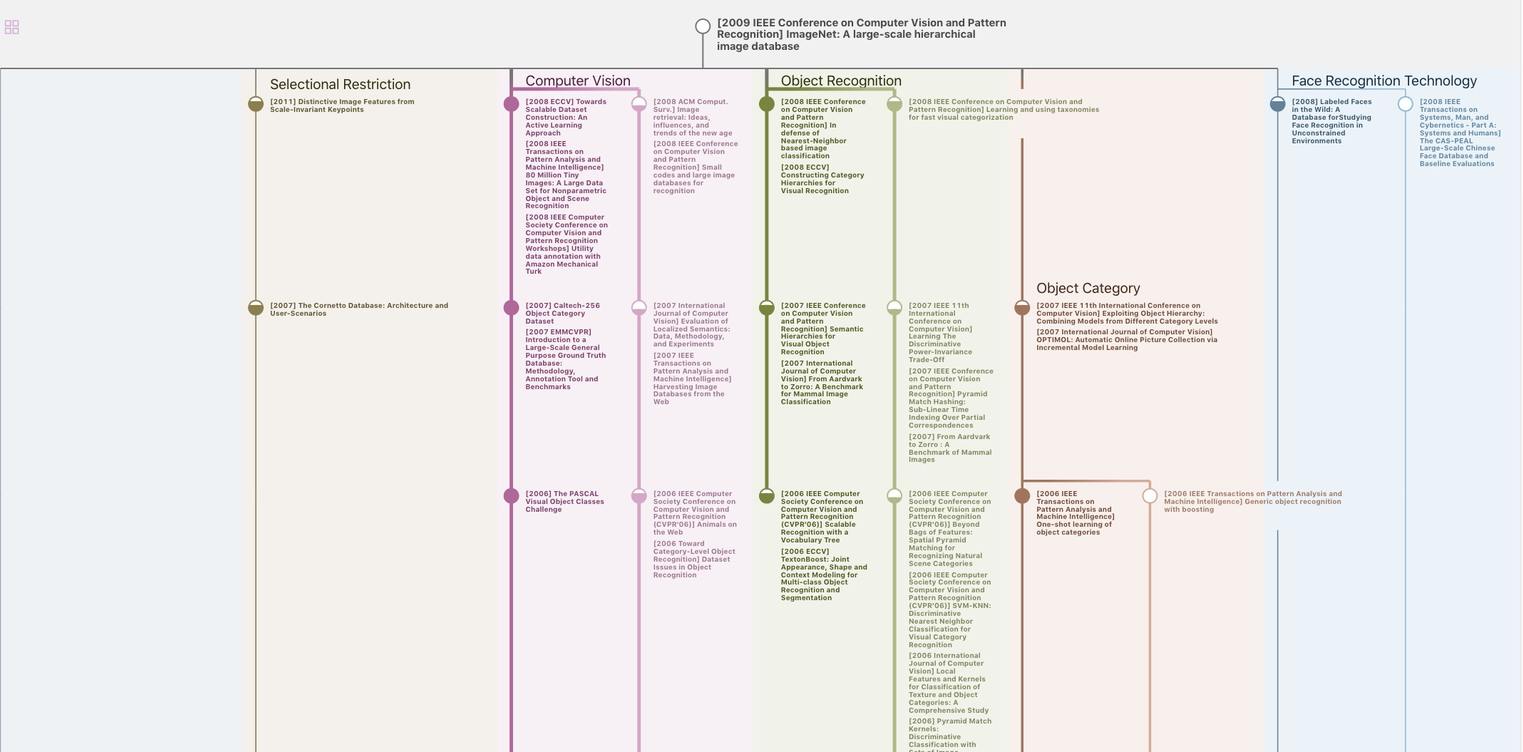
生成溯源树,研究论文发展脉络
Chat Paper
正在生成论文摘要