Private Online Learning via Lazy Algorithms
CoRR(2024)
Abstract
We study the problem of private online learning, specifically, online
prediction from experts (OPE) and online convex optimization (OCO). We propose
a new transformation that transforms lazy online learning algorithms into
private algorithms. We apply our transformation for differentially private OPE
and OCO using existing lazy algorithms for these problems. Our final algorithms
obtain regret, which significantly improves the regret in the high privacy
regime ε≪ 1, obtaining √(T log d) + T^1/3log(d)/ε^2/3 for DP-OPE and √(T) + T^1/3√(d)/ε^2/3 for DP-OCO. We also complement our results with a
lower bound for DP-OPE, showing that these rates are optimal for a natural
family of low-switching private algorithms.
MoreTranslated text
AI Read Science
Must-Reading Tree
Example
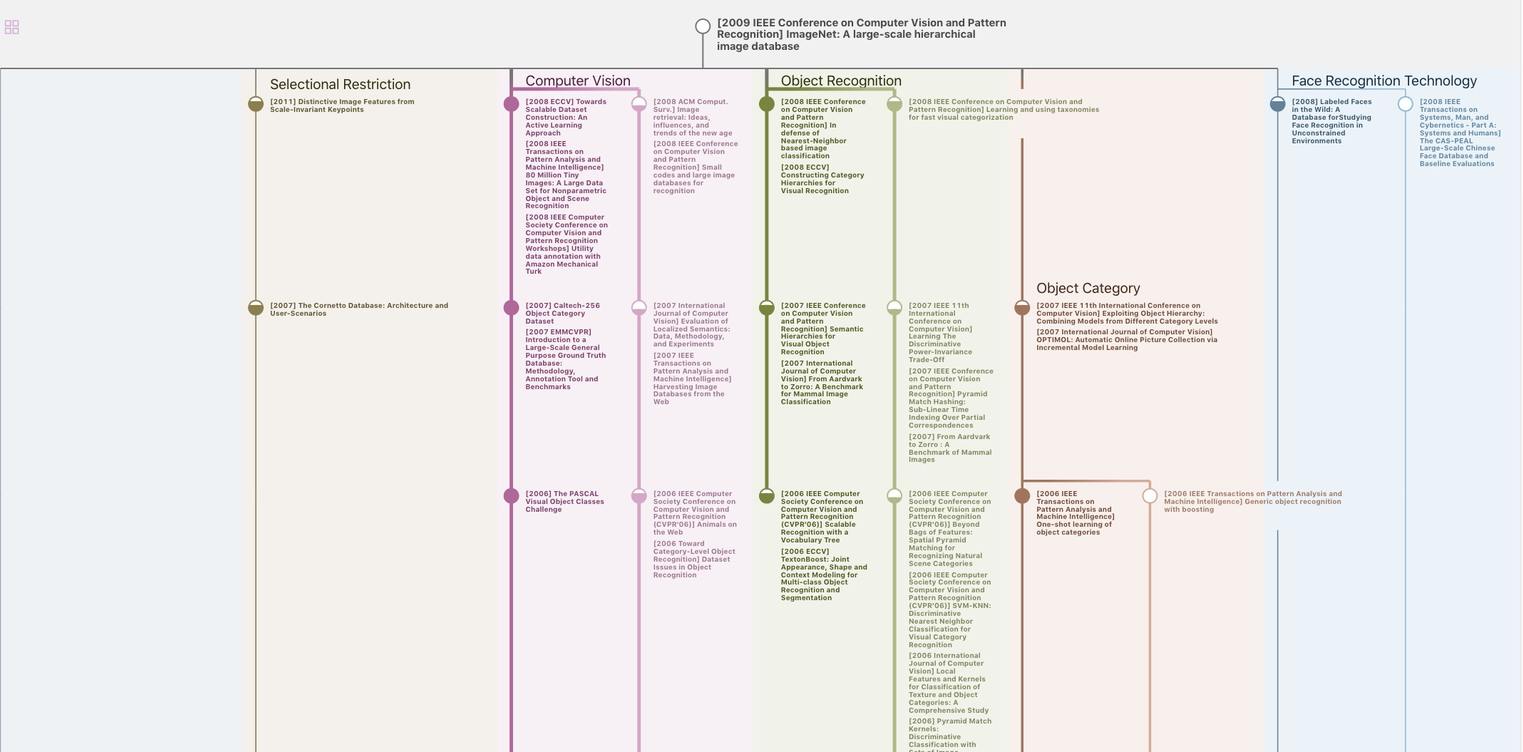
Generate MRT to find the research sequence of this paper
Chat Paper
Summary is being generated by the instructions you defined