Edge-Cloud Remote Sensing Data Based Plant Disease Detection Using Deep Neural Networks With Transfer Learning
IEEE Journal of Selected Topics in Applied Earth Observations and Remote Sensing(2024)
摘要
These days, the disease among different plants has been increasing day by day. It is a very hard task for government institutions and farmers to collect data on plant diseases from different distributed lands among regions. Therefore, data collection, disease detection, and processing are the key issues for plants when they are suffering from healthy and unhealthy issues in different lands. This paper presents edge-cloud remote sensing data-based plant disease detection by exploiting deep neural networks with transfer learning. The objective is to solve the aforementioned issues, such as data collection at a wide range, disease detection, and processing them with higher accuracy and time on different machines. We suggest transfer learning commutative fuzzy deep convolutional neural network (FCDCNN) schemes based on combinatorial optimization problems. The convex function optimizes the processing time and learning rate of data training on different edge and cloud nodes to collect more and more data from different plants from distributed lands. In the concave function, we predict the diseases among different plants such as sugar cane, blueberry, cotton, and cherry with images, videos, and numeric values. The plant disease detection app uses edge nodes and remote satellite point cloud nodes to gather and train data using transfer learning and make predictions using fuzzy DCNN schemes that are more accurate and take less time to process. Simulation results show that FCDCNN obtained higher accuracy by 98% with less processing time 25% and trained with a higher ratio of data than existing schemes.
更多查看译文
关键词
Edge point cloud,fuzzy deep neural networks,plant disease detection,remote sensing data,transfer learning
AI 理解论文
溯源树
样例
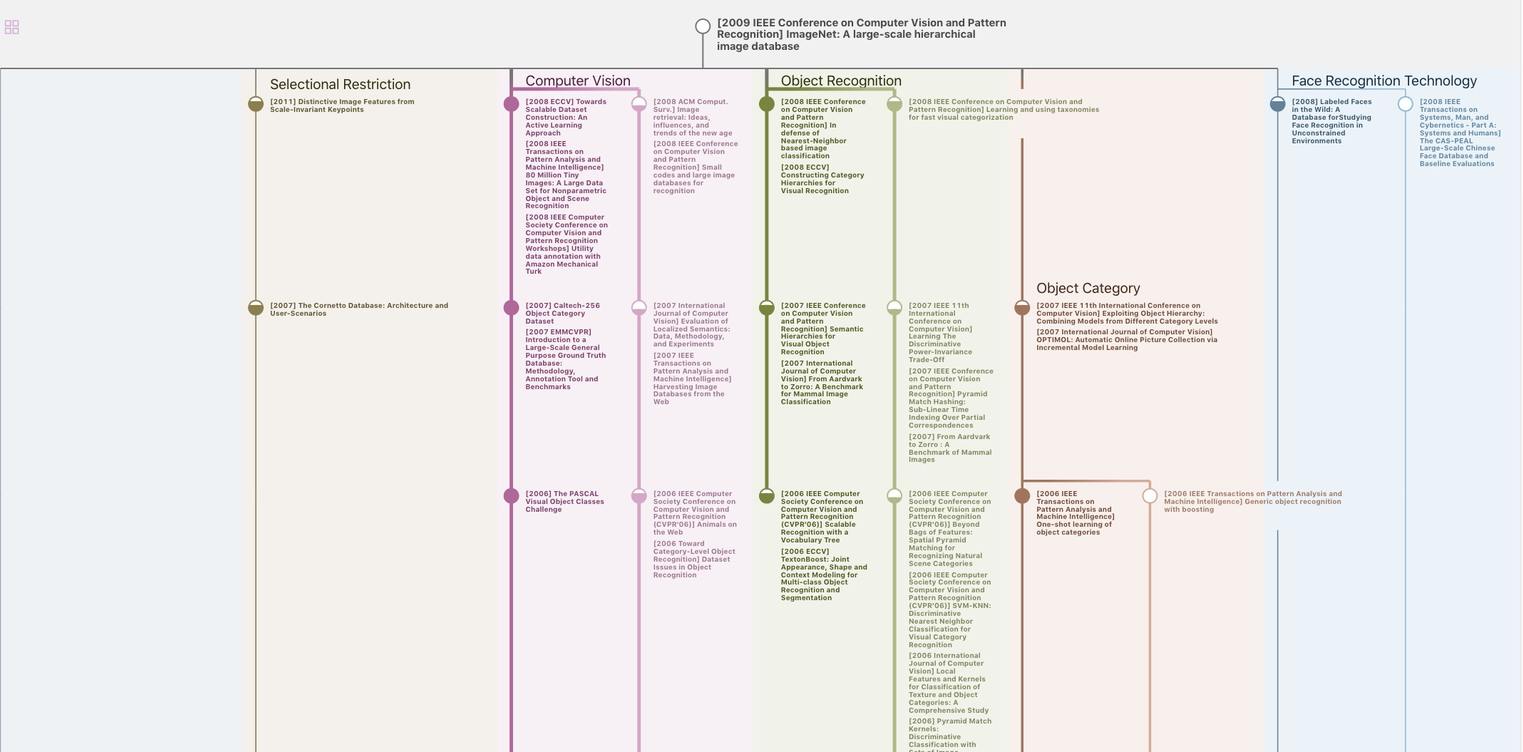
生成溯源树,研究论文发展脉络
Chat Paper
正在生成论文摘要