Monocular Localization with Semantics Map for Autonomous Vehicles
ICRA 2024(2024)
摘要
Accurate and robust localization remains a significant challenge for autonomous vehicles. The cost of sensors and limitations in local computational efficiency make it difficult to scale to large commercial applications. Traditional vision-based approaches focus on texture features that are susceptible to changes in lighting, season, perspective, and appearance. Additionally, the large storage size of maps with descriptors and complex optimization processes hinder system performance. To balance efficiency and accuracy, we propose a novel lightweight visual semantic localization algorithm that employs stable semantic features instead of low-level texture features. First, semantic maps are constructed offline by detecting semantic objects, such as ground markers, lane lines, and poles, using cameras or LiDAR sensors. Then, online visual localization is performed through data association of semantic features and map objects. We evaluated our proposed localization framework in the publicly available KAIST Urban dataset and in scenarios recorded by ourselves. The experimental results demonstrate that our method is a reliable and practical localization solution in various autonomous driving localization tasks.
更多查看译文
关键词
Autonomous Vehicle Navigation,Localization,Intelligent Transportation Systems
AI 理解论文
溯源树
样例
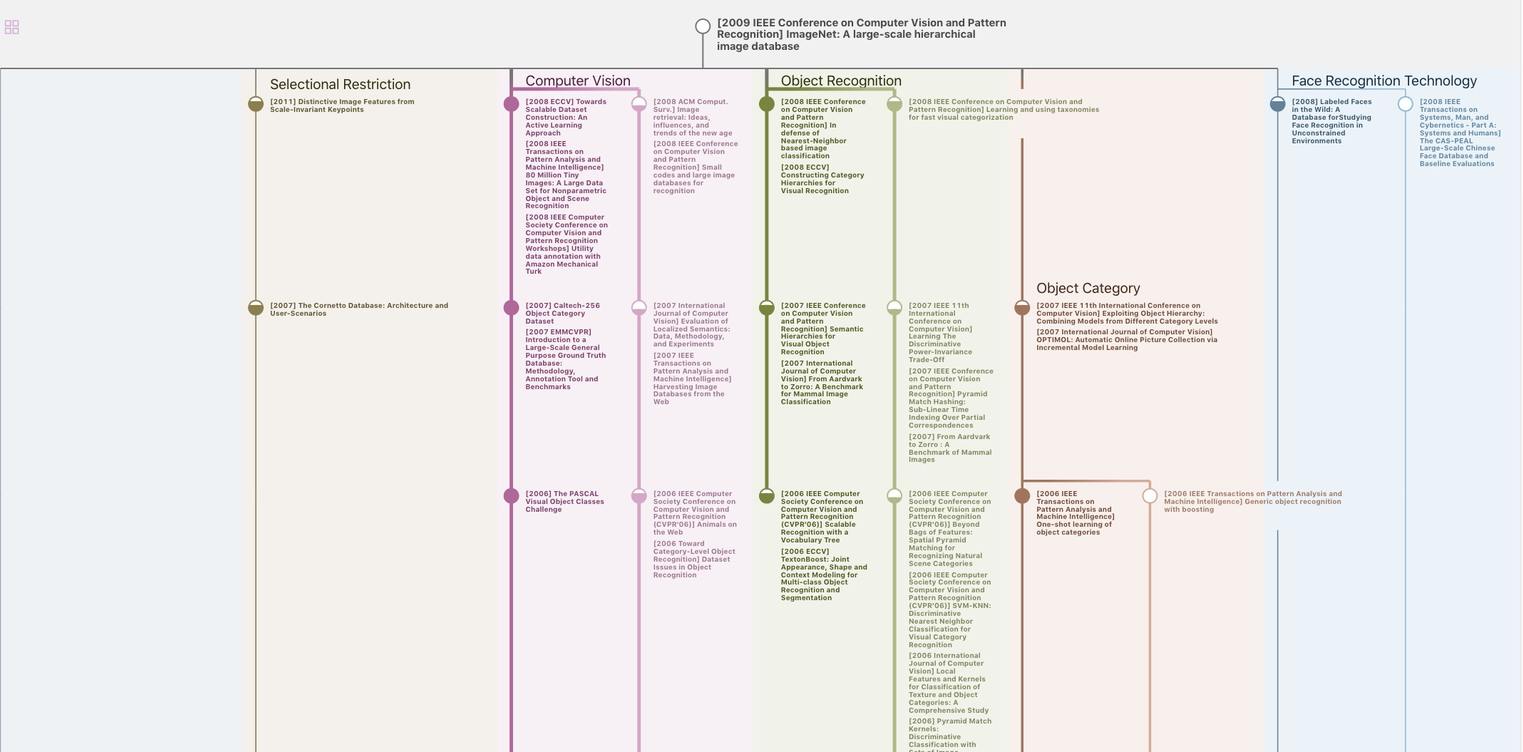
生成溯源树,研究论文发展脉络
Chat Paper
正在生成论文摘要