Synthetic Oversampling: Theory and A Practical Approach Using LLMs to Address Data Imbalance.
CoRR(2024)
AI Read Science
Must-Reading Tree
Example
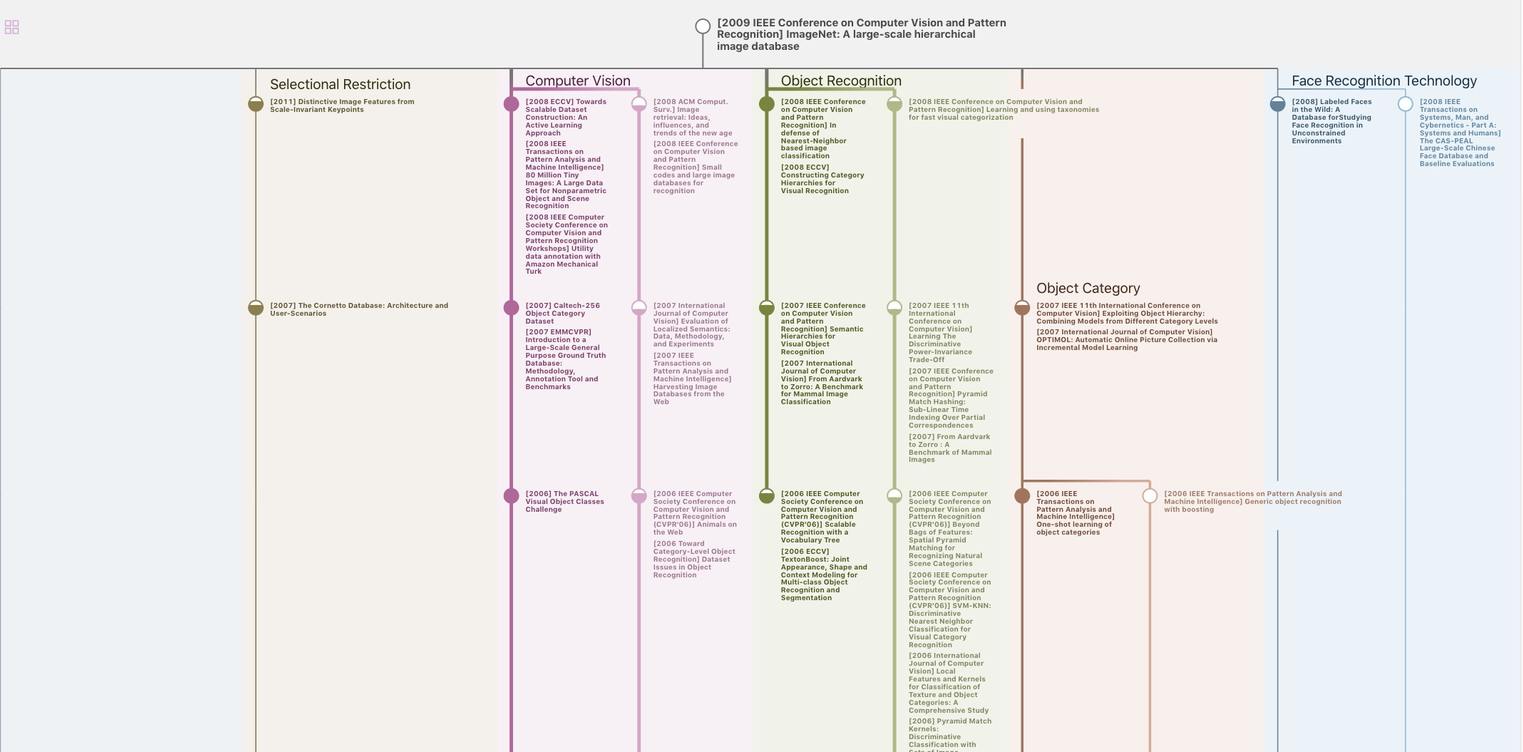
Generate MRT to find the research sequence of this paper
Chat Paper
Summary is being generated by the instructions you defined