Active ML for 6G: Towards Efficient Data Generation, Acquisition, and Annotation
CoRR(2024)
摘要
This paper explores the integration of active machine learning (ML) for 6G
networks, an area that remains under-explored yet holds potential. Unlike
passive ML systems, active ML can be made to interact with the network
environment. It actively selects informative and representative data points for
training, thereby reducing the volume of data needed while accelerating the
learning process. While active learning research mainly focuses on data
annotation, we call for a network-centric active learning framework that
considers both annotation (i.e., what is the label) and data acquisition (i.e.,
which and how many samples to collect). Moreover, we explore the synergy
between generative artificial intelligence (AI) and active learning to overcome
existing limitations in both active learning and generative AI. This paper also
features a case study on a mmWave throughput prediction problem to demonstrate
the practical benefits and improved performance of active learning for 6G
networks. Furthermore, we discuss how the implications of active learning
extend to numerous 6G network use cases. We highlight the potential of active
learning based 6G networks to enhance computational efficiency, data annotation
and acquisition efficiency, adaptability, and overall network intelligence. We
conclude with a discussion on challenges and future research directions for
active learning in 6G networks, including development of novel query
strategies, distributed learning integration, and inclusion of human- and
machine-in-the-loop learning.
更多查看译文
AI 理解论文
溯源树
样例
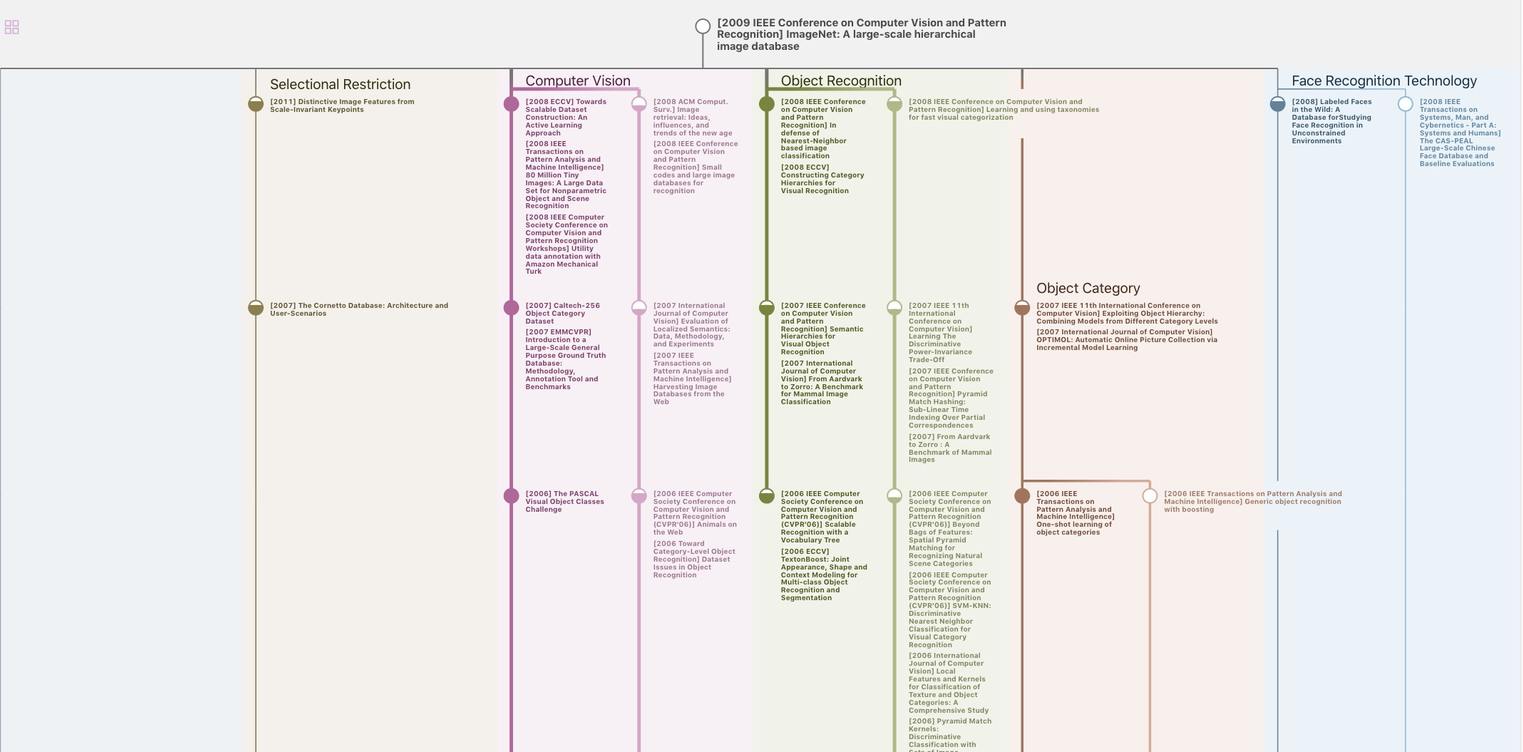
生成溯源树,研究论文发展脉络
Chat Paper
正在生成论文摘要